3D Hand Shape and Pose from Images in the Wild
Posted wjy-lulu
tags:
篇首语:本文由小常识网(cha138.com)小编为大家整理,主要介绍了3D Hand Shape and Pose from Images in the Wild相关的知识,希望对你有一定的参考价值。
目录
3D Hand Shape and Pose from Images in the Wild
一. 论文简介
从单张图像中恢复 2D keypoints + 3D keypoints + mesh + instrinsic(图像坐标系到像素坐标系) + mask,在数据量不充足的情况下进行弱监督。
主要做的贡献如下(可能之前有人已提出):
- Encoder and Regression
- Projection and Mask
- Dataset
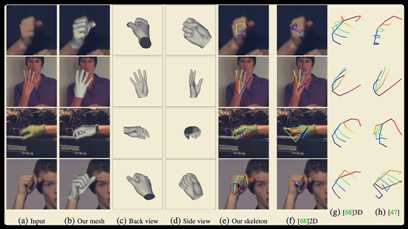
二. 模块详解
2.1 Encoder and Regression
- 这部分都是通用的,2018年左右的论文用的很多,阉割版的End to end recovery of human shape and pose
- 千万注意的一点是
s
是缩放参数,R
旋转参数,t
是平移参数。 - 这是预测的3D是图像坐标系,2D是像素坐标系
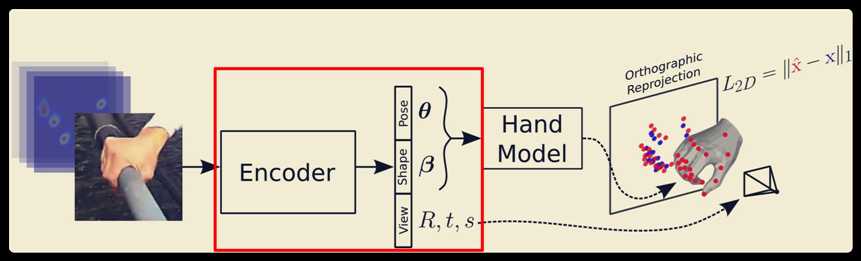
def forward(self, x):
if (self.input_option):
x = self.conv11(x)
else:
x = self.conv1(x[:,0:3])
x = self.bn1(x)
x = self.relu(x)
x = self.maxpool(x)
x = self.layer1(x)
x = self.layer2(x)
x = self.layer3(x)
x = self.layer4(x)
x = self.avgpool(x)
x = x.view(x.size(0), -1)
xs = self.fc(x)
xs = xs + self.mean
scale = xs[:,0] # image space to pixel space
trans = xs[:,1:3]
rot = xs[:,3:6] # 全局旋转(mano的旋视角)
theta = xs[:,6:12] #
beta = xs[:,12:]
x3d = rot_pose_beta_to_mesh(rot,theta,beta)
x = trans.unsqueeze(1) + scale.unsqueeze(1).unsqueeze(2) * x3d[:,:,:2]
x = x.view(x.size(0),-1)
#x3d = scale.unsqueeze(1).unsqueeze(2) * x3d
#x3d[:,:,:2] = trans.unsqueeze(1) + x3d[:,:,:2]
return x, x3d
2.2 Projection and Mask
- 针对数据没有mesh的情况(有mesh直接使用3D数据即可,比mask更准)进行的弱监督
- 没有具体看GrabCut后端,原理就是投影点距离mask的区域,在mask内部为1,外部为0(或者越近越大,越远到0)
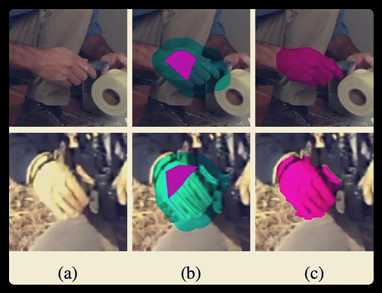
2.3 Dataset
- 文中的pretrained来自于自己构建的数据集,颜色参数来自Meshlab,mesh模型来自MANO,渲染来自Opendr。
- 注意的还是2.1节提到的,建立的是图像坐标系mesh,而不是相机坐标系
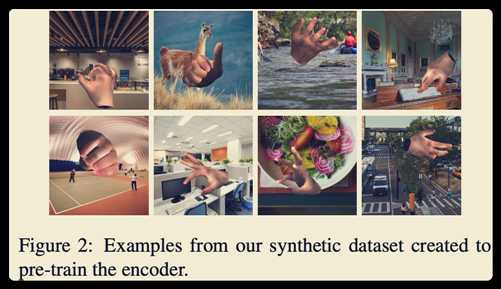
三. 缺点
- 实际中mask无法准确获取,关键点到mask在边缘不准确。
- 直接学习内参有点困难,之前的论文都是iteration,这里没有使用。
以上是关于3D Hand Shape and Pose from Images in the Wild的主要内容,如果未能解决你的问题,请参考以下文章
6D姿态估计从0单排——看论文的小鸡篇——Model Based Training, Detection and Pose Estimation of Texture-Less 3D Objects
手势跟踪论文学习:Realtime and Robust Hand Tracking from DepthCost Function
[Halcon] 算子学习_Calibration_Calibration Object