回顾丨全面回顾2020年图机器学习进展,12位大神论道寄望2021年大爆发!
Posted 中国人工智能学会
tags:
篇首语:本文由小常识网(cha138.com)小编为大家整理,主要介绍了回顾丨全面回顾2020年图机器学习进展,12位大神论道寄望2021年大爆发!相关的知识,希望对你有一定的参考价值。
转自 AI科技评论
作者 |Michael Bronstein
编译 | Mr.Bear
编辑 | 陈大鑫
1
消息传递机制
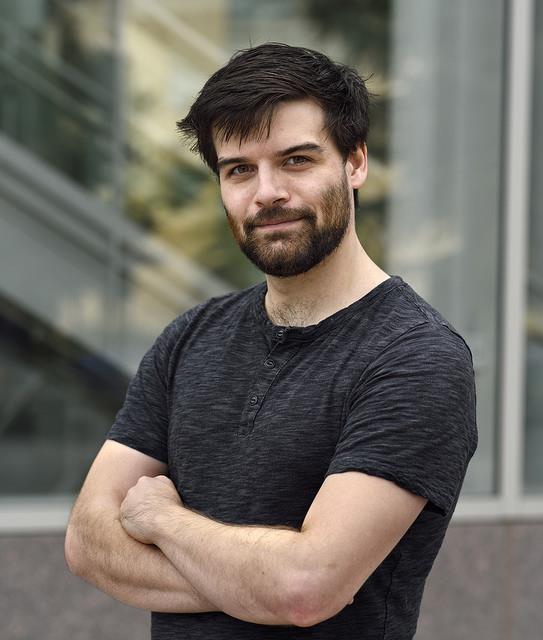
「2020 年,图机器学习领域开始受限于消息传递范式的本质缺陷。」
2
算法推理
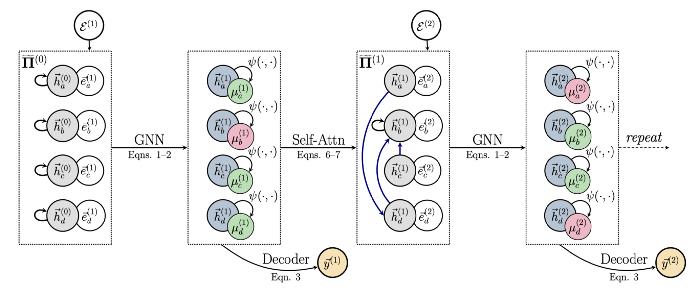
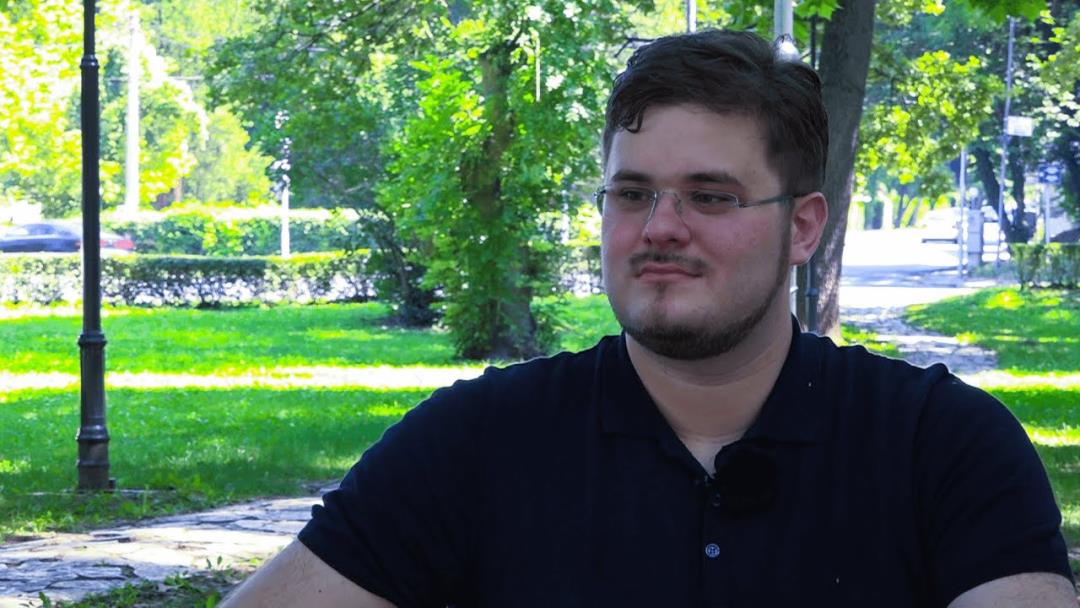
显然,图表征学习在 2020 年已经不可逆转地成为了机器学习领域最受瞩目的课题之一。
3
关系结构发现
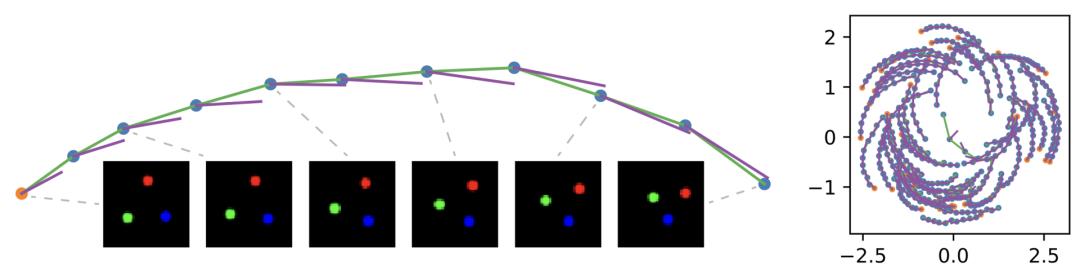
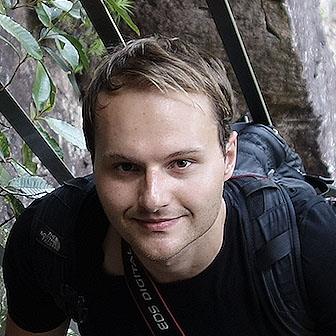
自从最近基于 GNN 的模型被广泛采用以来,图机器学习社区中一个值得注意的趋势是: 将计算结构与数据结构分离开来。
4
表达能力

图神经网络的表达能力是 2020 年图机器学习领域的核心问题之一。
5
可扩展性
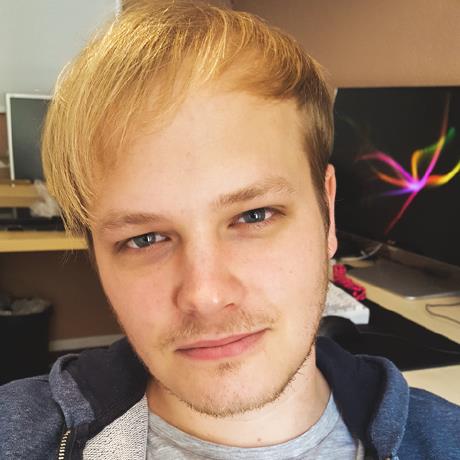
2020 年,解决 GNN 的可扩展性问题是图机器学习研究领域最热门的话题之一。
6
动态图
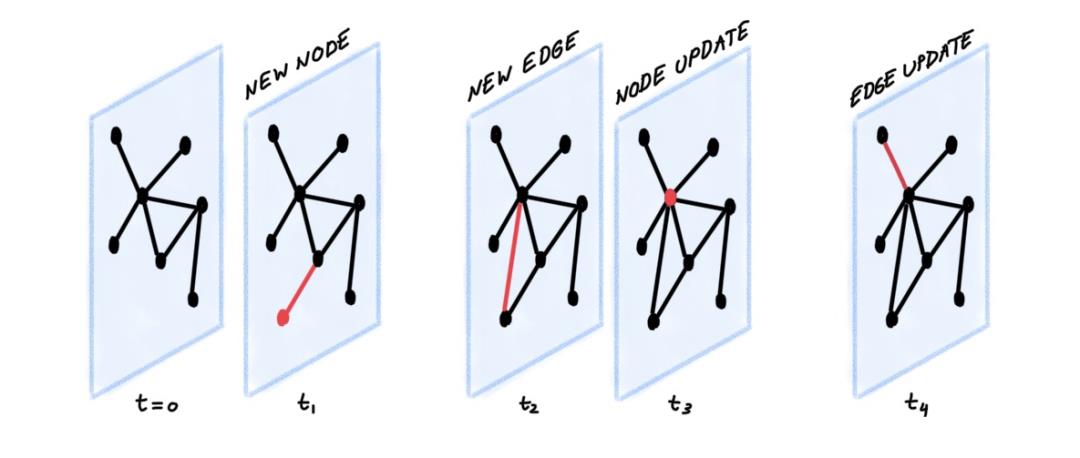
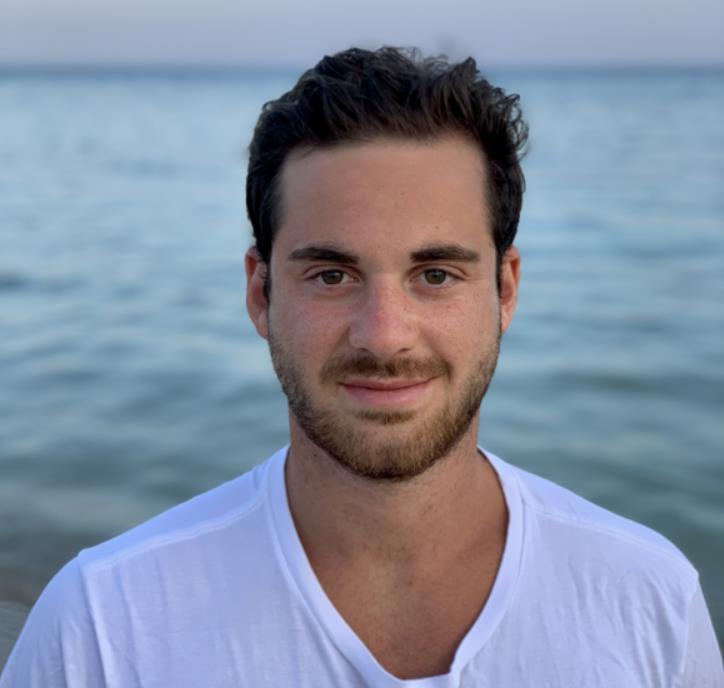
许多有趣的图机器学习应用本质上是动态的,其中图的拓扑结构和属性都会随着时间演化。
7
新的硬件
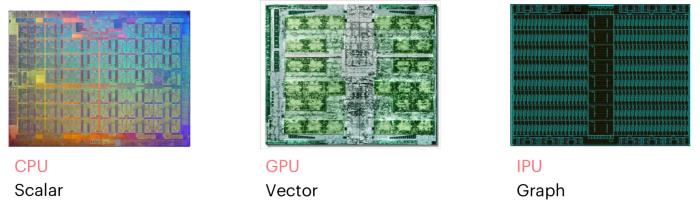
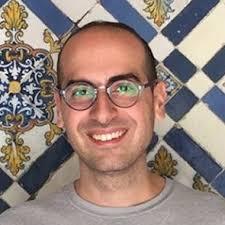
在我所共事过的人中,我想不出还有谁没有在生产中部署过图神经网络,或者正考虑这么做。
8
在工业界的应用
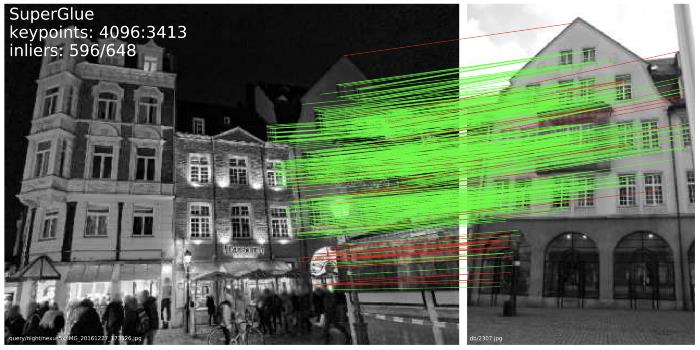
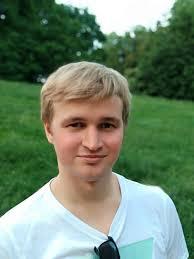
对于图机器学习研究领域来说,2020 年是令人震惊的一年。所有的机器学习会议都包含 10-20% 有关该领域的投稿。因此,每个人都可以找到自己感兴趣的有关图的课题。
9
在物理学的应用
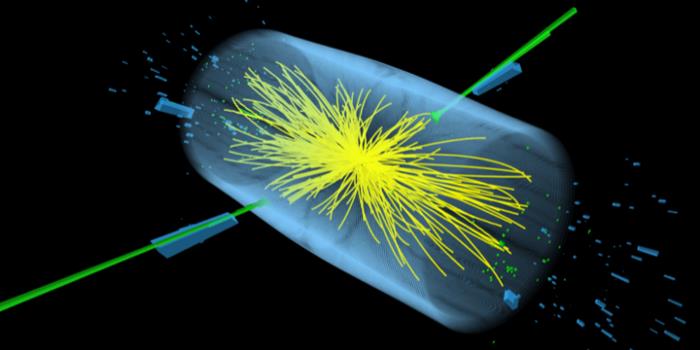
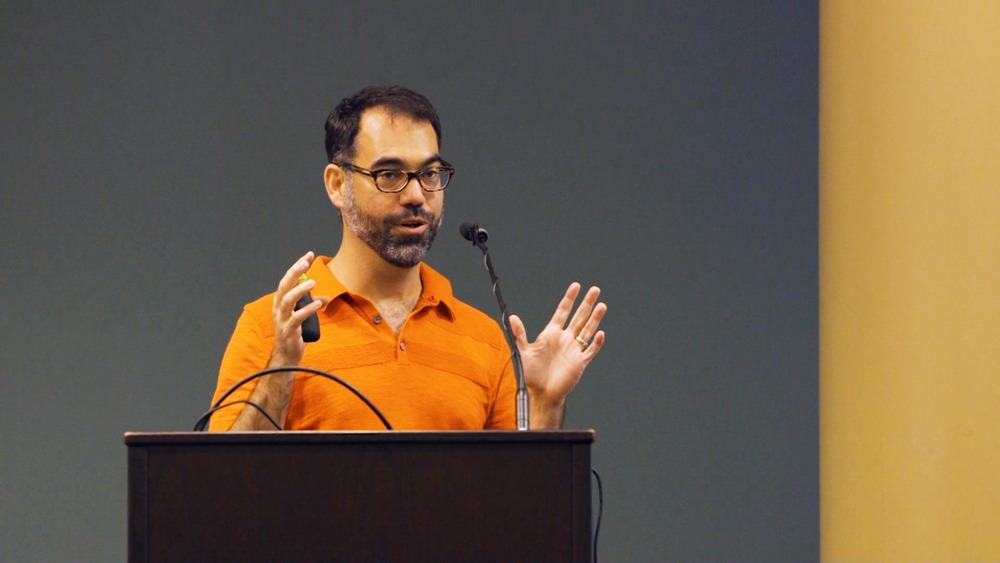
我们惊讶地发现,图机器学习在过去的两年中已经在物理学研究领域盛行。
10
在医疗领域的应用
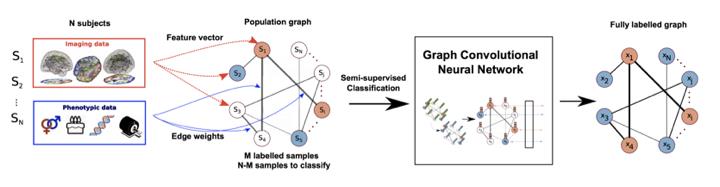
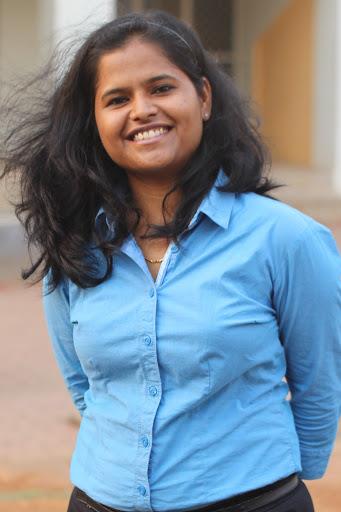
在医学领域中,图机器学习转变了我们分析多模态数据的方式,这种方式与医学专家在临床中根据所有可知的维度观察病人情况的方式非常相近。
11
在生物信息学的应用
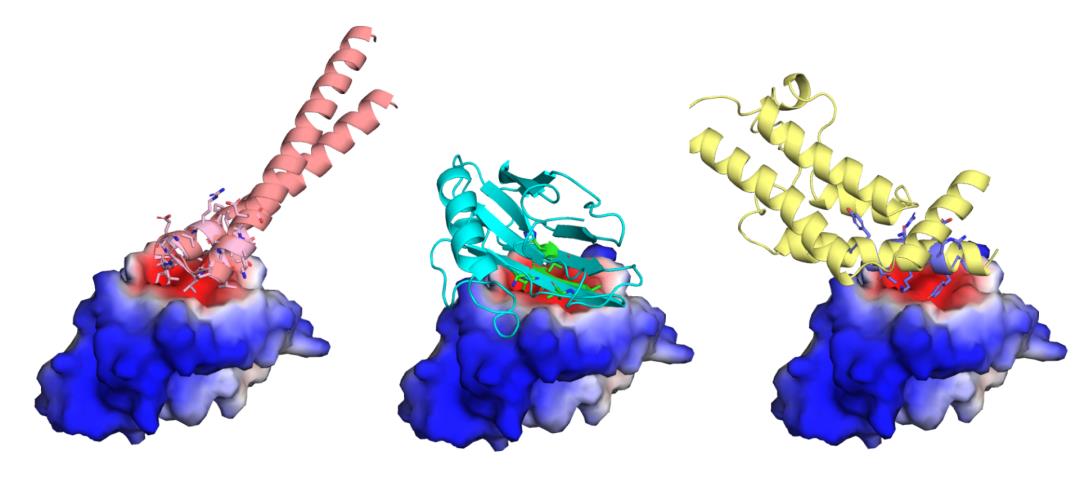
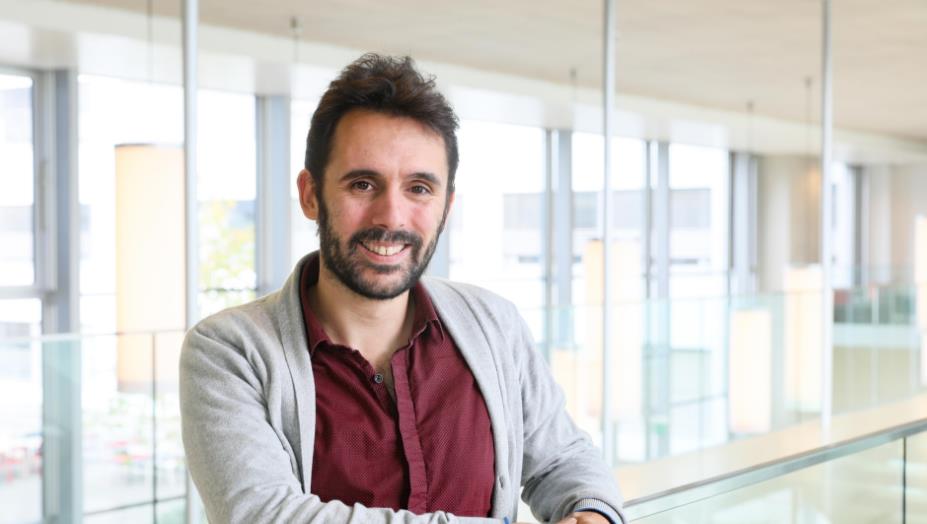
2020 年,作为生物信息学领域中的一个关键问题,蛋白质结构预测取得了激动人心的进展,分子表面的化学和几何模式对蛋白质功能至关重要。
12
在生命科学的应用
看到图机器学习在 2020 年进军生命科学领域令人十分振奋。
参考文献
[1] U. Alon and E. Yahav, On the bottleneck of graph neural networks and its practical implications (2020) arXiv:2006.05205.
[2] Q. Li, Z. Han, X.-M. Wu, Deeper insights into graph convolutional networks for semi-supervised learning (2019) Proc. AAAI.
[3] K. Xu et al. How powerful are graph neural networks? (2019) Proc. ICLR.
[4] C. Morris et al. Weisfeiler and Leman go neural: Higher-order graph neural networks (2019) Proc. AAAI.
[5] K. Xu et al. What can neural networks reason about? (2019) arXiv:1905.13211.
[6] Q. Huang et al. Combining label propagation and simple models out-performs graph neural networks (2020) arXiv:2010.13993.
[7] F. Frasca et al. SIGN: Scalable Inception Graph Neural Networks (2020) arXiv:2004.11198.
[8] A. Graves, G. Wayne, and I. Danihelka, Neural Turing Machines (2014) arXiv:1410.5401.
[9] A. Graves et al. Hybrid computing using a neural network with dynamic external memory (2016). Nature 538:471–476.
[10] G. Yehuda, M. Gabel, and A. Schuster. It’s not what machines can learn, it’s what we cannot teach (2020) arXiv:2002.09398.
[11] K. Xu et al. How neural networks extrapolate: From feedforward to graph neural networks (2020) arXiv:2009.11848.
[12] P. Veličković et al., Neural execution of graph algorithms (2019) arXiv:1910.10593.
[13] O. Richter and R. Wattenhofer, Normalized attention without probability cage (2020) arXiv:2005.09561.
[14] H. Tang et al., Towards scale-invariant graph-related problem solving by iterative homogeneous graph neural networks (2020) arXiv:2010.13547.
[15] P. Veličković et al. Pointer Graph Networks (2020) Proc. NeurIPS.
[16] Y. Yan et al. Neural execution engines: Learning to execute subroutines (2020) Proc. ICLR.
[17] C. K. Joshi et al. Learning TSP requires rethinking generalization (2020) arXiv:2006.07054.
[18] A. Deac et al. XLVIN: eXecuted Latent Value Iteration Nets (2020) arXiv:2010.13146.
[19] S. Löwe et al., Amortized Causal Discovery: Learning to infer causal graphs from time-series data (2020) arXiv:2006.10833.
[20] Y. Li et al., Causal discovery in physical systems from videos (2020) Proc. NeurIPS.
[21] D. Bieber et al., Learning to execute programs with instruction pointer attention graph neural networks (2020) Proc. NeurIPS.
[22] A. Kazi et al., Differentiable Graph Module (DGM) for graph convolutional networks (2020) arXiv:2002.04999
[23] D. D. Johnson, H. Larochelle, and D. Tarlow., Learning graph structure with a finite-state automaton layer (2020). arXiv:2007.04929.
[24] T. Pfaff et al., Learning mesh-based simulation with graph networks (2020) arXiv:2010.03409.
[25] T. Kipf et al., Contrastive learning of structured world models (2020) Proc. ICLR
[26] F. Locatello et al., Object-centric learning with slot attention (2020) Proc. NeurIPS.
[27] W. Azizian and M. Lelarge, Characterizing the expressive power of invariant and equivariant graph neural networks (2020) arXiv:2006.15646.
[28] A. Loukas, What graph neural networks cannot learn: depth vs width (2020) Proc. ICLR.
[29] Z. Chen et al., Can graph neural networks count substructures? (2020) Proc. NeurIPS.
[30] A. Bojchevski et al., Scaling graph neural networks with approximate PageRank (2020) Proc. KDD.
[31] E. Rossi et al., Temporal Graph Networks for deep learning on dynamic graphs (2020) arXiv:2006.10637.
[32] S. Kumar, X. Zhang, and J. Leskovec, Predicting dynamic embedding trajectory in temporal interaction networks (2019) Proc. KDD.
[33] R. Trivedi et al., DyRep: Learning representations over dynamic graphs (2019) Proc. ICLR.
[34] D. Xu et al., Inductive representation learning on temporal graphs (2019) Proc. ICLR.
[35] M. Noorshams, S. Verma, and A. Hofleitner, TIES: Temporal Interaction Embeddings for enhancing social media integrity at Facebook (2020) arXiv:2002.07917.
[36] X. Wang et al., APAN: Asynchronous Propagation Attention Network for real-time temporal graph embedding (2020) arXiv:2011.11545.
[37] E. A. Meirom et al., How to stop epidemics: Controlling graph dynamics with reinforcement learning and graph neural networks (2020) arXiv:2010.05313.
[38] S. Hooker, Hardware lottery (2020), arXiv:2009.06489.
[39] P. E. Sarlin et al., SuperGlue: Learning feature matching with graph neural networks (2020). Proc. CVPR.
[40] S. Ruhk et al., Learning representations of irregular particle-detector geometry with distance-weighted graph networks (2019) arXiv:1902.07987.
[41] J. Shlomi, P. Battaglia, J.-R. Vlimant, Graph Neural Networks in particle physics (2020) arXiv:2007.13681.
[42] J. Krupa et al., GPU coprocessors as a service for deep learning inference in high energy physics (2020) arXiv:2007.10359.
[43] A. Heintz et al., Accelerated charged particle tracking with graph neural networks on FPGAs (2020) arXiv:2012.01563.
[44] M. Cranmer et al., Discovering symbolic models from deep learning with inductive biases (2020) arXiv:2006.11287. Miles Cranmer is unrelated to Kyle Cranmer, though both are co-authors of the paper. See also the video presentation of the paper.
[45] Q. Cai et al., A survey on multimodal data-driven smart healthcare systems: Approaches and applications (2020) IEEE Access 7:133583–133599
[46] K. Gopinath, C. Desrosiers, and H. Lombaert, Graph domain adaptation for alignment-invariant brain surface segmentation (2020) arXiv:2004.00074
[47] J. Liu et al., Identification of early mild cognitive impairment using multi-modal data and graph convolutional networks (2020) BMC Bioinformatics 21(6):1–12
[48] H. E. Manoochehri and M. Nourani, Drug-target interaction prediction using semi-bipartite graph model and deep learning (2020). BMC Bioinformatics 21(4):1–16
[49] Y. Huang and A. C. Chung, Edge-variational graph convolutional networks for uncertainty-aware disease prediction (2020) Proc. MICCAI
[50] L. Cosmo et al., Latent-graph learning for disease prediction (2020) Proc. MICCAI
[51] G. Vivar et al., Simultaneous imputation and disease classification in incomplete medical datasets using Multigraph Geometric Matrix Completion (2020) arXiv:2005.06935.
[52] X. Li and J. Duncan, BrainGNN: Interpretable brain graph neural network for fMRI analysis (2020) bioRxiv:2020.05.16.100057
[53] X. Yu et al., ResGNet-C: A graph convolutional neural network for detection of COVID-19 (2020) Neurocomputing.
[54] P. Gainza et al., Deciphering interaction fingerprints from protein molecular surfaces using geometric deep learning (2020) Nature Methods 17(2):184–192.
[55] F. Sverrisson et al., Fast end-to-end learning on protein surfaces (2020) bioRxiv:2020.12.28.424589.
[56] A. Klimovskaia et al., Poincaré maps for analyzing complex hierarchies in single-cell data (2020) Nature Communications 11.
[57] J. Jumper et al., High accuracy protein structure prediction using deep learning (2020) a.k.a. AlphaFold 2.0 (paper not yet available).
[58] J. M. Stokes et al., A deep learning approach to antibiotic discovery (2020) Cell 180(4):688–702.
[59] D. Morselli Gysi et al., Network medicine framework for identifying drug repurposing opportunities for COVID-19 (2020) arXiv:2004.07229.
以上是关于回顾丨全面回顾2020年图机器学习进展,12位大神论道寄望2021年大爆发!的主要内容,如果未能解决你的问题,请参考以下文章
吴恩达机器学习丨思维导图丨坚持打卡23天——构建知识脉络,回顾总结复盘