机器学习实战︱基于朴素贝叶斯算法的垃圾信息的识别
Posted 清华计算机学堂
tags:
篇首语:本文由小常识网(cha138.com)小编为大家整理,主要介绍了机器学习实战︱基于朴素贝叶斯算法的垃圾信息的识别相关的知识,希望对你有一定的参考价值。
本文将带领大家亲手实现一个垃圾信息过滤的算法。
在正式讲解算法之前,最重要的是对整个任务有一个全面的认识,包括算法的输入和输出、可能会用到的技术,以及技术大致的流程。
本任务的目标是去识别一条短信是否为垃圾信息,即输入为一条文本信息,输出为二分类的分类结果。2002年,Paul Graham提出使用“贝叶斯推断”过滤垃圾邮件。1000封垃圾邮件可以过滤掉995封,且没有一个误判。另外,这种过滤器还具有自我学习的功能,会根据新收到的邮件,不断调整。收到的垃圾邮件越多,它的准确率就越高。
朴素贝叶斯算法是一种有监督的机器学习算法,即算法的实现包含了构建训练集、数据预处理、训练、在测试集上验证等步骤。在下文中首先介绍算法的理论基础,再逐一介绍代码实现算法的整个流程。
01
算法流程
1
●
解析训练集中所有信息,并提取每一个词。
2
●
统计每一个词出现在正常信息和垃圾信息的词频
根据这个初步统计结果可以实现一个垃圾信息的鉴别器。对于一个新的样本输入,可以提取每一个词并根据前面给出的贝叶斯公式进行计算,最终得到分类结果。下面对一个简单的样例进行手工模拟,来熟悉算法的内部原理。
假设通过初步的统计,得到了以下两个单词在垃圾信息和正常信息中出现的频率,如表 13-1所示。
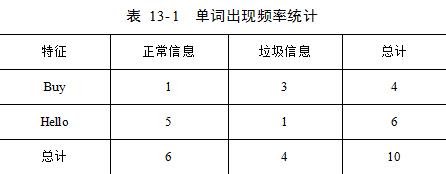
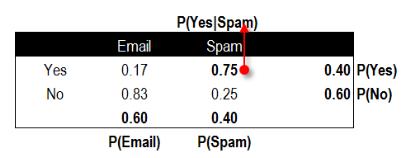
图 13-1 概率分布位置示意图
目前有一个新的信息为“Hello, Buy!”,首先分词得到Hello和Buy两个单词。根据贝叶斯公式,可以计算该信息属于垃圾信息的概率

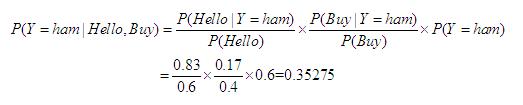
根据计算结果可知该信息属于正常信息。
02
数据集载入
代码清单 13-1定义了一个从文件中读取数据的函数,将信息内容与标签进行初步的处理,使其根据标签的不同,存储在不同的列表当中。
def getDateSet(dataPath=""r"./SMSSpamCollection"):
with open(dataPath, encoding='utf-8') as f:
txt_data = f.readlines()
data = [] # 所有信息
classTag = [] # 标签
for line in txt_data:
line_split = line.strip("\n").split('\t')
if line_split[0] == "ham":
data.append(line_split[1])
classTag.append(1)
elif line_split[0] == "spam":
data.append(line_split[1])
classTag.append(0)
return data, classTag
03
朴素贝叶斯模型
1
●
构造函数设计
代码清单 13-2 朴素贝叶斯构造函数
class NaiveBayes:
def __init__(self):
self.__ham_count = 0 # 正常短信数量
self.__spam_count = 0 # 垃圾短信数量
self.__ham_words_count = 0 # 正常短信单词总数
self.__spam_words_count = 0 # 垃圾短信单词总数
self.__ham_words = list() # 正常短信单词列表
self.__spam_words = list() # 垃圾短信单词列表
# 训练集中不重复单词集合
self.__word_dictionary_set = set()
self.__word_dictionary_size = 0
self.__ham_map = dict() # 正常短信的词频统计
self.__spam_map = dict() # 垃圾短信的词频统计
self.__ham_probability = 0.0
self.__spam_probability = 0.0
2
●
数据预处理
# 输入为一封信息的内容
def data_preprocess(self, sentence):
# 将输入转换为小写并将特殊字符替换为空格
temp_info = re.sub('\W', ' ', sentence.lower())
# 根据空格将其分割为一个一个单词
words = re.split(r'\s+', temp_info)
# 返回长度大于等于3的所有单词
return list(filter(lambda x: len(x) >= 3, words))
3
●
模型训练

图 13-2 输入样例

图 13-3 命令行输出单词列表
最后调用word_count函数来对正常短信和垃圾短信的词频进行统计,并计算垃圾短信和正常短信的概率。word_count函数最终获得的字典输出如图 13-4所示,字典的键为单词本身,值为其出现的次数。使用字典的形式进行存储,方便在后面预测时可以较快的进行索引。

图 13-4 词频统计
代码清单 13-4 训练函数
def fit(self, X_train, y_train):
words_line = []
for sentence in X_train:
words_line.append(self.data_preprocess(sentence))
self.build_word_set(words_line, y_train)
self.word_count()
def build_word_set(self, X_train, y_train):
for words, y in zip(X_train, y_train):
if y == 0:
# 正常短信
self.__ham_count += 1
self.__ham_words_count += len(words)
for word in words:
self.__ham_words.append(word)
self.__word_dictionary_set.add(word)
if y == 1:
# 垃圾短信
self.__spam_count += 1
self.__spam_words_count += len(words)
for word in words:
self.__spam_words.append(word)
self.__word_dictionary_set.add(word)
self.__word_dictionary_size = len(self.__word_dictionary_set)
def word_count(self):
# 不同类别下的词频统计
for word in self.__ham_words:
self.__ham_map[word] = self.__ham_map.setdefault(word, 0) + 1
for word in self.__spam_words:
self.__spam_map[word] = self.__spam_map.setdefault(word, 0) + 1
# 非垃圾短信的概率
self.__ham_probability = self.__ham_count / (self.__ham_count + self.__spam_count)
# 垃圾短信的概率
self.__spam_probability = self.__spam_count / (self._ham_count+ self._spam_count)
4
●
测试集预测
代码清单 13-5 朴素贝叶斯预测函数
def predict(self, X_test):
return [self.predict_one(sentence) for sentence in X_test]
def predict_one(self, sentence):
ham_pro = 0
spam_pro = 0
words = self.data_preprocess(sentence)
for word in words:
ham_pro += math.log(
(self.__ham_map.get(word, 0) + 1) / (self.__ham_count + self.__word_dictionary_size))
spam_pro += math.log(
(self.__spam_map.get(word, 0) + 1) / (self.__spam_count + self.__word_dictionary_size))
ham_pro += math.log(self.__ham_probability)
spam_pro += math.log(self.__spam_probability)
return int(spam_pro >= ham_pro)
5
●
主函数实现
本模块需要将前面实现的所有功能联合起来,对模型进行验证,首先加载数据集,并将数据集区分为训练集和测试集,在训练集上训练模型,并在测试集上验证模型的效果,代码实现如代码清单 13-6所示。
代码清单 13-6 朴素贝叶斯主函数
from sklearn.metrics import recall_score
from sklearn.metrics import precision_score
from sklearn.metrics import classification_report
from sklearn.metrics import accuracy_score
if __name__ == "__main__":
# 加载数据集
data, classTag = getDateSet()
# 设置训练集大小
train_size = 3000
# 训练集
train_X = data[:train_size]
train_y = classTag[:train_size]
# 测试集
test_X = data[train_size:]
test_y = classTag[train_size:]
# 在训练集上训练模型
nb_model = NaiveBayes()
nb_model.fit(train_X, train_y)
# 在测试集上得到预测结果
pre_y = nb_model.predict(test_X)
# 模型评价
accuracy_score_value = accuracy_score(test_y, pre_y)
recall_score_value = recall_score(test_y, pre_y)
precision_score_value = precision_score(test_y, pre_y)
classification_report_value = classification_report(test_y, pre_y)
print("准确率:", accuracy_score_value)
print("召回率:", recall_score_value)
print("精确率:", precision_score_value)
print(classification_report_value)
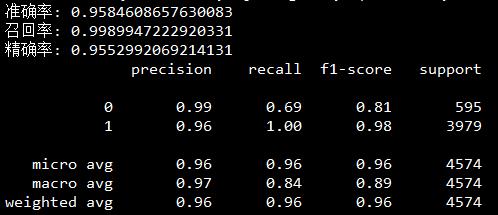
图 13-5 训练集1000条数据预测效果
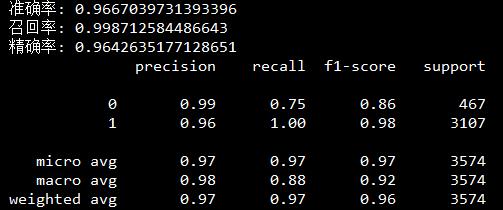
图 13-6 训练集2000条数据预测效果
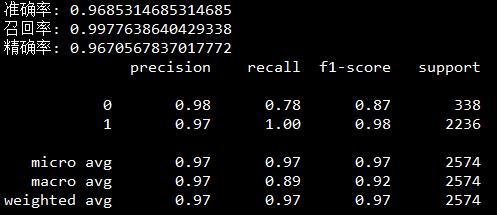
图 13-7 训练集3000条数据预测效果
04
参考教材
《Python机器学习实战-微课视频版》
ISBN:978-7-302-57641-9
吕云翔 王渌汀 袁琪 张凡 韩雪婷 编著
定价:59.8元
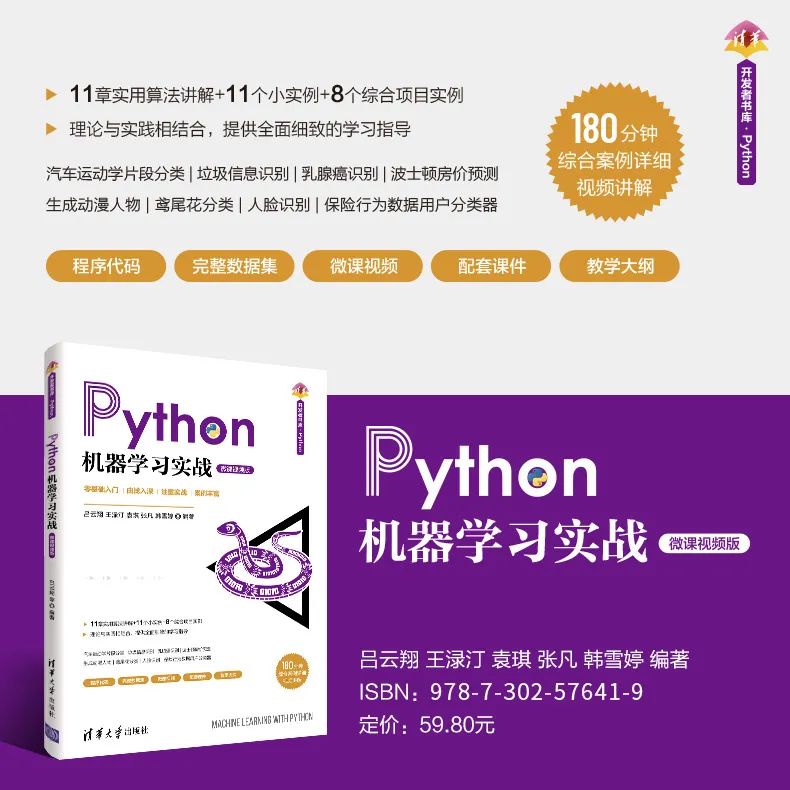
扫码优惠购书
以上是关于机器学习实战︱基于朴素贝叶斯算法的垃圾信息的识别的主要内容,如果未能解决你的问题,请参考以下文章