Flink Collector Output 接口源码解析
Posted JasonLee实时计算
tags:
篇首语:本文由小常识网(cha138.com)小编为大家整理,主要介绍了Flink Collector Output 接口源码解析相关的知识,希望对你有一定的参考价值。
在 Flink 中 Collector 接口主要用于 operator 发送(输出)元素,Output 接口是对 Collector 接口的扩展,增加了发送 WaterMark 的功能,在 Flink 里面只要涉及到数据的传递都必须实现这两个接口,下面就来梳理一下这些接口的源码。
Output Collector UML 图
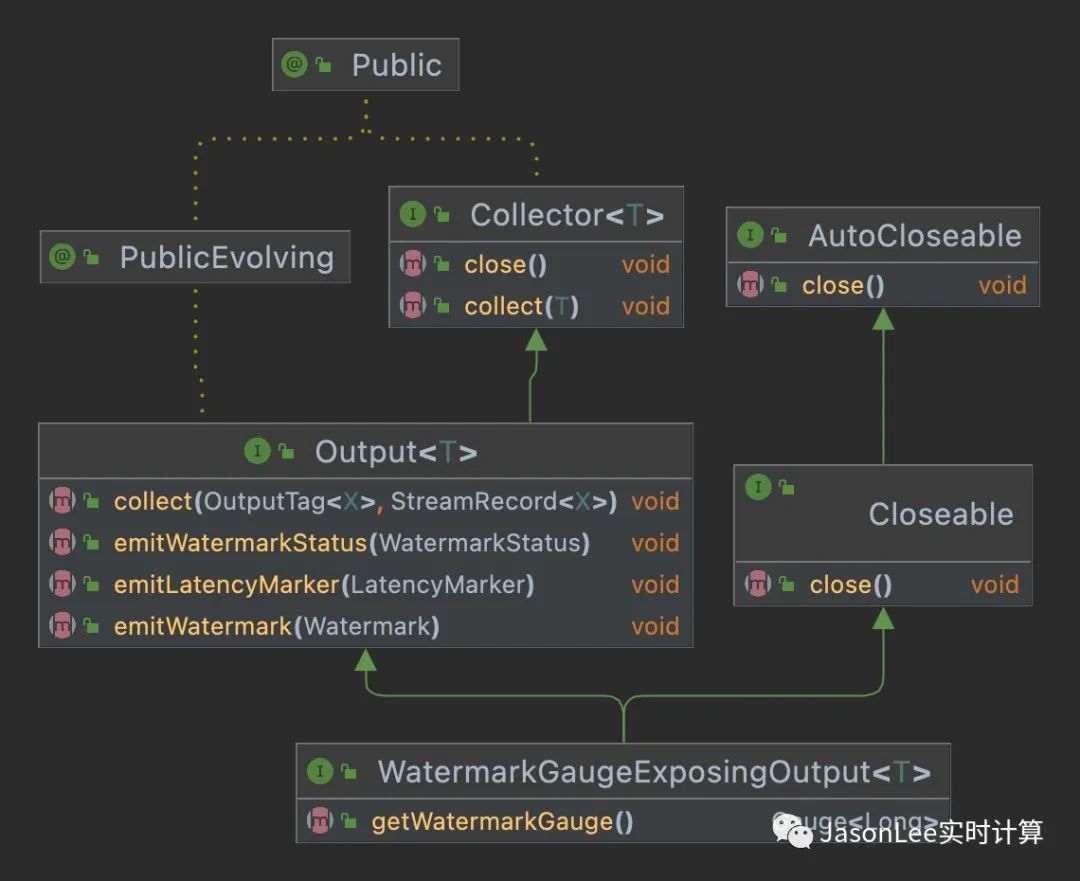
Collector 接口只有 2 个方法:
collect(T record) 用于正常流输出数据。
close() 关闭 Output ,如果任何数据被缓冲,则该数据将被刷新。
Output 接口有 4 个方法:
emitWatermark(Watermark mark) 从 operator 发出 Watermark。此水印将广播到所有下游所有 operator。
emitWatermarkStatus(WatermarkStatus watermarkStatus) 发送水印状态。
collect(OutputTagoutputTag, StreamRecordrecord) 发送数据,这个方法和 Collector 接口中的 collect 方法作用是一样的,但是这个 collect 方法多了一个 OutputTag 参数,也就是说这个方法主要用在侧流输出场景下。
emitLatencyMarker(LatencyMarker latencyMarker) 发送 LatencyMarker 它是一种特殊的数据,用来测量数据的延迟。
WatermarkGaugeExposingOutput 接口只有 1 个方法:
getWatermarkGauge() 用来获取 WatermarkGauge,它是测量最后发出的水印。
我们今天主要说的是 collect 方法,也就是发送真实数据的方法,Output 接口的实现类是非常多的,因为只要你想发送数据就必须实现这个接口,那在众多的实现类里有几个是比较重要的,下面我会挑出 7 个常见的实现类进行介绍,我们先来看下面的 Output 实现类的 UML 类图。
Output 实现类 UML 图
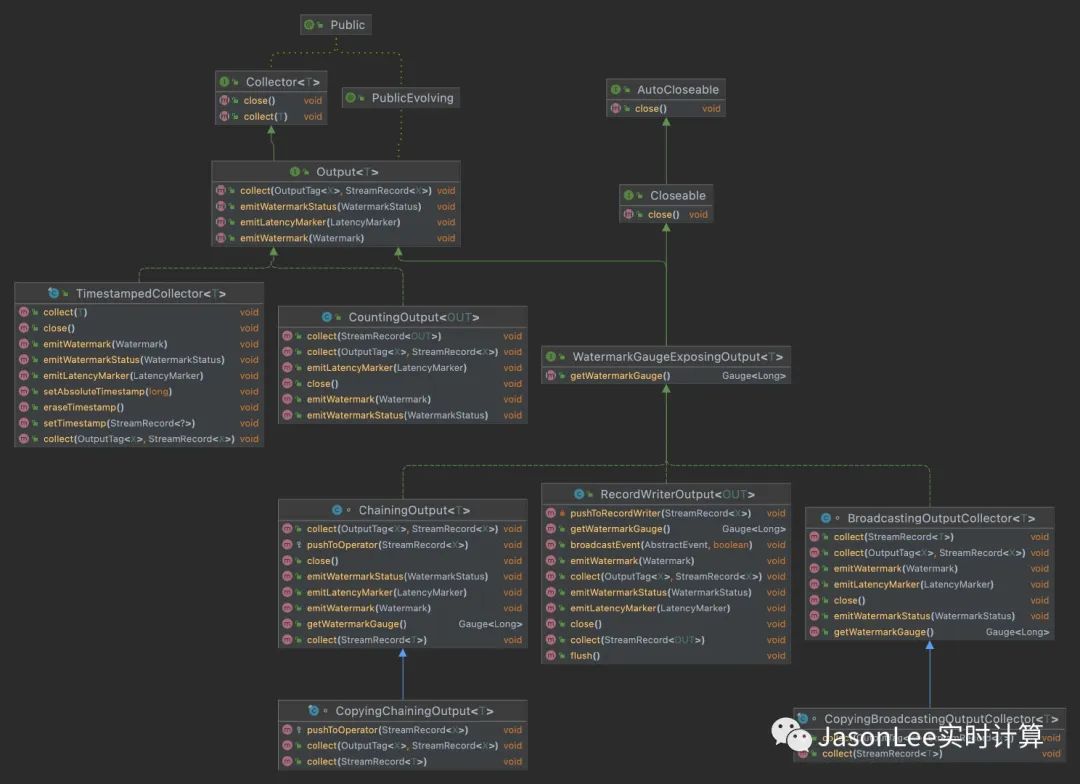
可以看到 TimestampedCollector 和 CountingOutput 是直接实现了 Output 接口的,ChainingOutput,RecordWriterOutput,BroadcastingOutputCollector 这三个类是实现了 WatermarkGaugeExposingOutput 接口,主要是为了显示当前输出的 Watermark 值,WatermarkGaugeExposingOutput 又继承了 Output 接口。
根据其使用场景的不同,我们可以把这些 Output 分成五大类:
「同 operatorChain」
ChainingOutput
CopyingChainingOutput
「跨 operatorChain」
RecordWriterOutput
「统计 Metrics」
CountingOutput
「广播」
BroadcastingOutputCollector
CopyingBroadcastingOutputCollector
「时间戳」
TimestampedCollector
OperatorChain 图
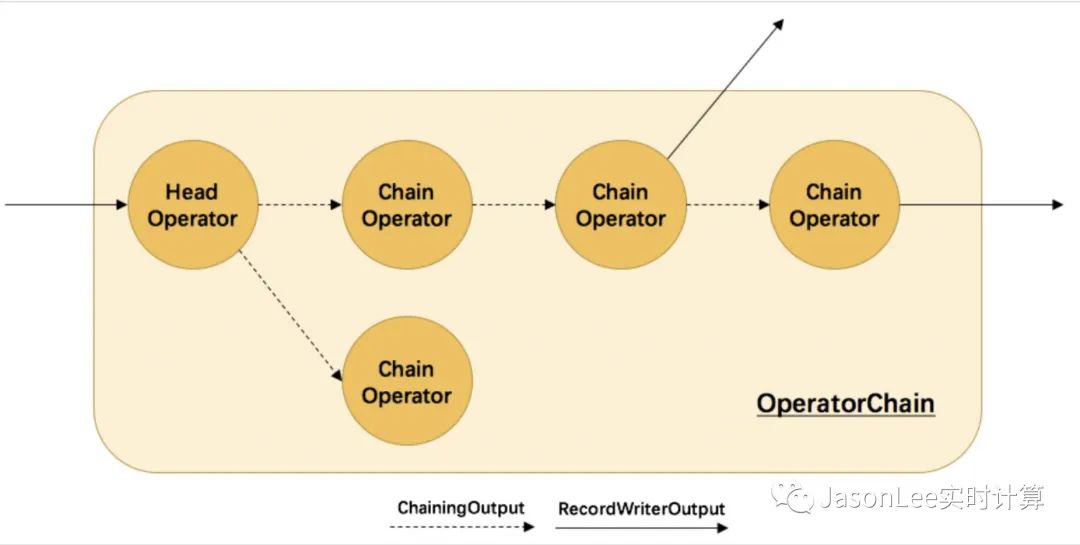
这是一张 OperatorChain 和 Output 的关系图,其中虚线代表的是同一个 operatorChain 之间的数据传递,使用的是 ChainingOutput,实线代表的是跨 operatorChain 之间数据传递,使用的是 RecordWriterOutput。
为了更好的展示每一个 Output 的使用场景,以及把整个数据传递流程串联起来,下面来看一个简单的 Demo。
Demo
上图中的 Kafka Source 和 Map 算子 chain 在一起形成了一个 operatorChain,其中 Kafka Source 又叫做 Head Operator,Map 算子又叫做 Chain Operator,后面的 Process,两个 Print 算子 chain 在一起形成了另外一个 operatorChain,其中 Process 算子又叫做 Head Operator,Print 算子又叫做 Chain Operator。
那 Kafka Source -> Map 之间的数据传递用的则是 ChainingOutput,对应着上图中的虚线部分,Map -> Process 之间的数据传递使用的是 RecordWriterOutput,对应着上图中的实线部分。
另外从上面的分类可以看出来,很多 Output 都有一个对应的 CopyingXXXOutput,比如同一个 operatorChain 内数据传递是有 ChainingOutput 和 CopyingChainingOutput 两个实现类的,那这两者之间又有什么区别和联系呢?我们接着往下面看。
同一个 operatorChain 之间(KafkaSource -> Map)
AsyncDataOutputToOutput#emitRecord
@Override
public void emitRecord(StreamRecord<T> streamRecord)
// 更新 metric
numRecordsOut.inc();
metricGroup.recordEmitted(streamRecord.getTimestamp());
// 这里是 CopyingChainingOutput
output.collect(streamRecord);
在 Kafka Source Operator 中发送数据的对象是 AsyncDataOutputToOutput,它会持有一个 Output ,这里的 Output 实际上是 CopyingChainingOutput 而不是 ChainingOutput,通过调用 collect 发送数据。
CopyingChainingOutput#collect
@Override
public void collect(StreamRecord<T> record)
// 如果是正常的流 outputTag 是空的所以会直接走下面的逻辑
if (this.outputTag != null)
// we are not responsible for emitting to the main output.
return;
pushToOperator(record);
正常的流是没有 outputTag 的(只有侧流输出才有)所以会直接走 pushToOperator 方法。
CopyingChainingOutput#pushToOperator
@Override
protected <X> void pushToOperator(StreamRecord<X> record)
try
// we know that the given outputTag matches our OutputTag so the record
// must be of the type that our operator (and Serializer) expects.
@SuppressWarnings("unchecked")
// 浅拷贝
StreamRecord<T> castRecord = (StreamRecord<T>) record;
// 更新 metric
numRecordsIn.inc();
// 对 record 做深拷贝
StreamRecord<T> copy = castRecord.copy(serializer.copy(castRecord.getValue()));
input.setKeyContextElement(copy);
// 调用下游的 processElement 方法
input.processElement(copy);
catch (ClassCastException e)
if (outputTag != null)
// Enrich error message
ClassCastException replace =
new ClassCastException(
String.format(
"%s. Failed to push OutputTag with id '%s' to operator. "
+ "This can occur when multiple OutputTags with different types "
+ "but identical names are being used.",
e.getMessage(), outputTag.getId()));
throw new ExceptionInChainedOperatorException(replace);
else
throw new ExceptionInChainedOperatorException(e);
catch (Exception e)
throw new ExceptionInChainedOperatorException(e);
pushToOperator 方法的逻辑很简单:
对 record 浅拷贝。
更新 metrics。
对 record 做深拷贝。
设置 Key 的上下文。
调用 chain operator 的 processElement 方法处理数据。
可以看到在深拷贝的时候是需要对数据进行序列化的,这跟我们广义上理解的 Flink 在 JobGraph 阶段主要优化了 operatorChain,从而减少数据在网络传输中序列化和反序列的开销是不太一致的,难道这句话是错的吗?
那我们就来看下 ChainingOutput 的 pushToOperator 方法和 CopyingChainingOutput 的 pushToOperator 有什么区别呢?
ChainingOutput#pushToOperator
protected <X> void pushToOperator(StreamRecord<X> record)
try
// we know that the given outputTag matches our OutputTag so the record
// must be of the type that our operator expects.
@SuppressWarnings("unchecked")
// 浅拷贝
StreamRecord<T> castRecord = (StreamRecord<T>) record;
// 更新 metric
numRecordsIn.inc();
// 设置 key 上下文
input.setKeyContextElement(castRecord);
// 调用下一个算子处理数据
input.processElement(castRecord);
catch (Exception e)
throw new ExceptionInChainedOperatorException(e);
你会发现 ChainingOutput 的 pushToOperator 方法和 CopyingChainingOutput 的几乎一致,唯一的区别就是这里没有对 record 做深拷贝,仅做了一个浅拷贝,显然,这种浅拷贝的方式性能是更高的,那是由什么决定使用 ChainingOutput 还是 CopyingChainingOutput 呢?其实是通过 env.getConfig().enableObjectReuse() 这个配置决定的,默认情况下 objectReuse 是 false 也就是会使用 CopyingChainingOutput 如果开启了 objectReuse 则会使用 ChainingOutput,也就是说如果不开启 objectReuse 是不能完全发挥 operatorChain 优化效果的。
那既然 ChainingOutput 的性能更高,为什么默认不使用 ChainingOutput 呢?因为在某些场景下,开启 objectReuse 可能会带来安全性问题,所以就选择了 CopyingChainingOutput 作为默认的 Output。
当前 operator 的输出是下一个 operator 的输入,所以这里的 input 是 StreamMap 对象,也就相当于是直接调用 StreamMap.processElement 方法来传输数据。
StreamMap#processElement
@Override
public void processElement(StreamRecord<IN> element) throws Exception
// 先调用我们自己的 map 逻辑
output.collect(element.replace(userFunction.map(element.getValue())));
在 processElement 方法里面会先调用 userFunction 的 map 方法,这里的 userFunction 其实就是我们自定义的 map 算子的代码逻辑,然后把返回的结果通过 collect 方法发送到下游算子,在发送之前需要先更新相关的 Metric,所以这里的 output 其实是 CountingOutput 。
CountingOutput#collect
@Override
public void collect(StreamRecord<OUT> record)
// 更新 metric
numRecordsOut.inc();
// 发送数据
output.collect(record);
CountingOutput 对象主要的作用是更新 Metric,然后再发送数据,因为 Map 是 operatorChain 的最后一个 operator,所以它持有的 Output 是 RecordWriterOutput 对象,也就是上图所说的实线传输数据。
不同 operatorChain 之间(Map -> Process)
RecordWriterOutput#collect
@Override
public void collect(StreamRecord<OUT> record)
if (this.outputTag != null)
// we are not responsible for emitting to the main output.
return;
pushToRecordWriter(record);
在 RecordWriterOutput 的 collect 方法里又调用了 pushToRecordWriter 方法。
RecordWriterOutput#pushToRecordWriter
private <X> void pushToRecordWriter(StreamRecord<X> record)
serializationDelegate.setInstance(record);
try
recordWriter.emit(serializationDelegate);
catch (IOException e)
throw new UncheckedIOException(e.getMessage(), e);
通过 recordWriter 的 emit 方法发送数据,因为是跨 operatorChain 的数据传输,并不像 operatorChain 之间数据传输那么简单,直接调用 Chain Operator 的 processElement 处理数据,而是上游先把数据写到 ResultPartition 里,然后下游算子通过 InputChannel 消费数据,这个过程就不在展开了,因为不是我们今天讨论的重点。
OneInputStreamTask#StreamTaskNetworkOutput#emitRecord
@Override
public void emitRecord(StreamRecord<IN> record) throws Exception
// 更新 metric
numRecordsIn.inc();
operator.setKeyContextElement(record);
operator.processElement(record);
下游消费到数据后通过 StreamTaskNetworkOutput 的 emitRecord 方法来发送数据,首先还是更新 Metrics,同样的道理,这里的 operator 表示的是下游算子 ProcessOperator,先要设置上下文 key,最后调用其 processElement 方法传递数据。
同一个 operatorChain 之间(Process -> Print)
从这里开始,其实还是同一个 operatorChain 内的数据传递,整体上的逻辑和上面同一个 operatorChain 之间数据传递的逻辑是一样的,所以下面有些地方就一笔带过了。
ProcessOperator#processElement
@Override
public void processElement(StreamRecord<IN> element) throws Exception
// 设置时间戳
collector.setTimestamp(element);
// 把 element 赋值给 context.element
context.element = element;
// 调用用户的代码逻辑
userFunction.processElement(element.getValue(), context, collector);
// 把 context.element 赋值为空
context.element = null;
在 processElement 方法里主要做了下面几件事情:
给 StreamRecord 对象设置时间戳属性。
把 element 赋值给 context.element。
执行用户自定义的 ProcessFunction 的 processElement 方法。
把 context.element 设置为空。
其中步骤 3 是最重要的,所以我们再来看下在 3 里面是如何传递数据的?
ProcessFunction#processElement
new ProcessFunction<JasonLeePOJO, JasonLeePOJO>()
@Override
public void processElement(
JasonLeePOJO value,
ProcessFunction<JasonLeePOJO, JasonLeePOJO>.Context ctx,
Collector<JasonLeePOJO> out)
throws Exception
if (value.getName().equals("flink"))
// 正常流
out.collect(value);
else if (value.getName().equals("spark"))
// 侧流
ctx.output(test, value);
)
ProcessFunction 是我们自定义的代码逻辑,主要实现了 processElement 方法,在这里会有两种不同的 Output,一种是正常的流输出,一种是侧流输出,正常的流输出用的是 TimestampedCollector,侧流输出用的是 ContextImpl 对象,它实现了 Context 抽象类的 output 方法。
TimestampedCollector#collect
@Override
public void collect(T record)
output.collect(reuse.replace(record));
在 TimestampedCollector 的 collect 方法里没做任何处理,直接调用 CountingOutput 的 collect 方法传递数据。
ContextImpl#output
@Override
public <X> void output(OutputTag<X> outputTag, X value)
if (outputTag == null)
throw new IllegalArgumentException("OutputTag must not be null.");
output.collect(outputTag, new StreamRecord<>(value, element.getTimestamp()));
侧流和正常流稍微不同的是它并没有实现 Output 接口,而是实现了 Context 对象,但是 output 方法里的 output 同样也是 CountingOutput。
CountingOutput#collect
@Override
public void collect(StreamRecord<OUT> record)
numRecordsOut.inc();
output.collect(record);
CountingOutput 的 collect 里先是更新 Metrics,因为需要像下游广播数据,所以这里的 output 是 BroadcastingOutputCollector。
BroadcastingOutputCollector#collect
@Override
public void collect(StreamRecord<T> record)
for (Output<StreamRecord<T>> output : outputs)
output.collect(record);
因为这是在同一个 operatorChain 内传递数据,所以这里的 output 是 CopyingChainingOutput。与 BroadcastingOutputCollector 对应的还有一个 CopyingBroadcastingOutputCollector,这里也顺便看一下。
CopyingBroadcastingOutputCollector#collect
@Override
public <X> void collect(OutputTag<X> outputTag, StreamRecord<X> record)
for (int i = 0; i < outputs.length - 1; i++)
Output<StreamRecord<T>> output = outputs[i];
StreamRecord<X> shallowCopy = record.copy(record.getValue());
output.collect(outputTag, shallowCopy);
if (outputs.length > 0)
// don't copy for the last output
outputs[outputs.length - 1].collect(outputTag, record);
CopyingBroadcastingOutputCollector 是 BroadcastingOutputCollector 的特殊版本,在 collect 方法里面多了一个浅拷贝的逻辑,如果开启了 objectReuse 则使用 CopyingBroadcastingOutputCollector 否则使用 BroadcastingOutputCollector。
CopyingChainingOutput#collect
@Override
public void collect(StreamRecord<T> record)
if (this.outputTag != null)
// we are not responsible for emitting to the main output.
return;
pushToOperator(record);
这里逻辑和上面一样,就跳过了。
CopyingChainingOutput#pushToOperator
@Override
protected <X> void pushToOperator(StreamRecord<X> record)
try
// we know that the given outputTag matches our OutputTag so the record
// must be of the type that our operator (and Serializer) expects.
@SuppressWarnings("unchecked")
StreamRecord<T> castRecord = (StreamRecord<T>) record;
numRecordsIn.inc();
StreamRecord<T> copy = castRecord.copy(serializer.copy(castRecord.getValue()));
input.setKeyContextElement(copy);
input.processElement(copy);
catch (ClassCastException e)
if (outputTag != null)
// Enrich error message
ClassCastException replace =
new ClassCastException(
String.format(
"%s. Failed to push OutputTag with id '%s' to operator. "
+ "This can occur when multiple OutputTags with different types "
+ "but identical names are being used.",
e.getMessage(), outputTag.getId()));
throw new ExceptionInChainedOperatorException(replace);
else
throw new ExceptionInChainedOperatorException(e);
catch (Exception e)
throw new ExceptionInChainedOperatorException(e);
还是和上面一样,直接调用下游 operator 的 processElement 传递数据,这里的下游是 StreamSink,所以 input 是 StreamSink。
StreamSink#processElement
@Override
public void processElement(StreamRecord<IN> element) throws Exception
sinkContext.element = element;
userFunction.invoke(element.getValue(), sinkContext);
在 processElement 方法里会调用 userFunction 的 invoke 方法,但是这里的 userFunction 不是我们自定义实现的,而是 Flink 默认提供的 PrintSinkFunction,到这里整个数据传递流程就结束了。
为了更加方便的对比开启 objectReuse 和不开启 objectReuse 的不同之处,整个调用链路如下:
不开启(默认)objectReuse 的调用链:
AsyncDataOutputToOutput.emitRecord
-->CopyingChainingOutput.collect
-->CopyingChainingOutput.pushToOperator
-->StreamMap.processElement
-->CountingOutput.collect
-->RecordWriterOutput.collect
-->RecordWriterOutput.pushToRecordWriter
-->AbstractStreamTaskNetworkInput.emitNext
-->AbstractStreamTaskNetworkInput.processElement
-->OneInputStreamTask.StreamTaskNetworkOutput.emitRecord
-->ProcessOperator.processElement
-->ProcessFunction.processElement
-->TimestampedCollector.collect (这个是正常流的链路)
-->CountingOutput.collect
-->BroadcastingOutputCollector.collect
-->CopyingChainingOutput.collect
-->CopyingChainingOutput.pushToOperator
-->StreamSink.processElement
-->SinkFunction.invoke
-->PrintSinkFunction.invoke
开启 objectReuse 的调用链:
AsyncDataOutputToOutput.emitRecord
-->ChainingOutput.collect
-->ChainingOutput.pushToOperator
-->StreamMap.processElement
-->CountingOutput.collect
-->RecordWriterOutput.collect
-->RecordWriterOutput.pushToRecordWriter
-->AbstractStreamTaskNetworkInput.emitNext
-->AbstractStreamTaskNetworkInput.processElement
-->OneInputStreamTask.StreamTaskNetworkOutput.emitRecord
-->ProcessFunction.processElement
-->ProcessOperator.ContextImpl.output (这个是侧流输出的链路)
-->CountingOutput.collect
-->CopyingBroadcastingOutputCollector.collect
-->ChainingOutput.collect
-->ChainingOutput.pushToOperator
-->StreamSink.processElement
-->SinkFunction.invoke
-->PrintSinkFunction.invoke
经过对比整个调用链路,你会发现,不开启(默认)objectReuse 的时候,在 operatorChain 之间传递数据用的是 CopyingChainingOutput,在有侧流输出广播的场景下用的是 BroadcastingOutputCollector,开启 objectReuse 的话,在 operatorChain 之间传递数据用的是 ChainingOutput,在有侧流输出广播的场景下用的是 CopyingBroadcastingOutputCollector,其他地方没有差别。
总结
本文从一个简单的 Flink 应用程序出发,介绍了常见的几个 Output 实现类的使用场景及源码解析,ChainingOutput 主要用在 operatorChain 内部传递数据,RecordWriterOutput 主要用在跨 operatorChain 不同 Task 之间传递数据,CountingOutput 主要是为了更新 Metrics,BroadcastingOutputCollector 主要用于广播场景下,TimestampedCollector 主要用来给 StreamRecord 设置时间戳属性。
推荐阅读
❝Flink 1.14.0 全新的 Kafka Connector
Flink 1.14.0 消费 kafka 数据自定义反序列化类
❞
如果你觉得文章对你有帮助,麻烦点一下赞
和在看
吧,你的支持是我创作的最大动力。
以上是关于Flink Collector Output 接口源码解析的主要内容,如果未能解决你的问题,请参考以下文章