Elasticsearch专题精讲——What's new in 8.7?
Posted 左扬(你们的胃叫胃,孤的叫胃PLUS)
tags:
篇首语:本文由小常识网(cha138.com)小编为大家整理,主要介绍了Elasticsearch专题精讲——What's new in 8.7?相关的知识,希望对你有一定的参考价值。
What\'s new in 8.7?
https://www.elastic.co/guide/en/elasticsearch/reference/8.7/release-highlights.html , orther versions:8.6 | 8.5 | 8.4 | 8.3 | 8.2 | 8.1 | 8.0
Time series (TSDS) GA (时间序列)
Time Series Data Stream (TSDS) is a feature for optimizing Elasticsearch indices for time series data. This involves sorting the indices to achieve better compression and using synthetic _source to reduce index size. As a result, TSDS indices are significantly smaller than non-time_series indices that contain the same data. TSDS is particularly useful for managing time series data with high volume.
时间序列数据流(TSDS)是用于优化时间序列数据的 Elasticsearch 索引的一个特性。这涉及到对索引进行排序以实现更好的压缩,并使用综合 _source 来减少索引大小。因此, TSDS 指数明显小于包含相同数据的非时间序列指数。TSDS 对于管理大容量的时间序列数据特别有用。
Downsampling GA (降采样GA)
Downsampling is a feature that reduces the number of stored documents in Elasticsearch time series indices, resulting in smaller indices and improved query latency. This optimization is achieved by pre-aggregating time series indices, using the time_series index schema to identify the time series. Downsampling is configured as an action in ILM, making it a useful tool for managing large volumes of time series data in Elasticsearch.
降采样 (Downsampling) 是 Elasticsearch 中的一项功能,它可以减少时间序列索引中存储的文档数量,从而降低索引大小并提高查询响应速度。通过使用时间序列索引架构识别时间序列并进行预聚合,实现这种优化。降采样是在 ILM 中配置的一个操作,可用于管理 Elasticsearch 中的大量时间序列数据,是一个非常有用的工具。 通过预先聚合数据,降采样减少了查询时需要进行的计算量,从而提高了查询响应速度。此外,由于降采样过程减少了索引文档的数量,可以减少索引存储空间的要求,对于大规模部署来说非常重要。 总的来说,降采样是 Elasticsearch 中的一个强大功能,可帮助用户优化它们的时间序列数据存储和分析能力,并提高其整体查询性能。
Geohex aggregations on both geo_point and geo_shape fields (Geohex聚合可适用于包括geo_point和geo_shape字段在内的数据类型)
Previously Elasticsearch 8.1.0 expanded geo_grid aggregation support from rectangular tiles (geotile and geohash) to include hexagonal tiles, but for geo_point only. Now Elasticsearch 8.7.0 will support Geohex aggregations over geo_shape as well, which completes the long desired need to perform hexagonal aggregations on spatial data
之前, Elasticsearch 8.1.0 将 geo_grid 聚合的支持从 rectangular(矩形) tiles(瓦片) (geotile和geohash) 扩展到包括 hexagonal(六边形) tiles(瓦片), 但仅适用于 geo_point。现在, Elasticsearch 8.7.0 将支持在 geo_shape 上进行 Geohex 聚合, 这满足了长期以来在空间数据上执行 hexagonal(六边形) 聚合的需求。
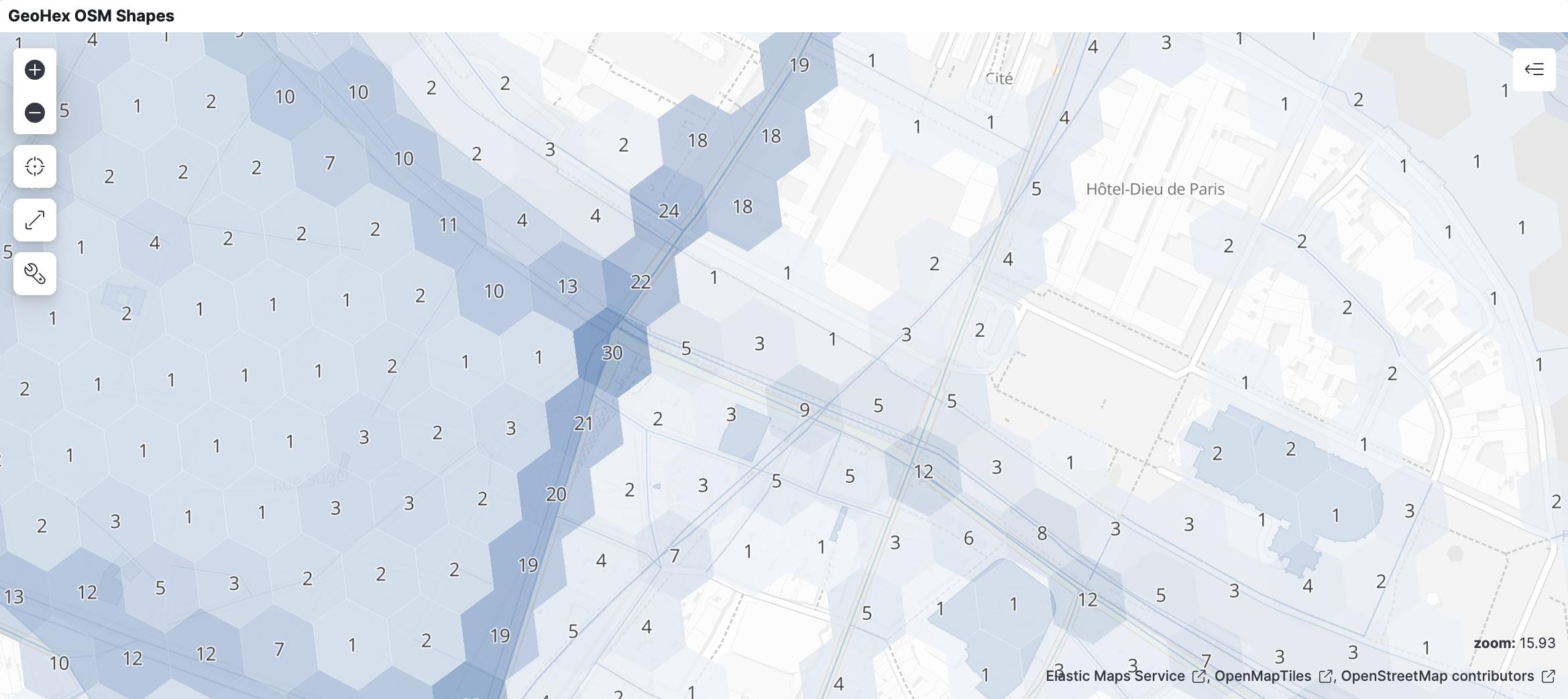
2018年,优步(Uber)宣布开放了自己的 h2库,使地球正六边形镶嵌能够更好地分析自己的流量和区域定价模型。使用六角瓦片进行分析已经变得越来越流行,因为每个瓦片代表地球上非常相似的地理区域,以及瓦片中心之间的距离在所有方向上都非常相似,并且在整个地图上都是一致的。现在所有 Elasticsearch 用户都可以享受这些好处。
Allow more than one KNN search clause允许多个 KNN 搜索子句edit编辑
Some vector search scenarios require relevance ranking using a few kNN clauses, e.g. when ranking based on several fields, each with its own vector, or when a document includes a vector for the image and another vector for the text. The user may want to obtain relevance ranking based on a combination of all of these kNN clauses.
一些向量搜索场景需要使用几个 kNN 子句进行相关性排序,例如,当基于几个字段进行排序时,每个字段都有自己的向量,或者当文档包含图像的向量和文本的另一个向��时。用户可能希望基于所有这些 kNN 子句的组合获得相关性排名。
Make natural language processing GA制作自然语言处理 GAedit编辑
From 8.7, NLP model management, model allocation, and support for inference against third party models are generally available. (The new text_embedding extension to
knn search is still in technical preview.)
从8.7开始,NLP 模型管理、模型分配和支持对第三方模型的推理通常是可用的。(knn 搜索的新文本嵌入扩展仍处于技术预览阶段。)
Speed up ingest geoip processors加速摄取地理位置处理器edit编辑
The geoip
ingest processor is significantly faster.
Geoip 摄取处理器明显更快。
Previous versions of the geoip library needed special permission to execute databinding code, requiring an expensive permissions check and AccessController.doPrivileged call. The current version of the geoip library no longer requires that, however, so the expensive code has been removed, resulting in better performance for the ingest geoip processor.
以前版本的 Geoip 库需要特殊权限来执行数据绑定代码,需要昂贵的权限检查和 AccessController.doPrivileged 调用。然而,当前版本的 Geoip 库不再需要这个功能,因此删除了昂贵的代码,从而为摄取的 Geoip 处理器带来了更好的性能。
Speed up ingest set and append processors加速摄取集和追加处理器edit编辑
The set
and append ingest processors that use mustache templates are significantly faster.
设置和追加使用胡子模板的摄取处理器要快得多。
Improved downsampling performance改进的下采样性能edit编辑
Several improvements were made to the performance of downsampling. All hashmap lookups were removed. Also metrics/label producers were modified so that they extract the doc_values directly from the leaves. This allows for extra optimizations for cases such as labels/counters that do not extract doc_values unless they are consumed. Those changes yielded a 3x-4x performance improvement of the downsampling operation, as measured by our benchmarks.
对下采样的性能作了一些改进。所有的散列表查找都被删除了。还修改了度量/标签生成器,以便它们直��从叶子中提取 doc _ value。这允许对诸如标签/计数器之类的情况进行额外的优化,除非使用了 doc _ value,否则它们不会提取 doc _ value。根据我们的基准测试,这些变化使得下采样操作的性能提高了3-4倍。
The Health API is now generally availableHealthAPI 现在普遍可用edit编辑
Elasticsearch introduces a new Health API designed to report the health of the cluster. The new API provides both a high level overview of the cluster health, and a very detailed report that can include a precise diagnosis and a resolution.
Elasticsearch 引入了一个新的 HealthAPI,旨在报告集群的健康状况。新的 API 既提供了集群健康状况的高级概述,也提供了包括精确诊断和解决方案的非常详细的报告。
Improved performance for get, mget and indexing with explicit `_id`s使用显式的“ _ id”改进了 get、 mget 和索引的性能edit编辑
The false positive rate for the bloom filter on the _id field was reduced from ~10% to ~1%, reducing the I/O load if a term is not present in a segment. This improves performance when retrieving documents by
_id
, which happens when performing get or mget requests, or when issuing _bulk requests that provide explicit `_id`s.
开花滤波器的假阳性率从 ~ 10% 降低到 ~ 1% ,减少了 I/O 负荷,如果一个项目没有出现在一个段。这提高了通过 _ id 检索文档时的性能,这在执行 get 或 mget 请求时发生,或者在发出提供显式‘ _ id’的 _ mass 请求时发生。
Speed up ingest processing with multiple pipelines使用多个管道加速摄取处理edit编辑
Processing documents with both a request/default and a final pipeline is significantly faster.
处理同时具有请求/默认值和最终管道的文档要快得多。
Rather than marshalling a document from and to json once per pipeline, a document is now marshalled from json before any pipelines execute and then back to json after all pipelines have executed.
现在不需要在每个管道之前将文档从 json 封送到 json,而是在执行任何管道之前将文档从 json 封送到 json,然后在执行所有管道之后将文档返回到 json。
Support geo_grid ingest processor支持 geo _ grid 摄取处理器edit编辑
The geo_grid
ingest processor supports creating indexable geometries from geohash, geotile and h2 cells.
Geo _ grid 摄取处理器支持从 Geohash、 Geotiles 和 h2单元创建可索引的几何图形。
There already exists a circle
ingest processor that creates a polygon from a point and radius definition. This concept is useful when there is need to use spatial operations that work with indexable geometries on geometric objects that are not defined spatially (or at least not indexable by lucene). In this case, the string 4/8/5 does not have spatial meaning, until we interpret it as the address of a rectangular
geotile
, and save the bounding box defining its border for further use. Likewise we can interpret geohash strings like
u0
as a tile, and h2 strings like 811fbffffffffff as an hexagonal cell, saving the cell border as a polygon.
已经存在一个圆摄取处理器,它根据点和半径定义创建一个多边形。当需要使用空间操作时,这个概念非常有用,这些空间操作可以对没有空间定义的几何对象(或者至少不能被 Lucene 索引)使用可索引的几何形状。在这种情况下,字符串4/8/5没有空间意义,直到我们将其解释为一个矩形土工织物的地址,并保存定义其边界的边框以供进一步使用。同样,我们可以将 u0这样的地理哈希字符串解释为平铺字符串,将811fbffffffff 这样的 h2字符串解释为六边形单元格,从而将单元格边界保存为多边形。
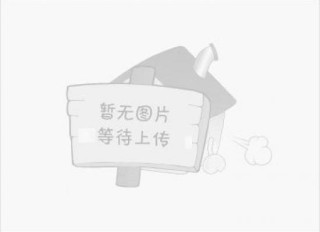
Make 制造 frequent_item_sets aggregation GA 聚合 GA
edit编辑
edit编辑
The frequent_item_sets
aggregation has been moved from technical preview to general availability.
经常项目集聚合已经从技术预览转移到通用可用性。
Release time_series and rate (on counter fields) aggegations as tech preview作为技术预览发布时间序列和速率(在计数器字段上)聚合edit编辑
Make time_series
aggregation and rate
aggregation (on counter fields) available without using the time series feature flag. This change makes these aggregations available as tech preview.
使时间序列聚合和速率聚合(在计数器字段上)可用,而不使用时间序列特性标志。此更改使这些聚合可以作为技术预览。
Currently there is no documentation about the time_series aggregation. This will be added in a followup change.
目前没有关于 time _ Series 聚合的文档,这将在后续更改中添加。
四十四 Python分布式爬虫打造搜索引擎Scrapy精讲—elasticsearch(搜索引擎)的基本查询
1、elasticsearch(搜索引擎)的查询
elasticsearch是功能非常强大的搜索引擎,使用它的目的就是为了快速的查询到需要的数据
查询分类:
基本查询:使用elasticsearch内置的查询条件进行查询
组合查询:把多个查询条件组合在一起进行复合查询
过滤:查询同时,通过filter条件在不影响打分的情况下筛选数据
2、elasticsearch(搜索引擎)创建数据
首先我们先创建索引、表、以及字段属性、字段类型、添加好数据
注意:一般我们中文使用ik_max_word中文分词解析器,所有在需要分词建立倒牌索引的字段都要指定,ik_max_word中文分词解析器
系统默认不是ik_max_word中文分词解析器
ik_max_word中文分词解析器是elasticsearch(搜索引擎)的一个插件,在elasticsearch安装目录的plugins/analysis-ik文件夹里,版本为5.1.1
更多说明:https://github.com/medcl/elasticsearch-analysis-ik
说明:
#创建索引(设置字段类型) #注意:一般我们中文使用ik_max_word中文分词解析器,所有在需要分词建立倒牌索引的字段都要指定,ik_max_word中文分词解析器 #系统默认不是ik_max_word中文分词解析器 PUT jobbole #创建索引设置索引名称 { "mappings": { #设置mappings映射字段类型 "job": { #表名称 "properties": { #设置字段类型 "title":{ #表名称 "store": true, #字段属性true表示保存数据 "type": "text", #text类型,text类型可以分词,建立倒排索引 "analyzer": "ik_max_word" #设置分词解析器,ik_max_word是一个中文分词解析器插件 }, "company_name":{ #字段名称 "store": true, #字段属性true表示保存数据 "type": "keyword" #keyword普通字符串类型,不分词 }, "desc":{ #字段名称 "type": "text" #text类型,text类型可以分词,但是没有设置分词解析器,使用系统默认 }, "comments":{ #字段名称 "type": "integer" #integer数字类型 }, "add_time":{ #字段名称 "type": "date", #date时间类型 "format":"yyyy-MM-dd" #yyyy-MM-dd时间格式化 } } } } }
#保存文档(相当于数据库的写入数据) POST jobbole/job { "title":"python django 开发工程师", #字段名称:值 "company_name":"美团科技有限公司", #字段名称:值 "desc":"对django的概念熟悉, 熟悉python基础知识", #字段名称:值 "comments":20, #字段名称:值 "add_time":"2017-4-1" #字段名称:值 } POST jobbole/job { "title":"python scrapy redis 分布式爬虫基础", "company_name":"玉秀科技有限公司", "desc":"对scrapy的概念熟悉, 熟悉redis基础知识", "comments":5, "add_time":"2017-4-2" } POST jobbole/job { "title":"elasticsearch打造搜索引擎", "company_name":"通讯科技有限公司", "desc":"对elasticsearch的概念熟悉", "comments":10, "add_time":"2017-4-3" } POST jobbole/job { "title":"pyhhon打造推荐引擎系统", "company_name":"智能科技有限公司", "desc":"熟悉推荐引擎系统算法", "comments":60, "add_time":"2017-4-4" }
通过上面可以看到我们创建了索引并且设置好了字段的属性、类型、以及分词解析器,创建了4条数据
3、elasticsearch(搜索引擎)基本查询
match查询【用的最多】
会将我们的搜索词在当前字段设置的分词器进行分词,到当前字段查找,匹配度越高排名靠前,如果搜索词是大写字母会自动转换成小写
#match查询 #会将我们的搜索词进行分词,到指定字段查找,匹配度越高排名靠前 GET jobbole/job/_search { "query": { "match": { "title": "搜索引擎" } } }
term查询
不会将我们的搜索词进行分词,将搜索词完全匹配的查询
#term查询 #不会将我们的搜索词进行分词,将搜索词完全匹配的查询 GET jobbole/job/_search { "query": { "term": { "title":"搜索引擎" } } }
terms查询
传递一个数组,将数组里的词分别匹配
#terms查询 #传递一个数组,将数组里的词分别匹配 GET jobbole/job/_search { "query": { "terms": { "title":["工程师","django","系统"] } } }
控制查询的返回数量
from从第几条数据开始
size获取几条数据
#控制查询的返回数量 #from从第几条数据开始 #size获取几条数据 GET jobbole/job/_search { "query": { "match": { "title": "搜索引擎" } }, "from": 0, "size": 3 }
match_all查询,查询所有数据
#match_all查询,查询所有数据 GET jobbole/job/_search { "query": { "match_all": {} } }
match_phrase查询
短语查询
短语查询,会将搜索词分词,放进一个列表如[python,开发]
然后搜索的字段必须满足列表里的所有元素,才符合
slop是设置分词后[python,开发]python 与 开发,之间隔着多少个字符算匹配
间隔字符数小于slop设置算匹配到,间隔字符数大于slop设置不匹配
#match_phrase查询 #短语查询 #短语查询,会将搜索词分词,放进一个列表如[python,开发] #然后搜索的字段必须满足列表里的所有元素,才符合 #slop是设置分词后[python,开发]python 与 开发,之间隔着多少个字符算匹配 #间隔字符数小于slop设置算匹配到,间隔字符数大于slop设置不匹配 GET jobbole/job/_search { "query": { "match_phrase": { "title": { "query": "elasticsearch引擎", "slop":3 } } } }
multi_match查询
比如可以指定多个字段
比如查询title字段和desc字段里面包含python的关键词数据
query设置搜索词
fields要搜索的字段
title^3表示权重,表示title里符合的关键词权重,是其他字段里符合的关键词权重的3倍
#multi_match查询 #比如可以指定多个字段 #比如查询title字段和desc字段里面包含python的关键词数据 #query设置搜索词 #fields要搜索的字段 #title^3表示权重,表示title里符合的关键词权重,是其他字段里符合的关键词权重的3倍 GET jobbole/job/_search { "query": { "multi_match": { "query": "搜索引擎", "fields": ["title^3","desc"] } } }
stored_fields设置搜索结果只显示哪些字段
注意:使用stored_fields要显示的字段store属性必须为true,如果要显示的字段没有设置store属性那么默认为false,如果为false将不会显示该字段
#stored_fields设置搜索结果只显示哪些字段 GET jobbole/job/_search { "stored_fields": ["title","company_name"], "query": { "multi_match": { "query": "搜索引擎", "fields": ["title^3","desc"] } } }
通过sort搜索结果排序
注意:排序的字段必须是数字或者日期
desc升序
asc降序
#通过sort搜索结果排序 #注意:排序的字段必须是数字或者日期 #desc升序 #asc降序 GET jobbole/job/_search { "query": { "match_all": {} }, "sort": [{ "comments": { "order": "asc" } }] }
range字段值范围查询
查询一个字段的值范围
注意:字段值必须是数字或者时间
gte大于等于
ge大于
lte小于等于
lt小于
boost是权重,可以给指定字段设置一个权重
#range字段值范围查询 #查询一个字段的值范围 #注意:字段值必须是数字或者时间 #gte大于等于 #ge大于 #lte小于等于 #lt小于 #boost是权重,可以给指定字段设置一个权重 GET jobbole/job/_search { "query": { "range": { "comments": { "gte": 10, "lte": 20, "boost": 2.0 } } } }
range字段值为时间范围查询
#range字段值为时间范围查询 #查询一个字段的时间值范围 #注意:字段值必须是时间 #gte大于等于 #ge大于 #lte小于等于 #lt小于 #now为当前时间 GET jobbole/job/_search { "query": { "range": { "add_time": { "gte": "2017-4-1", "lte": "now" } } } }
wildcard查询,通配符查询
*代表一个或者多个任意字符
#wildcard查询,通配符查询 #*代表一个或者多个任意字符 GET jobbole/job/_search { "query": { "wildcard": { "title": { "value": "py*n", "boost": 2 } } } }
fuzzy模糊查询
#fuzzy模糊搜索 #搜索包含词的内容 GET lagou/biao/_search { "query": { "fuzzy": {"title": "广告"} }, "_source": ["title"] } #fuzziness设置编辑距离,编辑距离就是把要查找的字段值,编辑成查找的关键词需要编辑多少个步骤(插入、删除、替换) #prefix_length为关键词前面不参与变换的长度 GET lagou/biao/_search { "query": { "fuzzy": { "title": { "value": "广告录音", "fuzziness": 2, "prefix_length": 2 } } }, "_source": ["title"] }
以上是关于Elasticsearch专题精讲——What's new in 8.7?的主要内容,如果未能解决你的问题,请参考以下文章
四十六 Python分布式爬虫打造搜索引擎Scrapy精讲—elasticsearch(搜索引擎)scrapy写入数据到elasticsearch中
第三百六十五节,Python分布式爬虫打造搜索引擎Scrapy精讲—elasticsearch(搜索引擎)的查询
四十四 Python分布式爬虫打造搜索引擎Scrapy精讲—elasticsearch(搜索引擎)的基本查询
第三百六十四节,Python分布式爬虫打造搜索引擎Scrapy精讲—elasticsearch(搜索引擎)的mapping映射管理
四十三 Python分布式爬虫打造搜索引擎Scrapy精讲—elasticsearch(搜索引擎)的mapping映射管理