鍖诲鑷劧璇█澶勭悊鐩稿叧璧勬簮鏁寸悊
Posted 鏈哄櫒瀛︿範AI绠楁硶宸ョ▼
tags:
篇首语:本文由小常识网(cha138.com)小编为大家整理,主要介绍了鍖诲鑷劧璇█澶勭悊鐩稿叧璧勬簮鏁寸悊相关的知识,希望对你有一定的参考价值。
鍚慉I杞瀷鐨勭▼搴忓憳閮藉叧娉ㄤ簡杩欎釜鍙?/span>馃憞馃憞馃憞
Chinese_medical_NLP
鍖荤枟NLP棰嗗煙锛堜富瑕佸叧娉ㄤ腑鏂囷級 璇勬祴鏁版嵁闆?涓?璁烘枃绛夌浉鍏宠祫婧愩€?/p>
涓枃璇勬祴鏁版嵁闆?/strong>
1. Yidu-S4K锛氬尰娓′簯缁撴瀯鍖?K鏁版嵁闆?/strong>
鏁版嵁闆嗘弿杩帮細
Yidu-S4K 鏁版嵁闆嗘簮鑷狢CKS 2019 璇勬祴浠诲姟涓€锛屽嵆鈥滈潰鍚戜腑鏂囩數瀛愮梾鍘嗙殑鍛藉悕瀹炰綋璇嗗埆鈥濈殑鏁版嵁闆嗭紝鍖呮嫭涓や釜瀛愪换鍔★細
1锛夊尰鐤楀懡鍚嶅疄浣撹瘑鍒細鐢变簬鍥藉唴娌℃湁鍏紑鍙幏寰楃殑闈㈠悜涓枃鐢靛瓙鐥呭巻鍖荤枟瀹炰綋璇嗗埆鏁版嵁闆嗭紝鏈勾搴︿繚鐣欎簡鍖荤枟鍛藉悕瀹炰綋璇嗗埆浠诲姟锛屽2017骞村害鏁版嵁闆嗗仛浜嗕慨璁紝骞堕殢浠诲姟涓€鍚屽彂甯冦€傛湰瀛愪换鍔$殑鏁版嵁闆嗗寘鎷缁冮泦鍜屾祴璇曢泦銆?/p>
2锛夊尰鐤楀疄浣撳強灞炴€ф娊鍙栵紙璺ㄩ櫌杩佺Щ锛夛細鍦ㄥ尰鐤楀疄浣撹瘑鍒殑鍩虹涓婏紝瀵归瀹氫箟瀹炰綋灞炴€ц繘琛屾娊鍙栥€傛湰浠诲姟涓鸿縼绉诲涔犱换鍔★紝鍗冲湪鍙彁渚涚洰鏍囧満鏅皯閲忔爣娉ㄦ暟鎹殑鎯呭喌涓嬶紝閫氳繃鍏朵粬鍦烘櫙鐨勬爣娉ㄦ暟鎹強闈炴爣娉ㄦ暟鎹繘琛岀洰鏍囧満鏅殑璇嗗埆浠诲姟銆傛湰瀛愪换鍔$殑鏁版嵁闆嗗寘鎷缁冮泦锛堥潪鐩爣鍦烘櫙鍜岀洰鏍囧満鏅殑鏍囨敞鏁版嵁銆佸悇涓満鏅殑闈炴爣娉ㄦ暟鎹級鍜屾祴璇曢泦锛堢洰鏍囧満鏅殑鏍囨敞鏁版嵁
http://openkg.cn/dataset/yidu-s4k
鎻愬彇鐮侊細flql
2.鐟為噾鍖婚櫌绯栧翱鐥呮暟鎹泦
鏁版嵁闆嗘弿杩帮細
鏁版嵁闆嗘潵鑷ぉ姹犲ぇ璧涖€傛鏁版嵁闆嗘棬鍦ㄩ€氳繃绯栧翱鐥呯浉鍏崇殑鏁欑涔︺€佺爺绌惰鏂囨潵鍋氱硸灏跨梾鏂囩尞鎸栨帢骞舵瀯寤虹硸灏跨梾鐭ヨ瘑鍥捐氨銆傚弬璧涢€夋墜闇€瑕佽璁¢珮鍑嗙‘鐜囷紝楂樻晥鐨勭畻娉曟潵鎸戞垬杩欎竴绉戝闅鹃銆傜涓€璧涘璇鹃涓衡€滃熀浜庣硸灏跨梾涓村簥鎸囧崡鍜岀爺绌惰鏂囩殑瀹炰綋鏍囨敞鏋勫缓鈥濓紝绗簩璧涘璇鹃涓衡€滃熀浜庣硸灏跨梾涓村簥鎸囧崡鍜岀爺绌惰鏂囩殑瀹炰綋闂村叧绯绘瀯寤衡€濄€?/p>
瀹樻柟鎻愪緵鐨勬暟鎹彧鍖呭惈璁粌闆嗭紝鐪熸鐢ㄤ簬鏈€缁堟帓鍚嶇殑娴嬭瘯闆嗘病鏈夌粰鍑恒€?/p>
https://tianchi.aliyun.com/competition/entrance/231687/information
鎻愬彇鐮侊細0c54
3.Yidu-N7K锛氬尰娓′簯鏍囧噯鍖?K鏁版嵁闆?/strong>
鏁版嵁闆嗘弿杩帮細
Yidu-N4K 鏁版嵁闆嗘簮鑷狢HIP 2019 璇勬祴浠诲姟涓€锛屽嵆鈥滀复搴婃湳璇爣鍑嗗寲浠诲姟鈥濈殑鏁版嵁闆嗐€?/p>
涓村簥鏈鏍囧噯鍖栦换鍔℃槸鍖诲缁熻涓笉鍙垨缂虹殑涓€椤逛换鍔°€備复搴婁笂锛屽叧浜庡悓涓€绉嶈瘖鏂€佹墜鏈€佽嵂鍝併€佹鏌ャ€佸寲楠屻€佺棁鐘剁瓑寰€寰€浼氭湁鎴愮櫨涓婂崈绉嶄笉鍚岀殑鍐欐硶銆傛爣鍑嗗寲锛堝綊涓€锛夎瑙e喅鐨勯棶棰樺氨鏄负涓村簥涓婂悇绉嶄笉鍚岃娉曟壘鍒板搴旂殑鏍囧噯璇存硶銆傛湁浜嗘湳璇爣鍑嗗寲鐨勫熀纭€锛岀爺绌朵汉鍛樻墠鍙鐢靛瓙鐥呭巻杩涜鍚庣画鐨勭粺璁″垎鏋愩€傛湰璐ㄤ笂锛屼复搴婃湳璇爣鍑嗗寲浠诲姟涔熸槸璇箟鐩镐技搴﹀尮閰嶄换鍔$殑涓€绉嶃€備絾鏄敱浜庡師璇嶈〃杩版柟寮忚繃浜庡鏍凤紝鍗曚竴鐨勫尮閰嶆ā鍨嬪緢闅捐幏寰楀緢濂界殑鏁堟灉銆?/p>
http://openkg.cn/dataset/yidu-n7k
鍗曡偐鍖咃紡鍙岃偐鍖咃紡鏂滄寧鍖咃紡鎵嬫彁鍖咃紡鑳稿寘锛忔梾琛屽寘锛忎笂璇句功鍖?锛忎釜鎬у竷琚嬬瓑鍚勫紡鍖呴グ鎸戦€?/span>
https://shop585613237.taobao.com/
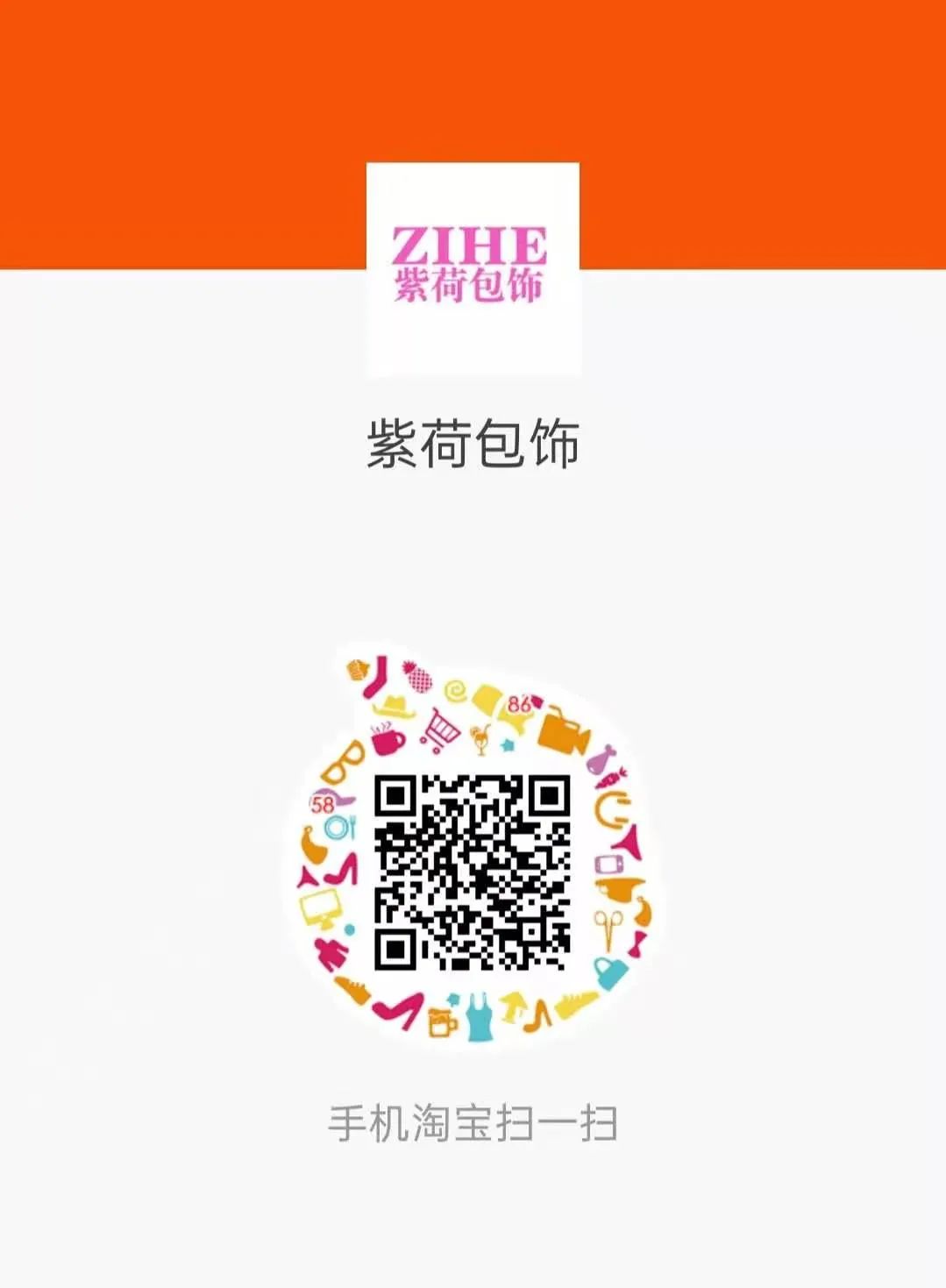
4.涓枃鍖诲闂瓟鏁版嵁闆?/strong>
鏁版嵁闆嗘弿杩帮細
涓枃鍖昏嵂鏂归潰鐨勯棶绛旀暟鎹泦锛岃秴杩?0涓囨潯銆?/p>
鏁版嵁璇存槑:
questions.csv锛氭墍鏈夌殑闂鍙婂叾鍐呭銆俛nswers.csv 锛氭墍鏈夐棶棰樼殑绛旀銆?/p>
train_candidates.txt锛?dev_candidates.txt锛?test_candidates.txt 锛氬皢涓婅堪涓や釜鏂囦欢杩涜浜嗘媶鍒嗐€?/p>
https://www.kesci.com/home/dataset/5d313070cf76a60036e4b023/document
https://github.com/zhangsheng93/cMedQA2
5.骞冲畨鍖荤枟绉戞妧鐤剧梾闂瓟杩佺Щ瀛︿範姣旇禌
鏁版嵁闆嗘弿杩帮細
鏈姣旇禌鏄痗hip2019涓殑璇勬祴浠诲姟浜岋紝鐢卞钩瀹夊尰鐤楃鎶€涓诲姙銆俢hip2019浼氳璇︽儏瑙侀摼鎺ワ細http://cips-chip.org.cn/evaluation
杩佺Щ瀛︿範鏄嚜鐒惰瑷€澶勭悊涓殑閲嶈涓€鐜紝鍏朵富瑕佺洰鐨勬槸閫氳繃浠庡凡瀛︿範鐨勭浉鍏充换鍔′腑杞Щ鐭ヨ瘑鏉ユ敼杩涙柊浠诲姟鐨勫涔犳晥鏋滐紝浠庤€屾彁楂樻ā鍨嬬殑娉涘寲鑳藉姏銆?/p>
鏈璇勬祴浠诲姟鐨勪富瑕佺洰鏍囨槸閽堝涓枃鐨勭柧鐥呴棶绛旀暟鎹紝杩涜鐥呯闂寸殑杩佺Щ瀛︿範銆傚叿浣撹€岃█锛岀粰瀹氭潵鑷?涓笉鍚岀梾绉嶇殑闂彞瀵癸紝瑕佹眰鍒ゅ畾涓や釜鍙ュ瓙璇箟鏄惁鐩稿悓鎴栬€呯浉杩戙€傛墍鏈夎鏂欐潵鑷簰鑱旂綉涓婃偅鑰呯湡瀹炵殑闂锛屽苟缁忚繃浜嗙瓫閫夊拰浜哄伐鐨勬剰鍥惧尮閰嶆爣娉ㄣ€?/p>
https://www.biendata.com/competition/chip2019/
6.澶╂睜鏂板啝鑲虹値闂彞鍖归厤姣旇禌
鏁版嵁闆嗘弿杩帮細
鏈澶ц禌鏁版嵁鍖呮嫭锛氳劚鏁忎箣鍚庣殑鍖荤枟闂鏁版嵁瀵瑰拰鏍囨敞鏁版嵁銆傚尰鐤楅棶棰樻秹鍙娾€滆偤鐐庘€濄€佲€滄敮鍘熶綋鑲虹値鈥濄€佲€滄敮姘旂鐐庘€濄€佲€滀笂鍛煎惛閬撴劅鏌撯€濄€佲€滆偤缁撴牳鈥濄€佲€滃摦鍠樷€濄€佲€滆兏鑶滅値鈥濄€佲€滆偤姘旇偪鈥濄€佲€滄劅鍐掆€濄€佲€滃挸琛€鈥濈瓑10涓梾绉嶃€?/p>
鏁版嵁鍏卞寘鍚玹rain.csv銆乨ev.csv銆乼est.csv涓変釜鏂囦欢锛屽叾涓粰鍙傝禌閫夋墜鐨勬枃浠跺寘鍚缁冮泦train.csv鍜岄獙璇侀泦dev.csv锛屾祴璇曢泦test.csv 瀵瑰弬璧涢€夋墜涓嶅彲瑙併€?/p>
姣忎竴鏉℃暟鎹敱 Category锛孮uery1锛孮uery2锛孡abel鏋勬垚锛屽垎鍒〃绀洪棶棰樼被鍒€侀棶鍙?銆侀棶鍙?銆佹爣绛俱€侺abel琛ㄧず闂彞涔嬮棿鐨勮涔夋槸鍚︾浉鍚岋紝鑻ョ浉鍚岋紝鏍囦负1锛岃嫢涓嶇浉鍚岋紝鏍囦负0銆傚叾涓紝璁粌闆哃abel宸茬煡锛岄獙璇侀泦鍜屾祴璇曢泦Label鏈煡銆?/p>
绀轰緥
绫诲埆锛氳偤鐐?/p>
闂彞1锛氳偤閮ㄥ彂鐐庢槸浠€涔堝師鍥犲紩璧风殑锛?/p>
闂彞2锛氳偤閮ㄥ彂鐐庢槸浠€涔堝紩璧风殑
鏍囩:1
绫诲埆锛氳偤鐐?/p>
闂彞1锛氳偤閮ㄥ彂鐐庢槸浠€涔堝師鍥犲紩璧风殑锛?/p>
闂彞2锛氳偤閮ㄧ値鐥囨湁浠€涔堢棁鐘?/p>
鏍囩:0
https://tianchi.aliyun.com/competition/entrance/231776/information
涓枃鍖诲鐭ヨ瘑鍥捐氨
CMeKG
http://cmekg.pcl.ac.cn/
绠€浠嬶細CMeKG锛圕hinese Medical Knowledge Graph锛夋槸鍒╃敤鑷劧璇█澶勭悊涓庢枃鏈寲鎺樻妧鏈紝鍩轰簬澶ц妯″尰瀛︽枃鏈暟鎹紝浠ヤ汉鏈虹粨鍚堢殑鏂瑰紡鐮斿彂鐨勪腑鏂囧尰瀛︾煡璇嗗浘璋便€侰MeKG鐨勬瀯寤哄弬鑰冧簡ICD銆丄TC銆丼NOMED銆丮eSH绛夋潈濞佺殑鍥介檯鍖诲鏍囧噯浠ュ強瑙勬ā搴炲ぇ銆佸婧愬紓鏋勭殑涓村簥鎸囧崡銆佽涓氭爣鍑嗐€佽瘖鐤楄鑼冧笌鍖诲鐧剧绛夊尰瀛︽枃鏈俊鎭€侰MeKG 1.0鍖呮嫭锛?310绉嶇柧鐥呫€?9853绉嶈嵂鐗╋紙瑗胯嵂銆佷腑鎴愯嵂銆佷腑鑽夎嵂锛夈€?237绉嶈瘖鐤楁妧鏈強璁惧鐨勭粨鏋勫寲鐭ヨ瘑鎻忚堪锛屾兜鐩栫柧鐥呯殑涓村簥鐥囩姸銆佸彂鐥呴儴浣嶃€佽嵂鐗╂不鐤椼€佹墜鏈不鐤椼€侀壌鍒瘖鏂€佸奖鍍忓妫€鏌ャ€侀珮鍗卞洜绱犮€佷紶鎾€斿緞銆佸鍙戠兢浣撱€佸氨璇婄瀹ょ瓑浠ュ強鑽墿鐨勬垚鍒嗐€侀€傚簲鐥囥€佺敤娉曠敤閲忋€佹湁鏁堟湡銆佺蹇岃瘉绛?0浣欑甯歌鍏崇郴绫诲瀷锛孋MeKG鎻忚堪鐨勬蹇靛叧绯诲疄渚嬪強灞炴€т笁鍏冪粍杈?00浣欎竾銆?/p>
鑻辨枃鏁版嵁闆?/p>
PubMedQA: A Dataset for Biomedical Research Question Answering
鏁版嵁闆嗘弿杩帮細鍩轰簬Pubmed鎻愬彇鐨勫尰瀛﹂棶绛旀暟鎹泦銆侾ubMedQA has 1k expert-annotated, 61.2k unlabeled and 211.3k artificially gen- erated QA instances.
https://arxiv.org/abs/1909.06146
鐩稿叧璁烘枃
1.鍖荤枟棰嗗煙棰勮缁僥mbedding
娉細鐩墠娌℃湁鏀堕泦鍒颁腑鏂囧尰鐤楅鍩熺殑寮€婧愰璁粌妯″瀷锛屼互涓嬪垪鍑鸿嫳鏂囪鏂囦緵鍙傝€冦€?/p>
Bio-bert
璁烘枃棰樼洰锛欱ioBERT: a pre-trained biomedical language representation model for biomedical text mining
https://academic.oup.com/bioinformatics/advance-article/doi/10.1093/bioinformatics/btz682/5566506
https://github.com/dmis-lab/biobert
璁烘枃姒傝锛氫互閫氱敤棰嗗煙棰勮缁僢ert涓哄垵濮嬫潈閲嶏紝鍩轰簬Pubmed涓婂ぇ閲忓尰鐤楅鍩熻嫳鏂囪鏂囪缁冦€傚湪澶氫釜鍖荤枟鐩稿叧涓嬫父浠诲姟涓秴瓒奡OTA妯″瀷鐨勮〃鐜般€?/p>
璁烘枃鎽樿锛?/p>
Motivation: Biomedical text mining is becoming increasingly important as the number of biomedical documents rapidly grows. With the progress in natural language processing (NLP), extracting valuable information from bio- medical literature has gained popularity among researchers, and deep learning has boosted the development of ef- fective biomedical text mining models. However, directly applying the advancements in NLP to biomedical text min- ing often yields unsatisfactory results due to a word distribution shift from general domain corpora to biomedical corpora. In this article, we investigate how the recently introduced pre-trained language model BERT can be adapted for biomedical corpora.
Results: We introduce BioBERT (Bidirectional Encoder Representations from Transformers for Biomedical Text Mining), which is a domain-specific language representation model pre-trained on large-scale biomedical corpora. With almost the same architecture across tasks, BioBERT largely outperforms BERT and previous state-of-the-art models in a variety of biomedical text mining tasks when pre-trained on biomedical corpora. While BERT obtains performance comparable to that of previous state-of-the-art models, BioBERT significantly outperforms them on the following three representative biomedical text mining tasks: biomedical named entity recognition (0.62% F1 score improvement), biomedical relation extraction (2.80% F1 score improvement) and biomedical question answering (12.24% MRR improvement). Our analysis results show that pre-training BERT on biomedical corpora helps it to understand complex biomedical texts.
Availability and implementation: We make the pre-trained weights of BioBERT freely available at https://github.com/naver/biobert-pretrained, and the source code for fine-tuning BioBERT available at https://github.com/dmis-lab/biobert.
sci-bert
璁烘枃棰樼洰锛歋CIBERT: A Pretrained Language Model for Scientific Text
https://arxiv.org/abs/1903.10676
https://github.com/allenai/scibert/
璁烘枃姒傝锛欰llenAI 鍥㈤槦鍑哄搧.鍩轰簬Semantic Scholar 涓?110涓? 鏂囩珷璁粌鐨?绉戝棰嗗煙bert.
璁烘枃鎽樿锛歄btaining large-scale annotated data for NLP tasks in the scientific domain is challeng- ing and expensive. We release SCIBERT, a pretrained language model based on BERT (Devlin et al., 2019) to address the lack of high-quality, large-scale labeled scientific data. SCIBERT leverages unsupervised pretraining on a large multi-domain corpus of scientific publications to improve perfor- mance on downstream scientific NLP tasks. We evaluate on a suite of tasks including sequence tagging, sentence classification and dependency parsing, with datasets from a variety of scientific domains. We demon- strate statistically significant improvements over BERT and achieve new state-of-the- art results on several of these tasks. The code and pretrained models are available at https://github.com/allenai/scibert/.
clinical-bert
璁烘枃棰樼洰锛歅ublicly Available Clinical BERT Embeddings
https://www.aclweb.org/anthology/W19-1909/
https://github.com/EmilyAlsentzer/clinicalBERT
璁烘枃姒傝锛氬嚭鑷狽AACL Clinical NLP Workshop 2019.鍩轰簬MIMIC-III鏁版嵁搴撲腑鐨?00涓囦唤鍖荤枟璁板綍璁粌鐨勪复搴婇鍩焍ert.
璁烘枃鎽樿锛欳ontextual word embedding models such as ELMo and BERT have dramatically improved performance for many natural language processing (NLP) tasks in recent months. However, these models have been minimally explored on specialty corpora, such as clinical text; moreover, in the clinical domain, no publicly-available pre-trained BERT models yet exist. In this work, we address this need by exploring and releasing BERT models for clinical text: one for generic clinical text and another for discharge summaries specifically. We demonstrate that using a domain-specific model yields performance improvements on 3/5 clinical NLP tasks, establishing a new state-of-the-art on the MedNLI dataset. We find that these domain-specific models are not as performant on 2 clinical de-identification tasks, and argue that this is a natural consequence of the differences between de-identified source text and synthetically non de-identified task text.
clinical-bert(鍙︿竴鍥㈤槦鐨勭増鏈?
璁烘枃棰樼洰锛欳linicalBert: Modeling Clinical Notes and Predicting Hospital Readmission
https://arxiv.org/abs/1904.05342
https://github.com/kexinhuang12345/clinicalBERT
璁烘枃姒傝锛氬悓鏍峰熀浜嶮IMIC-III鏁版嵁搴?浣嗗彧闅忔満閫夊彇浜?0涓囦唤鍖荤枟璁板綍璁粌鐨勪复搴婇鍩焍ert.
璁烘枃鎽樿锛欳linical notes contain information about patients that goes beyond structured data like lab values and medications. However, clinical notes have been underused relative to structured data, because notes are high-dimensional and sparse. This work develops and evaluates representations of clinical notes using bidirectional transformers (ClinicalBert). Clini- calBert uncovers high-quality relationships between medical concepts as judged by hu- mans. ClinicalBert outperforms baselines on 30-day hospital readmission prediction using both discharge summaries and the first few days of notes in the intensive care unit. Code and model parameters are available.
BEHRT
璁烘枃棰樼洰锛欱EHRT: TRANSFORMER FOR ELECTRONIC HEALTH RECORDS
https://arxiv.org/abs/1907.09538
璁烘枃姒傝锛氳繖绡囪鏂囦腑embedding鏄熀浜庡尰瀛﹀疄浣撹缁冿紝鑰屼笉鏄熀浜庡崟璇嶃€?/p>
璁烘枃鎽樿锛歍oday, despite decades of developments in medicine and the growing interest in precision healthcare, vast majority of diagnoses happen once patients begin to show noticeable signs of illness. Early indication and detection of diseases, however, can provide patients and carers with the chance of early intervention, better disease management, and efficient allocation of healthcare resources. The latest developments in machine learning (more specifically, deep learning) provides a great opportunity to address this unmet need. In this study, we introduce BEHRT: A deep neural sequence transduction model for EHR (electronic health records), capable of multitask prediction and disease trajectory mapping. When trained and evaluated on the data from nearly 1.6 million individuals, BEHRT shows a striking absolute improvement of 8.0-10.8%, in terms of Average Precision Score, compared to the existing state-of-the-art deep EHR models (in terms of average precision, when predicting for the onset of 301 conditions). In addition to its superior prediction power, BEHRT provides a personalised view of disease trajectories through its attention mechanism; its flexible architecture enables it to incorporate multiple heterogeneous concepts (e.g., diagnosis, medication, measurements, and more) to improve the accuracy of its predictions; and its (pre-)training results in disease and patient representations that can help us get a step closer to interpretable predictions.
2.缁艰堪绫绘枃绔?/p>
nature medicine鍙戣〃鐨勭患杩?/p>
璁烘枃棰樼洰锛欰 guide to deep learning in healthcare
https://www.nature.com/articles/s41591-018-0316-z
璁烘枃姒傝锛氬彂琛ㄤ簬nature medicine锛屽寘鍚尰瀛﹂鍩熶笅CV,NLP,寮哄寲瀛︿範绛夋柟闈㈢殑搴旂敤缁艰堪銆?/p>
璁烘枃鎽樿锛欻ere we present deep-learning techniques for healthcare, centering our discussion on deep learning in computer vision, natural language processing, reinforcement learning, and generalized methods. We describe how these computational techniques can impact a few key areas of medicine and explore how to build end-to-end systems. Our discussion of computer vision focuses largely on medical imaging, and we describe the application of natural language processing to domains such as electronic health record data. Similarly, reinforcement learning is discussed in the context of robotic-assisted surgery, and generalized deep- learning methods for genomics are reviewed.
3.鐢靛瓙鐥呭巻鐩稿叧鏂囩珷
Transfer Learning from Medical Literature for Section Prediction in Electronic Health Records
https://www.aclweb.org/anthology/D19-1492/
璁烘枃姒傝锛氬彂琛ㄤ簬EMNLP2019銆傚熀浜庡皯閲廼n-domain鏁版嵁鍜屽ぇ閲弌ut-of-domain鏁版嵁杩涜EHR鐩稿叧鐨勮縼绉诲涔犮€?/p>
璁烘枃鎽樿锛歴ections such as Assessment and Plan, So- cial History, and Medications. These sec- tions help physicians find information easily and can be used by an information retrieval system to return specific information sought by a user. However, it is common that the exact format of sections in a particular EHR does not adhere to known patterns. There- fore, being able to predict sections and headers in EHRs automatically is beneficial to physi- cians. Prior approaches in EHR section pre- diction have only used text data from EHRs and have required significant manual annota- tion. We propose using sections from med- ical literature (e.g., textbooks, journals, web content) that contain content similar to that found in EHR sections. Our approach uses data from a different kind of source where la- bels are provided without the need of a time- consuming annotation effort. We use this data to train two models: an RNN and a BERT- based model. We apply the learned models along with source data via transfer learning to predict sections in EHRs. Our results show that medical literature can provide helpful su- pervision signal for this classification task.
4.鍖诲鍏崇郴鎶藉彇
Leveraging Dependency Forest for Neural Medical Relation Extraction
https://www.aclweb.org/anthology/D19-1020/
璁烘枃姒傝锛氬彂琛ㄤ簬EMNLP 2019. 鍩轰簬dependency forest鏂规硶锛屾彁鍗囧鍖诲璇彞涓緷瀛樺叧绯荤殑鍙洖鐜囷紝鍚屾椂寮曡繘浜嗕竴閮ㄥ垎鍣0锛屽熀浜庡浘寰幆缃戠粶杩涜鐗瑰緛鎻愬彇锛屾彁渚涗簡鍦ㄥ尰鐤楀叧绯绘娊鍙栦腑浣跨敤渚濆瓨鍏崇郴锛屽悓鏃跺噺灏戣宸紶閫掔殑涓€绉嶆€濊矾銆?/p>
璁烘枃鎽樿锛歁edical relation extraction discovers relations between entity mentions in text, such as research articles. For this task, dependency syntax has been recognized as a crucial source of features. Yet in the medical domain, 1-best parse trees suffer from relatively low accuracies, diminishing their usefulness. We investigate a method to alleviate this problem by utilizing dependency forests. Forests contain more than one possible decisions and therefore have higher recall but more noise compared with 1-best outputs. A graph neural network is used to represent the forests, automatically distinguishing the useful syntactic information from parsing noise. Results on two benchmarks show that our method outperforms the standard tree-based methods, giving the state-of-the-art results in the literature.
5.鍖诲鐭ヨ瘑鍥捐氨
Learning a Health Knowledge Graph from Electronic Medical Records
https://www.nature.com/articles/s41598-017-05778-z
璁烘枃姒傝锛氬彂琛ㄤ簬nature scientificreports锛?017锛? 鍩轰簬27涓囦綑浠界數瀛愮梾鍘嗘瀯寤虹殑鐤剧梾-鐥囩姸鐭ヨ瘑鍥捐氨銆?/p>
璁烘枃鎽樿锛欴emand for clinical decision support systems in medicine and self-diagnostic symptom checkers has substantially increased in recent years. Existing platforms rely on knowledge bases manually compiled through a labor-intensive process or automatically derived using simple pairwise statistics. This study explored an automated process to learn high quality knowledge bases linking diseases and symptoms directly from electronic medical records. Medical concepts were extracted from 273,174 de-identified patient records and maximum likelihood estimation of three probabilistic models was used to automatically construct knowledge graphs: logistic regression, naive Bayes classifier and a Bayesian network using noisy OR gates. A graph of disease-symptom relationships was elicited from the learned parameters and the constructed knowledge graphs were evaluated and validated, with permission, against Google鈥檚 manually-constructed knowledge graph and against expert physician opinions. Our study shows that direct and automated construction of high quality health knowledge graphs from medical records using rudimentary concept extraction is feasible. The noisy OR model produces a high quality knowledge graph reaching precision of 0.85 for a recall of 0.6 in the clinical evaluation. Noisy OR significantly outperforms all tested models across evaluation frameworks (p鈥?lt;鈥?.01).
6.杈呭姪璇婃柇
Evaluation and accurate diagnoses of pediatric diseases using artificial intelligence
https://www.nature.com/articles/s41591-018-0335-9
璁烘枃姒傝锛氳鏂囩珷鐢卞箍宸炲競濡囧コ鍎跨鍖荤枟涓績涓庝緷鍥惧尰鐤楃瓑浼佷笟鍜岀鐮旀満鏋勫叡鍚屽畬鎴愶紝鍩轰簬鏈哄櫒瀛︿範鐨勮嚜鐒惰瑷€澶勭悊锛圢LP锛夋妧鏈疄鐜颁笉杈撲汉绫诲尰鐢熺殑寮哄ぇ璇婃柇鑳藉姏锛屽苟鍏峰澶氬満鏅殑搴旂敤鑳藉姏銆傛嵁浠嬬粛锛岃繖鏄叏鐞冮娆″湪椤剁骇鍖诲鏉傚織鍙戣〃鏈夊叧鑷劧璇█澶勭悊锛圢LP锛夋妧鏈熀浜庣數瀛愬仴搴疯褰曪紙EHR锛夊仛涓村簥鏅鸿兘璇婃柇鐨勭爺绌舵垚鏋滐紝涔熸槸鍒╃敤浜哄伐鏅鸿兘鎶€鏈瘖鏂効绉戠柧鐥呯殑閲嶇绉戠爺鎴愭灉銆?/p>
璁烘枃鎽樿锛欰rtificial intelligence (AI)-based methods have emerged as powerful tools to transform medical care. Although machine learning classifiers (MLCs) have already demonstrated strong performance in image-based diagnoses, analysis of diverse and massive electronic health record (EHR) data remains challenging. Here, we show that MLCs can query EHRs in a manner similar to the hypothetico-deductive reasoning used by physicians and unearth associations that previous statistical methods have not found. Our model applies an automated natural language processing system using deep learning techniques to extract clinically relevant information from EHRs. In total, 101.6 million data points from 1,362,559 pediatric patient visits presenting to a major referral center were analyzed to train and validate the framework. Our model demonstrates high diagnostic accuracy across multiple organ systems and is comparable to experienced pediatricians in diagnosing common childhood diseases. Our study provides a proof of concept for implementing an AI-based system as a means to aid physicians in tackling large amounts of data, augmenting diagnostic evaluations, and to provide clinical decision support in cases of diagnostic uncertainty or complexity. Although this impact may be most evident in areas where healthcare providers are in relative shortage, the benefits of such an AI system are likely to be universal.
涓枃鍖荤枟棰嗗煙璇枡
鍖诲鏁欐潗+鍩硅鑰冭瘯 锛堝叡57G锛?/p>
璇枡璇存槑锛氭牴鎹璞嗙摚閾炬帴鏁寸悊銆傛暣鍚堝埌涓€涓枃浠跺す鍐咃紝渚夸簬淇濆瓨銆傚幓鎺変簡鍏朵腑瑙嗛閮ㄥ垎銆?/p>
鎻愬彇鐮侊細xd0c
鍝堝伐澶с€婂ぇ璇嶆灄銆嬪紑鏀?5涓囨牳蹇冨疄浣撹瘝鍙婄浉鍏虫蹇点€佸叧绯诲垪琛紙鍖呭惈涓嵂/鍖婚櫌/鐢熺墿 绫诲埆锛?/p>
璇枡璇存槑:鍝堝伐澶у紑婧愪簡銆婂ぇ璇嶆灄銆嬩腑鐨?5涓囩殑鏍稿績瀹炰綋璇嶏紝浠ュ強杩欎簺鏍稿績瀹炰綋璇嶅搴旂殑缁嗙矑搴︽蹇佃瘝锛堝叡1.8涓囨蹇佃瘝锛?00涓囧疄浣?姒傚康鍏冪粍锛夛紝杩樻湁鐩稿叧鐨勫叧绯讳笁鍏冪粍锛堝叡300涓囷級銆傝繖75涓囨牳蹇冨疄浣撳垪琛ㄦ兜鐩栦簡甯歌鐨勪汉鍚嶃€佸湴鍚嶃€佺墿鍝佸悕绛夋湳璇€傛蹇佃瘝鍒楄〃鍒欏寘鍚簡缁嗙矑搴︾殑瀹炰綋姒傚康淇℃伅銆傚€熷姪浜庣粏绮掑害鐨勪笂浣嶆蹇靛眰娆$粨鏋勫拰涓板瘜鐨勫疄浣撻棿鍏崇郴锛屾湰娆″紑婧愮殑鏁版嵁鑳藉涓轰汉鏈哄璇濄€佹櫤鑳芥帹鑽愩€佺瓑搴旂敤鎶€鏈彁渚涙暟鎹敮鎸併€?/p>
http://101.200.120.155/browser/
鎻愬彇鐮侊細mwmj
寮€婧愬伐鍏峰寘
鍒嗚瘝宸ュ叿
PKUSEG
https://github.com/lancopku/pkuseg-python
椤圭洰璇存槑锛氬寳浜ぇ瀛︽帹鍑虹殑澶氶鍩熶腑鏂囧垎璇嶅伐鍏凤紝鏀寔閫夋嫨鍖诲棰嗗煙銆?/p>
宸ヤ笟绾т骇鍝佽В鍐虫柟妗?/p>
鐏靛尰鏅烘収
https://01.baidu.com/index.html
宸︽墜鍖荤敓
https://open.zuoshouyisheng.com/
鍙嬫儏閾炬帴
awesome_Chinese_medical_NLP
https://github.com/GanjinZero/awesome_Chinese_medical_NLP
涓枃NLP鏁版嵁闆嗘悳绱?/p>
https://www.cluebenchmarks.com/dataSet_search.html
https://github.com/lrs1353281004/Chinese_medical_NLP
闃呰杩囨湰鏂囩殑浜鸿繕鐪嬩簡浠ヤ笅鏂囩珷锛?/strong>
涓嶆柇鏇存柊璧勬簮
娣卞害瀛︿範銆佹満鍣ㄥ涔犮€佹暟鎹垎鏋愩€乸ython
鏈哄ぇ鏁版嵁鎶€鏈笌鏈哄櫒瀛︿範宸ョ▼
以上是关于鍖诲鑷劧璇█澶勭悊鐩稿叧璧勬簮鏁寸悊的主要内容,如果未能解决你的问题,请参考以下文章
2020-2021骞翠箣闂?65+寮€鍙戣祫婧愭暣鐞?/a>
NLP-BERT 璋锋瓕鑷劧璇█澶勭悊妯″瀷锛欱ERT-鍩轰簬pytorch