R语言数据绘图学习(0x01)-安装ggplot2与尝试
Posted medianet-ytc
tags:
篇首语:本文由小常识网(cha138.com)小编为大家整理,主要介绍了R语言数据绘图学习(0x01)-安装ggplot2与尝试相关的知识,希望对你有一定的参考价值。
0x01 安装与R基础
一直听说数据分析里R语言是比较‘正统’,况且久闻ggplot2这些R语言的数据分析库大名,想到今后数据分析和整理的需要,这里开一个简单的系列学习一些R语言和ggplot2的绘图基础。本人学习的书籍是Winston Chang大佬的《R Graphics Cookbook》,且稍有一点Python里的Plotnine绘图基础。但我算是R语言小白,所以整个系列也可以记录自己学习遇到的坑,供大家参考。
库安装
我使用的R版本为3.6.3,需要下载的库包括ggplot2,gcookbook和dplyr库。可以使用如下的安装指令:
install.packages("ggplo2")
install.packages("dplyr")
install.packages("gcookbook")
如果遇到了installation of package ‘ggplot2’ had non-zero exit status类似的问题,可以指定安装程序强制安装二进制文件[1],如
install.packages("ggplo2", type = "binary")
R语言数据处理基础
首先简单介绍数据输入常用的两种方法,一种是CSV数据,我们可以采用函数read.csv(file, args)
输入;另一种常用数据EXCEL数据可以使用read_excel(file)
函数输入数据。
dplyr包(magrittr包)提供了一种运算符%>%,可以在R中实现类似连续函数调用的编程方法,以嵌套函数f,g,h为例:
h(g(f(x)))
# 等价于
x %>%
f() %>%
g() %>%
h()
这样的函数调用相比嵌套函数更加直观,尤其是在多个连续的数据集处理场合。
基础绘图以及概念
散点图和完整流程
绘制图片使用的数据集为mtcars:
mtcars %>% head()
mpg cyl disp hp drat wt qsec vs am gear carb
Mazda RX4 21.0 6 160 110 3.90 2.620 16.46 0 1 4 4
Mazda RX4 Wag 21.0 6 160 110 3.90 2.875 17.02 0 1 4 4
Datsun 710 22.8 4 108 93 3.85 2.320 18.61 1 1 4 1
Hornet 4 Drive 21.4 6 258 110 3.08 3.215 19.44 1 0 3 1
Hornet Sportabout 18.7 8 360 175 3.15 3.440 17.02 0 0 3 2
Valiant 18.1 6 225 105 2.76 3.460 20.22 1 0 3 1
在开始绘图之前,有一点基本的R语言基础设施需要准备。R语言画图需要有基础的画布,可以用dev.new()函数来开启一个新的画布(如果是windows系统需要使用windows()函数)。
dev.new()
# windows() # windows系统使用
下面直接展示最简单的散点图绘制代码:
p <- ggplot(mtcars, aes(x = wt, y = mpg)) +
geom_point()
上面的代码就是一个使用ggplot2绘图的最基本结构,ggplot函数是保存数据集和映射信息(aes)等基本信息的绘图主函数,也可以理解为存储所有信息的画布底层。第一个参数放入数据集,后续的映射都在此数据集上进行,本例中就是使用了mtcars中的wt列作为x,mpg列作为y值。ggplot2中的函数基本均是加法进行组合,所以这里加上了geom_point函数组合进绘图。此时geom_point函数通过ggplot函数可以得知其绘图需要用到的键信息(x, y)分别对应的数据值。代码运行结果如下:

绘制完图后,可以选择print(p)来查看图片并保存,或者使用ggplot2自带的函数ggsave来保存图片,基本的格式例如png,pdf等都有[2]。
ggsave(p, "p.png")
如果想要绘图的数据不属于同一个data frame,那么可以不指定ggplot函数的数据项,在aes映射中直接定义,如下所示:
ggplot(data=null, aes(x = mtcars$wt, y = mtcars$mpg))
其他基本绘图与基础
下面的代码展示了R语言绘制线形图的基本函数geom_line,同时展示了ggplot2通过组合函数geom_line和geom_point来绘图的基本逻辑:
p <- ggplot(pressure, aes(x = temperature, y = pressure)) +
geom_line() +
geom_point()
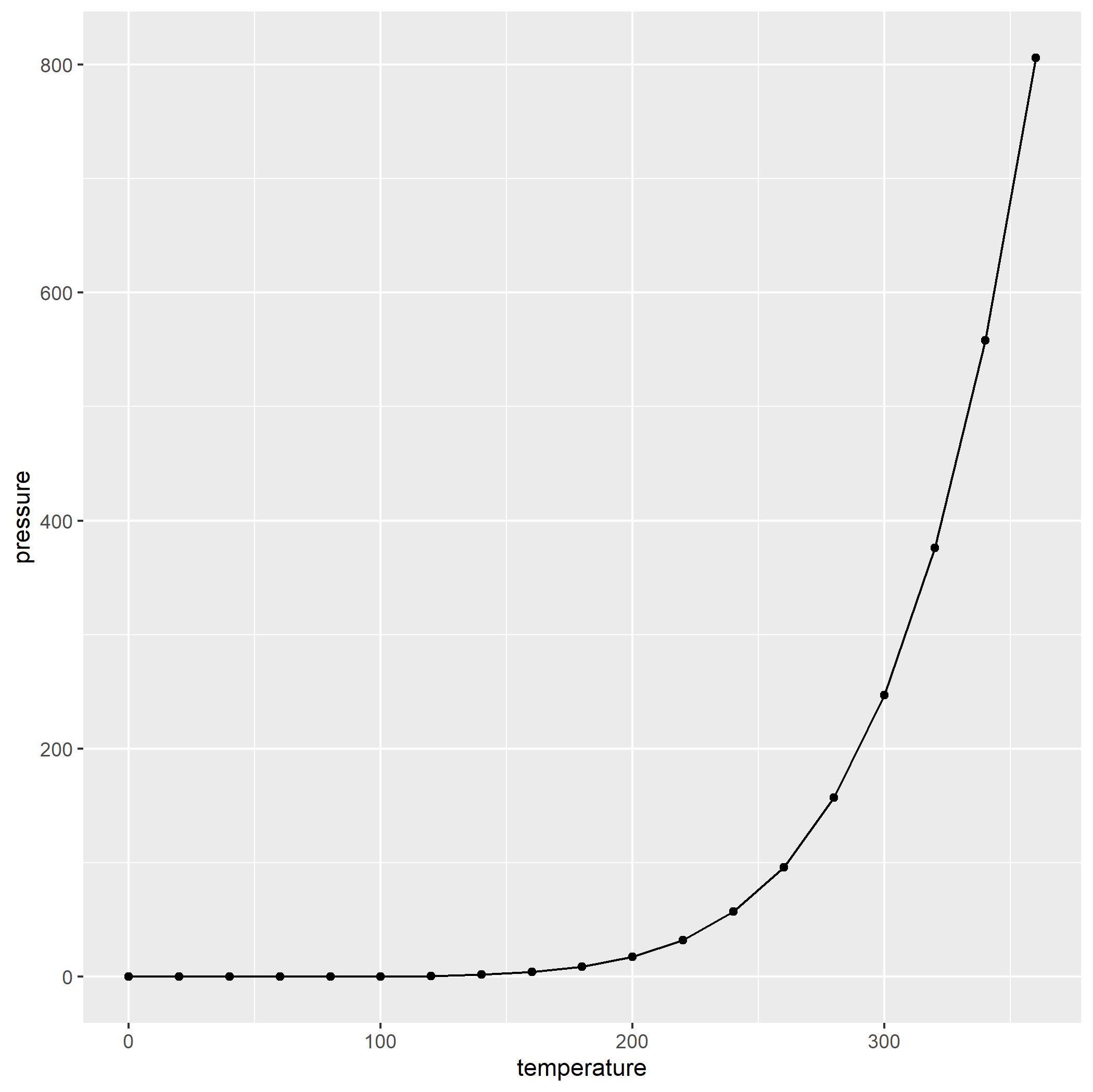
对于柱形图,ggplot2的相关支持比较多,这里展示最基础的两种,一个是geom_bar函数:
p <- ggplot(mtcars, aes(x = factor(cyl))) +
geom_bar()
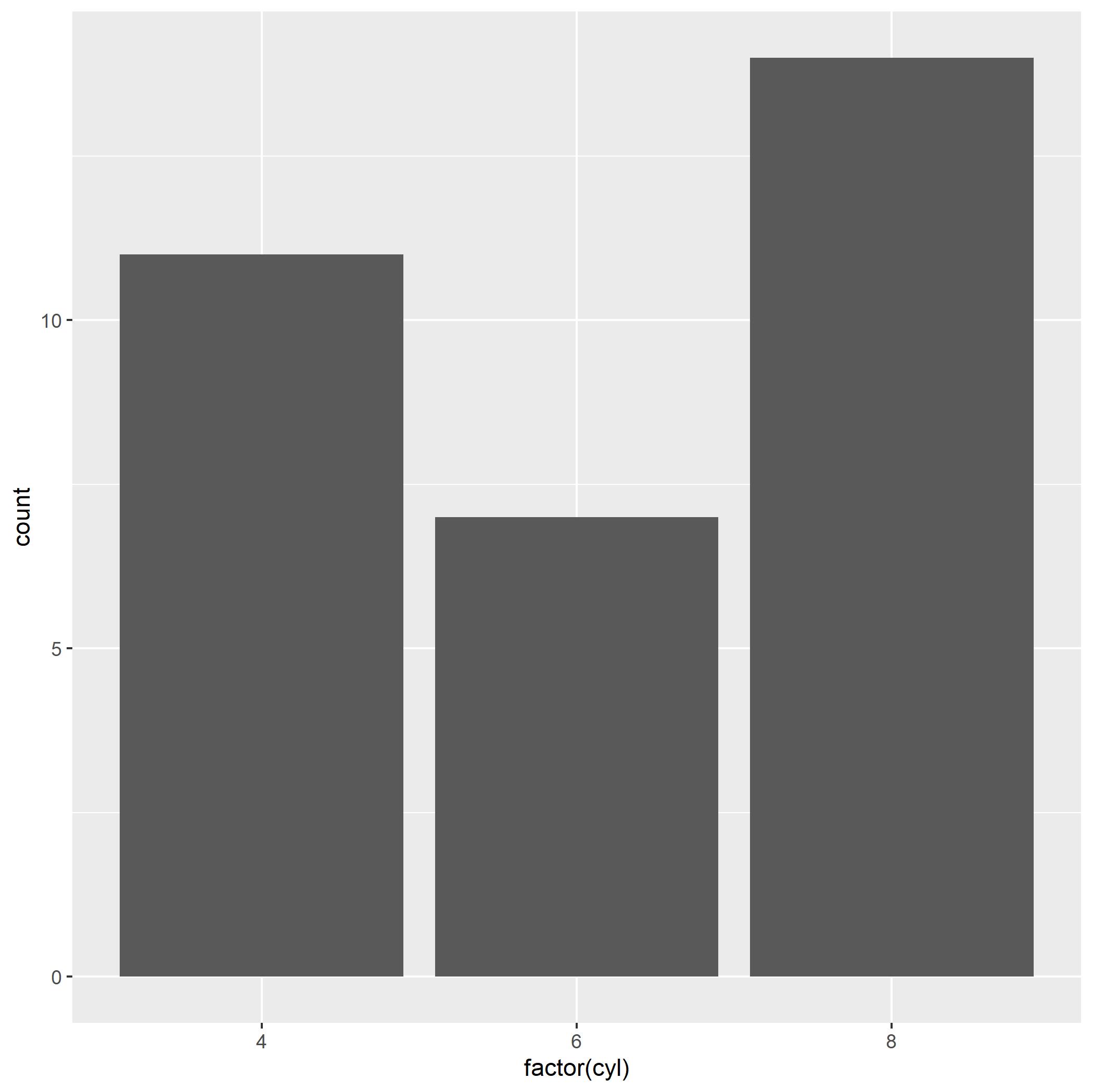
这里有一个新的函数factor,这个函数是将数据列变化为因子,体现在图上类似于将连续变量转化为了离散的变量。可以看到,上述的映射信息中不包括y值。这是因为geom_bar函数默认的运作方式count,也就是统计x的出现次数并绘图,相当于geom_bar(stat="count"),其中stat选项是统计函数的意思。如果需要绘制具体数值类似y值的柱形图就需要用到下一个函数了。
第二个柱形图的函数是geom_col函数:
p <- ggplot(BOD, aes(x = factor(Time), y = demand)) +
geom_col()
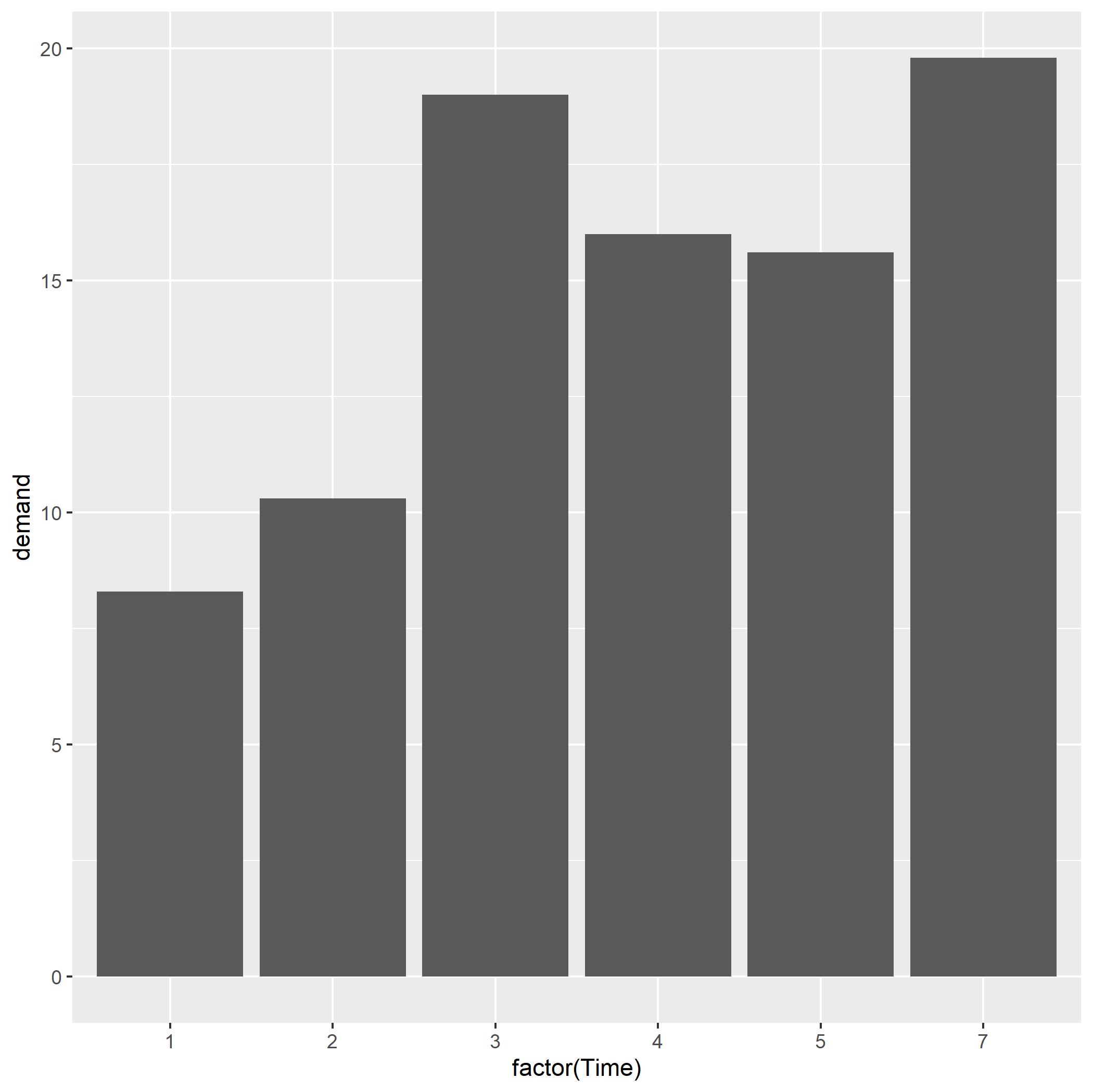
其实geom_col的效果与geom_bar(stat="identity")是一致的,这样就能随心所欲的绘制柱形图了。
下面的代码示例展示了绘制和柱形图很像的直方图的案例:
p <- ggplot(mtcars, aes(x = mpg)) +
geom_histogram(binwidth = 3)
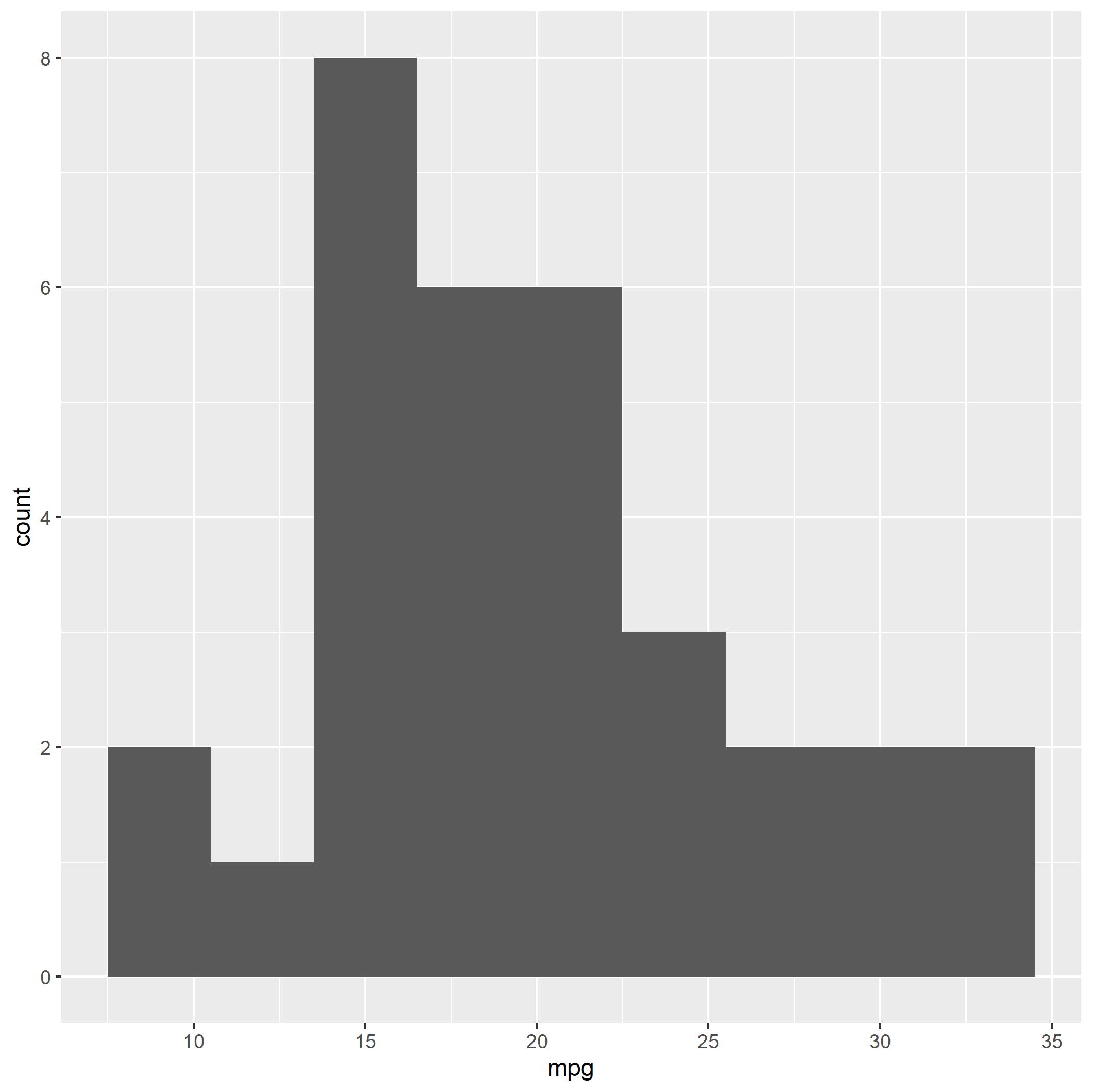
下面的代码展示了如何绘制箱型图的例子:
p <- ggplot(ToothGrowth, aes(x = interaction(supp, dose), y = len)) +
geom_boxplot()

除开这些基本绘图,数学函数的绘制也比较容易:
# 自定义函数
myfun <- function(xvar)
1 / (1 + exp(-xvar + 10))
p <- ggplot(data.frame(xdata = c(0, 20)), aes(x = xdata)) +
stat_function(fun = myfun, geom = "line")

总结
本节主旨在于R语言ggplot2绘图的初探,熟悉基本的绘图模式。后续将会一一展开学习库中的绘图细节,完善绘图质量。
参考资料:
吴裕雄--天生自然 R语言开发学习:使用ggplot2进行高级绘图
#----------------------------------------------------------# # R in Action (2nd ed): Chapter 19 # # Advanced graphics with ggplot2 # # requires packages ggplot2, RColorBrewer, gridExtra, # # and car (for datasets) # # install.packages(c("ggplot2", "gridExtra", # # "RColorBrewer", "car")) # #----------------------------------------------------------# par(ask=TRUE) # Basic scatterplot library(ggplot2) ggplot(data=mtcars, aes(x=wt, y=mpg)) + geom_point() + labs(title="Automobile Data", x="Weight", y="Miles Per Gallon") # Scatter plot with additional options library(ggplot2) ggplot(data=mtcars, aes(x=wt, y=mpg)) + geom_point(pch=17, color="blue", size=2) + geom_smooth(method="lm", color="red", linetype=2) + labs(title="Automobile Data", x="Weight", y="Miles Per Gallon") # Scatter plot with faceting and grouping data(mtcars) mtcars$am <- factor(mtcars$am, levels=c(0,1), labels=c("Automatic", "Manual")) mtcars$vs <- factor(mtcars$vs, levels=c(0,1), labels=c("V-Engine", "Straight Engine")) mtcars$cyl <- factor(mtcars$cyl) library(ggplot2) ggplot(data=mtcars, aes(x=hp, y=mpg, shape=cyl, color=cyl)) + geom_point(size=3) + facet_grid(am~vs) + labs(title="Automobile Data by Engine Type", x="Horsepower", y="Miles Per Gallon") # Using geoms data(singer, package="lattice") ggplot(singer, aes(x=height)) + geom_histogram() ggplot(singer, aes(x=voice.part, y=height)) + geom_boxplot() data(Salaries, package="car") library(ggplot2) ggplot(Salaries, aes(x=rank, y=salary)) + geom_boxplot(fill="cornflowerblue", color="black", notch=TRUE)+ geom_point(position="jitter", color="blue", alpha=.5)+ geom_rug(side="l", color="black") # Grouping library(ggplot2) data(singer, package="lattice") ggplot(singer, aes(x=voice.part, y=height)) + geom_violin(fill="lightblue") + geom_boxplot(fill="lightgreen", width=.2) data(Salaries, package="car") library(ggplot2) ggplot(data=Salaries, aes(x=salary, fill=rank)) + geom_density(alpha=.3) ggplot(Salaries, aes(x=yrs.since.phd, y=salary, color=rank, shape=sex)) + geom_point() ggplot(Salaries, aes(x=rank, fill=sex)) + geom_bar(position="stack") + labs(title=‘position="stack"‘) ggplot(Salaries, aes(x=rank, fill=sex)) + geom_bar(position="dodge") + labs(title=‘position="dodge"‘) ggplot(Salaries, aes(x=rank, fill=sex)) + geom_bar(position="fill") + labs(title=‘position="fill"‘) # Placing options ggplot(Salaries, aes(x=rank, fill=sex))+ geom_bar() ggplot(Salaries, aes(x=rank)) + geom_bar(fill="red") ggplot(Salaries, aes(x=rank, fill="red")) + geom_bar() # Faceting data(singer, package="lattice") library(ggplot2) ggplot(data=singer, aes(x=height)) + geom_histogram() + facet_wrap(~voice.part, nrow=4) library(ggplot2) ggplot(Salaries, aes(x=yrs.since.phd, y=salary, color=rank, shape=rank)) + geom_point() + facet_grid(.~sex) data(singer, package="lattice") library(ggplot2) ggplot(data=singer, aes(x=height, fill=voice.part)) + geom_density() + facet_grid(voice.part~.) # Adding smoothed lines data(Salaries, package="car") library(ggplot2) ggplot(data=Salaries, aes(x=yrs.since.phd, y=salary)) + geom_smooth() + geom_point() ggplot(data=Salaries, aes(x=yrs.since.phd, y=salary, linetype=sex, shape=sex, color=sex)) + geom_smooth(method=lm, formula=y~poly(x,2), se=FALSE, size=1) + geom_point(size=2) # Modifying axes data(Salaries,package="car") library(ggplot2) ggplot(data=Salaries, aes(x=rank, y=salary, fill=sex)) + geom_boxplot() + scale_x_discrete(breaks=c("AsstProf", "AssocProf", "Prof"), labels=c("Assistant\\nProfessor", "Associate\\nProfessor", "Full\\nProfessor")) + scale_y_continuous(breaks=c(50000, 100000, 150000, 200000), labels=c("$50K", "$100K", "$150K", "$200K")) + labs(title="Faculty Salary by Rank and Sex", x="", y="") # Legends data(Salaries,package="car") library(ggplot2) ggplot(data=Salaries, aes(x=rank, y=salary, fill=sex)) + geom_boxplot() + scale_x_discrete(breaks=c("AsstProf", "AssocProf", "Prof"), labels=c("Assistant\\nProfessor", "Associate\\nProfessor", "Full\\nProfessor")) + scale_y_continuous(breaks=c(50000, 100000, 150000, 200000), labels=c("$50K", "$100K", "$150K", "$200K")) + labs(title="Faculty Salary by Rank and Gender", x="", y="", fill="Gender") + theme(legend.position=c(.1,.8)) # Scales ggplot(mtcars, aes(x=wt, y=mpg, size=disp)) + geom_point(shape=21, color="black", fill="cornsilk") + labs(x="Weight", y="Miles Per Gallon", title="Bubble Chart", size="Engine\\nDisplacement") data(Salaries, package="car") ggplot(data=Salaries, aes(x=yrs.since.phd, y=salary, color=rank)) + scale_color_manual(values=c("orange", "olivedrab", "navy")) + geom_point(size=2) ggplot(data=Salaries, aes(x=yrs.since.phd, y=salary, color=rank)) + scale_color_brewer(palette="Set1") + geom_point(size=2) library(RColorBrewer) display.brewer.all() # Themes data(Salaries, package="car") library(ggplot2) mytheme <- theme(plot.title=element_text(face="bold.italic", size="14", color="brown"), axis.title=element_text(face="bold.italic", size=10, color="brown"), axis.text=element_text(face="bold", size=9, color="darkblue"), panel.background=element_rect(fill="white", color="darkblue"), panel.grid.major.y=element_line(color="grey", linetype=1), panel.grid.minor.y=element_line(color="grey", linetype=2), panel.grid.minor.x=element_blank(), legend.position="top") ggplot(Salaries, aes(x=rank, y=salary, fill=sex)) + geom_boxplot() + labs(title="Salary by Rank and Sex", x="Rank", y="Salary") + mytheme # Multiple graphs per page data(Salaries, package="car") library(ggplot2) p1 <- ggplot(data=Salaries, aes(x=rank)) + geom_bar() p2 <- ggplot(data=Salaries, aes(x=sex)) + geom_bar() p3 <- ggplot(data=Salaries, aes(x=yrs.since.phd, y=salary)) + geom_point() library(gridExtra) grid.arrange(p1, p2, p3, ncol=3) # Saving graphs ggplot(data=mtcars, aes(x=mpg)) + geom_histogram() ggsave(file="mygraph.pdf")
以上是关于R语言数据绘图学习(0x01)-安装ggplot2与尝试的主要内容,如果未能解决你的问题,请参考以下文章
吴裕雄--天生自然 R语言开发学习:使用ggplot2进行高级绘图