机器学习 机器学习 分类算法--K近邻算法 KNN (下)
Posted zhangtaotqy
tags:
篇首语:本文由小常识网(cha138.com)小编为大家整理,主要介绍了机器学习 机器学习 分类算法--K近邻算法 KNN (下)相关的知识,希望对你有一定的参考价值。
六、网格搜索与 K 邻近算法中更多的超参数
七、数据归一化 Feature Scaling
解决方案:将所有的数据映射到同一尺度
八、scikit-learn 中的 Scaler
preprocessing.py
import numpy as np class StandardScaler: def __init__(self): self.mean_ = None self.scale_ = None def fit(self, X): """根据训练数据集X获得数据的均值和方差""" assert X.ndim == 2, "The dimension of X must be 2" self.mean_ = np.array([np.mean(X[:,i]) for i in range(X.shape[1])]) self.scale_ = np.array([np.std(X[:,i]) for i in range(X.shape[1])]) return self def transform(self, X): """将X根据这个StandardScaler进行均值方差归一化处理""" assert X.ndim == 2, "The dimension of X must be 2" assert self.mean_ is not None and self.scale_ is not None, "must fit before transform!" assert X.shape[1] == len(self.mean_), "the feature number of X must be equal to mean_ and std_" resX = np.empty(shape=X.shape, dtype=float) for col in range(X.shape[1]): resX[:,col] = (X[:,col] - self.mean_[col]) / self.scale_[col] return resX
九、更多有关 K 近邻算法的思考
优点:
解决分类问题
天然可以解决多分类问题
思想简单,效果强?大
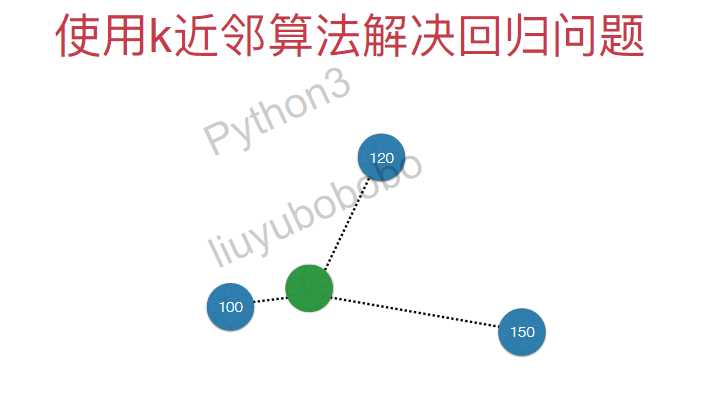
缺点:
?高度数据相关
预测结果不不具有可解释性
维数灾难
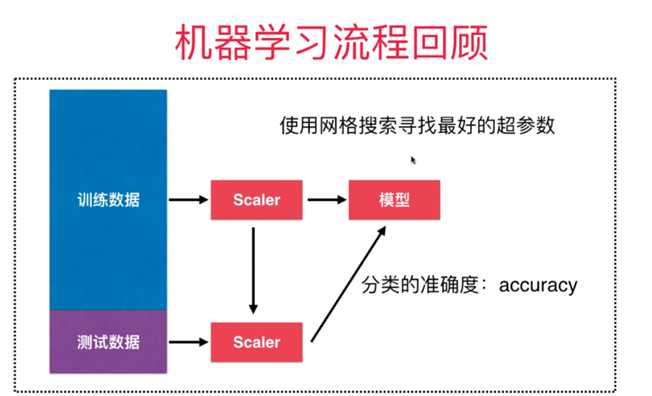
以上是关于机器学习 机器学习 分类算法--K近邻算法 KNN (下)的主要内容,如果未能解决你的问题,请参考以下文章