论文阅读笔记《Stochastic Grounded Action Transformation for Robot Learning in Simulation》
Posted 垆边画船听雨眠
tags:
篇首语:本文由小常识网(cha138.com)小编为大家整理,主要介绍了论文阅读笔记《Stochastic Grounded Action Transformation for Robot Learning in Simulation》相关的知识,希望对你有一定的参考价值。
Stochastic Grounded Action Transformation for Robot Learning in Simulation
发表于IROS 2020(CCF C)
模拟中机器人学习的随机接地动作转换
Desai S, Karnan H, Hanna J P, et al. Stochastic grounded action transformation for robot learning in simulation[C]//2020 IEEE/RSJ International Conference on Intelligent Robots and Systems (IROS). IEEE, 2020: 6106-6111.
介绍
由于摩擦、齿轮间隙、不平地形和环境中的其他噪声源等影响,学习值的分布将更准确地反映现实世界。他们将注意力集中在将模拟器视为黑盒的接地算法上,例如接地动作转换(GAT)算法。
他们展示了GAT未能充分接地模拟器的几个领域,他们提出了一种新的算法,随机接地动作转换(SGAT),它通过学习环境中的随机性来优雅地处理这个问题。
方法
随机接地动作转换学习正向动力学的随机模型。换句话说,模型预测的是下一个状态的分布,而不是最有可能的下一个状态。
在连续状态和动作域中,他们将下一个状态建模为多元高斯分布,并使用负对数似然(NLL)损失 \\(\\mathcalL = - log\\ p(s_t+1|s_t, a_t)\\) 训练前向模型。与GAT类似,他们使用具有两个全连接的64个神经元隐藏层的神经网络函数逼近器来表示正向和逆模型,但与GAT不同的是,SGAT中的正向模型输出高斯分布的参数,他们从中采样预测的下一个状态。在他们的实现中,最后的全连接层为状态向量的每个元素输出平均值,\\(µ\\) 和对数标准偏差,\\(log(σ)\\)。
更多具体方法细节,参见本作的前代研究《Grounded Action Transformation for Robot Learning in Simulation》。
实验
悬崖行走(sim-to-sim)实验
他们使用经典的强化学习域悬崖行走(网格世界)验证了SGAT的好处。在这个领域中,智能体必须绕过悬崖才能到达目标。在他们的问题版本中,他们假设有一个确定性模拟器,但在“真实”环境中,在每个时间步骤中,智能体都有很小的机会朝着随机方向移动,而不是它选择的方向。
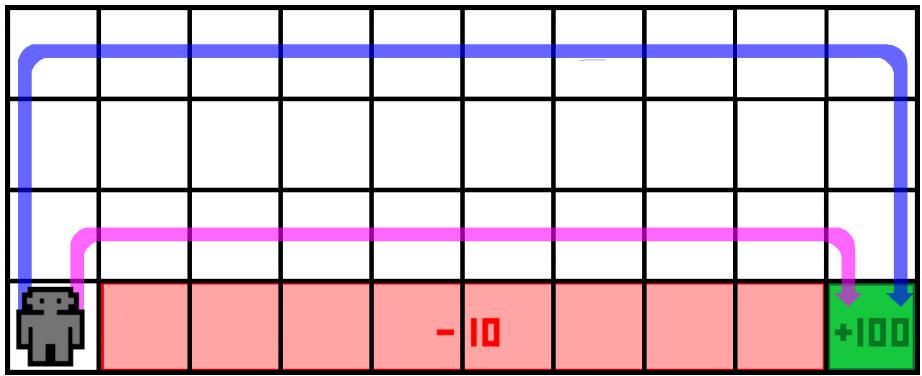
图7为不同环境噪声参数值下的GAT和SGAT。重复基础步骤和策略改进步骤,直到两种算法收敛为止。为了评估最终的策略,他们估计平均10,000集的预期回报。当值为0时,“真实”环境是完全确定的。当值为1时,每个跃迁都是随机的。因此,在这两个端点上,两种算法获得的预期收益之间没有区别。
对于每一个中间值,SGAT都优于GAT。
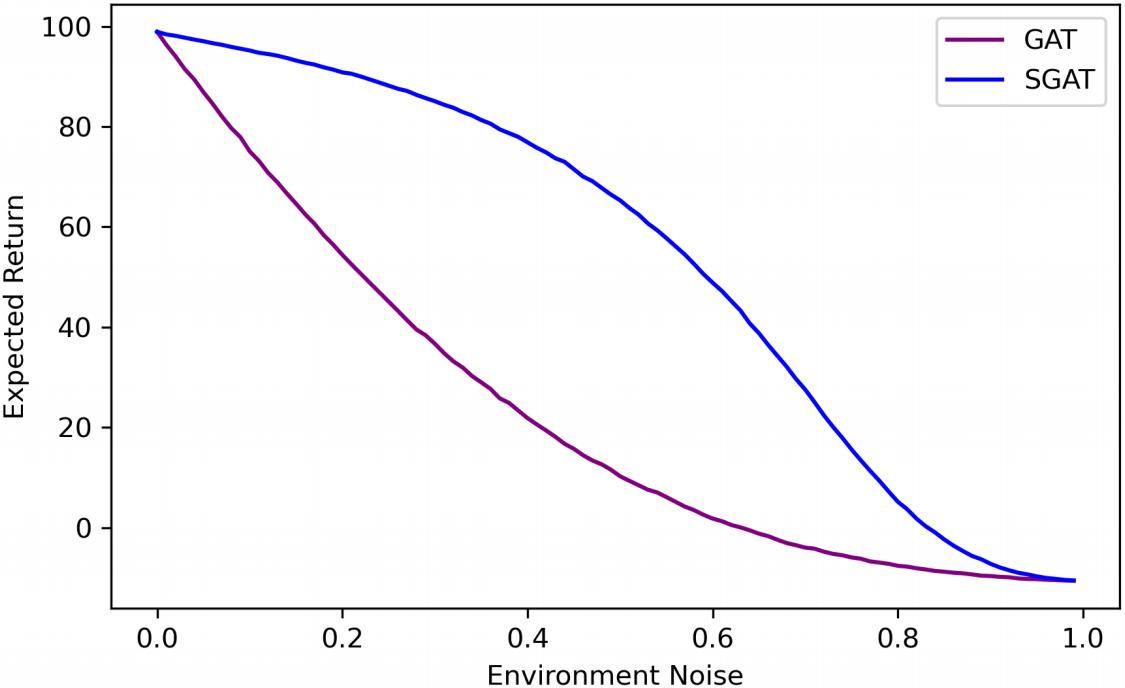
MuJoCo环境(sim-to-sim)实验
这篇论文研究了在连续控制领域中,结构化图注意力转移(SGAT)算法的性能评估。研究者在OpenAI Gym MuJoCo环境中进行实验,比较了SGAT和图注意力转移(GAT)算法在存在噪声的目标环境中的有效性。他们选择了Inverted Pendulum和Half Cheetah领域来测试SGAT在低维和高维状态及动作空间环境中的性能。结果表明,随着目标环境随机性的增加,使用SGAT学到的策略比使用GAT学到的策略表现更好,而动作噪声信封(ANE)方法只在特定的噪声值下表现良好。
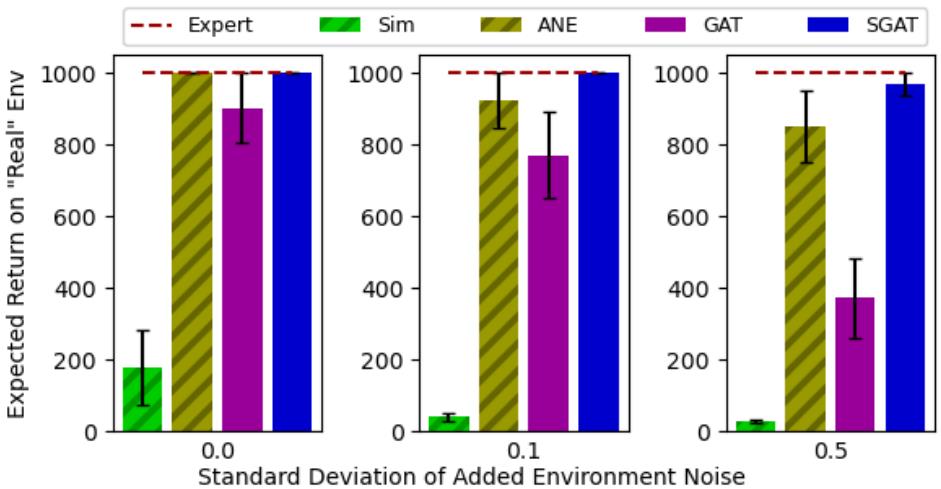
NAO 机器人(sim-to-real)实验
作者使用了 SoftBank NAO 机器人和 SimSpark 物理模拟器进行实验,在不平坦的地形上让机器人学习走路。作者比较了 GAT 和 SGAT 两种算法的效果,结果表明,SGAT 算法更成功,能够让机器人保持平衡走路。作者通过模拟器优化不稳定性来改进策略,最后在不平衡地面上进行了十次试验,结果表明,采用 SGAT 算法产生的策略比使用 GAT 的更为稳定,成功率更高。论文最终得出结论,SGAT 算法适用于现实世界中机器人学习走路的应用。
Speed (cm/s) | Falls | Speed (cm/s) | Falls | |
---|---|---|---|---|
GAT | 15.7 ± 2.98 | 6/10 | 18.5 ± 3.63 | 10/10 |
SGAT | 16.9 ± 0.678 | 0/10 | 18.0 ± 2.15 | 1/10 |
总结
这两种算法在确定性环境中的表现相似。在现实世界中,我们无法在测试前知道环境的随机程度。这一事实表明,我们应该默认使用SGAT。
pytorch 笔记: 复现论文 Stochastic Weight Completion for Road Networks using Graph Convolutional Networks(代
1 理论部分
2 导入库
import torch
import torch.nn.functional as F
import numpy as np
import pandas as pd
import os
from torch_geometric.data import Data, DataLoader
from torch_geometric.utils import normalized_cut
from torch_geometric.nn import (ChebConv, graclus, GCNConv,
max_pool, max_pool_x, global_mean_pool)
from toolz.curried import *
3 数据集处理
数据集来源是uber movement 以及纽约的osm 地图数据
Uber Movement: Let's find smarter ways forward, together.
np.random.seed(123)
torch.manual_seed(123)
uberdir = "D:/"
nykjuly = os.path.join(uberdir, "movement-speeds-hourly-new-york-2019-7.csv.zip")
device = torch.device('cuda' if torch.cuda.is_available() else 'cpu')
nodes, edges = load_gdfs("data/newyork/")
#There are 4588 nodes and 9893 edges in the road networks.
df = pd.read_csv(nykjuly)
#获取一个月的出行数据
df = attach_edgeid(nodes, edges, df)
#原来的shape:(25365815, 13)
#去掉了['segment_id','start_junction_id','end_junction_id']三列,加上了edge_id(表示这条边是edges里面的第edge_id条边】)
#同时只保留起止边和way_id都在edges里面的边
#去掉之后的shape:(2653457, 11),少了很多条出行记录
dG = edge_topology_from_edges(edges)
#相当于边变成点,如果原图两条边在一个点相交,那么转换之后的图中两个点有连边
#Construct edge topology from the `edges` (The graph with road segments as nodes).
n_test = 3 * 24
#一个月最后三天作为测试集,之前的是训练集
n_epochs = 5
batch_size = 1
obs, unobs = split_obs_unobs(df, ratio=0.9)
#obs——744个小时,每一个小时90%的数据(DataFrame)
#uobs——744个小时,每一个小时后10%的数据(DataFrame)
obs = [g for (_, g) in obs.groupby(['month', 'day', 'hour'])]
unobs = [g for (_, g) in unobs.groupby(['month', 'day', 'hour'])]
#obs——744个小时,每一个小时10%的数据(list)
#划分有观测点的数据和无观测点的数据(的ground truth)
3.1 load_gdfs
导入地图数据,获得点集和边集
#导入地图数据 def load_gdfs(datadir: str) -> Tuple[GeoDataFrame, GeoDataFrame]: """ Load the nodes and edges GeoDataFrame from graphml saved by `save_graphml_from_places`. Usage: nodes, edges = load_gdfs("data/newyork/") """ G = ox.load_graphml(os.path.join(datadir, "graph.graphml")) #导入地图数据 nodes, edges = ox.graph_to_gdfs(G, nodes=True, edges=True) # Convert a MultiDiGraph to node and/or edge GeoDataFrames. nodes['osmid'] = nodes.index.values ## the centroid coordindates of road segments points = edges.geometry.to_crs(epsg=3395).centroid #切换crs投影坐标,同时point表示边的质心 coords = pipe(points.map(lambda p: (p.x, p.y)).values, map(list), list, np.array) #将质心提取为ndarray的二维数组,每一个元素是质心的横纵坐标(变成ndarray的原因是方便找到每一列的min) coords = coords - coords.min(axis=0) #减去每一列的最小值 edges['coords'] = pipe(coords, map(tuple), list) #变回list,添加到coords这一列中 edges['osmid'] = edges.osmid.map(lambda x: x if isinstance(x, list) else [x]) u, v, _ = list(zip(*edges.index)) edges["u"] = u edges["v"] = v edges['id'] = np.arange(edges.shape[0]) edges.set_index('id', inplace=True, drop=False) #将id作为edges的活跃列(从0开始的数字) print(f"There are {nodes.shape[0]} nodes and {edges.shape[0]} edges in the road networks.") #导入多少列,出来多少列 return nodes, edges
类似于simplified操作
同时将newyork里面的crs坐标系转换成uber使用的坐标系nodes几乎没动
edges加了一个coords条目,表示的是边的质心,同时edges的活跃列改为idnodes:
edges:
![]()
3.2 attach_edgeid
def attach_edgeid(nodes: GeoDataFrame, edges: GeoDataFrame, df: DataFrame) -> DataFrame: """ Filter and attaching uber one-month dataframe `df` a graph edge-id column, where the edge id is determined by (u, v, osmid) and only rows with edge id are kept. Usage: mh = attach_edgeid(nodes, edges, df) """ ## filtering by node ids sdf = df[df.osm_start_node_id.isin(nodes.osmid)&df.osm_end_node_id.isin(nodes.osmid)].copy() #start和end的点都在nodes里面的那些df行 ## dropping columns that will not be used sdf.drop(["segment_id", "start_junction_id", "end_junction_id"], axis=1, inplace=True) #丢弃这三行 edgeidmap = {(u, v): (osmid, edgeid) for (u, v, osmid, edgeid) in zip(edges.u, edges.v, edges.osmid, edges.id)} #对edges中的这四个属性,拼成一个字典 def getedgeid(u: int, v: int, osmid: int) -> int: """ Map the (u, v, osmid) tuple to the corresponding graph edge id and return -1 if there is no such edge in the graph. """ osmids, edgeid = get((u, v), edgeidmap, ([-1], -1)) #在edgeidmap中找(u,v),如果找到了,返回edgeid,否则,返回-1 return edgeid if osmid in osmids else -1 #如果osmids有这个osmid,那么成立,否则,不成立 edge_idx_cols = ['osm_start_node_id', 'osm_end_node_id', 'osm_way_id'] sdf['edgeid'] = sdf[edge_idx_cols].apply(lambda x: getedgeid(*x), axis=1) #首先,判断一条记录的起点和终点在不在edges的起止点上;其次,判断这条边的id在不在edges (u,v)对应的里面 sdf = sdf[sdf.edgeid >= 0]#留下存在的边 return sdf
3.3 edge_topology_from_edges
def edge_topology_from_edges(edges: GeoDataFrame) -> Graph: """ Construct edge topology from the `edges` (The graph with road segments as nodes). nx.line_graph() can construct the line graph directly from the original graph. Args edges: Geodataframe returned by load_gdfs. Returns G: A undirected graph whose node ids are edge ids in `edges`. """ triple = pd.concat([pd.DataFrame({'id': edges.id, 'u': edges.u, 'v': edges.v}), pd.DataFrame({'id': edges.id, 'u': edges.v, 'v': edges.u})], ignore_index=True) #一条边两个方向 pairs = [] for (_, g) in triple.groupby('u'): pairs += [(u, v) for u in g.id for v in g.id if u != v] for (_, g) in triple.groupby('v'): pairs += [(u, v) for u in g.id for v in g.id if u != v] #同时从一个点出发\\同时从一个点到达的边000 G = Graph() G.add_edges_from(pairs) #相当于边变成点,如果原图两条边在一个点相交,那么转换之后的图中两个点有连边 return G ''' 某一个的GROUPBY id u v 0 0 42421728 42432736 1 1 42421728 42435337 2 2 42421728 42421731 9898 5 42421728 42421731 12211 2318 42421728 42432736 12942 3049 42421728 42435337 '''
3.4 split_obs_unobs
def split_dataframe(df: DataFrame, ratio: Optional[float]=0.9) -> Tuple[DataFrame, DataFrame]: """ Split a dataframe into two parts along the row dimension by the given ratio. """ k = int(df.shape[0] * ratio) #要选择的行数 idx = np.random.permutation(df.shape[0]) #随机排列序号,划分为前k个和后面的部分 return df.iloc[idx[:k]], df.iloc[idx[k:]] def split_obs_unobs(df: DataFrame, ratio: Optional[float]=0.9) -> Tuple[DataFrame, DataFrame]: """ Split a one-month dataframe into observed and unobserved dataframes. Returns trn: Observations for a fraction of road segments. tst: Ground truth for road segments to be inferred. """ ## we should guarantee the results are invariant to calling order. np.random.seed(123) dfs = [split_dataframe(g, ratio=ratio) for (_, g) in df.groupby(['month', 'day', 'hour'])] trn = pd.concat(pipe(dfs, map(first), list)) tst = pd.concat(pipe(dfs, map(second), list)) return trn, tst
3.5 uber_movement数据集补充说明
len(osm_ids.osm_way_id) | 33320 |
len(osm_ids.osm_start_node_id) | 58601 |
len(osm_ids.osm_end_node_id) | 58605 |
只有osm_way_id、osm_start_node_id、osm_end_node_id 加起来,才能唯一确定一个子路段
原因是因为,即使我们知道了osm_start_node_id、osm_end_node_id,但因为可能有地面、高架等不同的重叠路段,所以osm_way_id可能会不同(比如上图,黄颜色的是高架,黑线是地面道路。画红色箭头的是两个高架匝道,所以相同的osm_start_node_id、osm_end_node_id可能分别对应了地面和高架)
4 dataloader
trn_list = [get_data(dG, o, u) for (o, u) in zip(obs[:-n_test], unobs[:-n_test])]
tst_list = [get_data(dG, o, u) for (o, u) in zip(obs[-n_test:], unobs[-n_test:])]
#train_list的每一个元素都是torch_geometric的元素
#以第一个小时为例:Data(x=[9893, 1], edge_index=[2, 34637], y=[9893, 1])
#纽约一共有9893条边【edges.shape[0]】(在这里表示9893个点)
#这边的”边“相当于是,如果在纽约两条边有公共点,
#那么在我们的图上,边对应的点它们就相连
#trn_list和tst_list每一个元素是一个data数据
trn_loader = DataLoader(trn_list, batch_size=batch_size)
tst_loader = DataLoader(tst_list, batch_size=batch_size)
4.1 get_data
def get_x(df: DataFrame, num_nodes: int) -> torch.FloatTensor: """ Get pytorch geometric input feature from observation dataframe. Inputs df: The observation dataframe with edgeid being attached. Returns x (num_nodes, num_features): Input feature tensor. """ node_obs = {u: [v] for (u, v) in zip(df.edgeid.values, df.speed_mph_mean.values)} ## (num_nodes, 1) #一个字典,键值是这一小时每个edge_id,value是这个edge_id对应的平均速度 return torch.FloatTensor([get(u, node_obs, [0]) for u in range(num_nodes)]) #训练集:[速度] 其他的都是[0] #测试集:[速度] 其他的都是[0] def get_data(G: Graph, obs: DataFrame, unobs: DataFrame) -> Data: #obs和unobs是某一个小时观测数据和未观测数据 edge_index = get_edge_index(G) #utils中的函数,将G的边集转换成Tensor,然后转置 x = get_x(obs, G.number_of_nodes()) y = get_x(unobs, G.number_of_nodes()) #训练集:速度 其他的都是0 #测试集:速度 其他的都是0 return Data(x=x, edge_index=edge_index, y=y)
5 model部分
model = ChebNet(1, dG.number_of_nodes()).to(device)
optimizer = torch.optim.Adam(model.parameters(), lr=0.001)
class ChebNet(torch.nn.Module):
def __init__(self, num_features, num_nodes):
super(ChebNet, self).__init__()
self.conv1 = ChebConv(num_features, 32, 2)
#切比雪夫近似的GCN,2阶切比雪夫多项式近似(输入维度num_features,输出维度32,2阶切比雪夫)
self.conv2 = ChebConv(32, 64, 2)
#切比雪夫近似的GCN,2阶切比雪夫多项式近似(输入维度32,输出维度64,2阶切比雪夫)
self.fc1 = torch.nn.Linear(64, 128)
self.fc2 = torch.nn.Linear(128, num_nodes)
#两层全连接层
def forward(self, data):
#以第一张图为例:
#data:Batch(x=[9893, 1], edge_index=[2, 34637], y=[9893, 1], batch=[9893], ptr=[2])
x = F.relu(self.conv1(data.x, data.edge_index))
#切比雪夫近似GCN+RELU
#x:torch.Size([9893, 1])
cluster = graclus(data.edge_index, num_nodes=x.shape[0])
#图点分类,cluster是一个x.shape[0]维度的Tensor,表示每个点所在的cluster
#cluster:torch.Size([9893])
data = max_pool(cluster, Data(x=x, batch=data.batch, edge_index=data.edge_index))
#data:Batch(x=[5870, 32], edge_index=[2, 22026], batch=[5870])
#max_pool操作,根据cluster的分簇情况,重新构造图data
x = F.relu(self.conv2(data.x, data.edge_index))
#x:torch.Size([5847, 64])
cluster = graclus(data.edge_index, num_nodes=x.shape[0])
#cluster:5847维的tensor
x, batch = max_pool_x(cluster, x, data.batch)
'''
x.shape,batch.shape
(torch.Size([3436, 64]), torch.Size([3436]))+
'''
x = global_mean_pool(x, batch)
#torch.Size([1, 64])
x = F.relu(self.fc1(x))
#torch.Size([1, 128])
x = F.dropout(x, training=self.training)
x = self.fc2(x)
#torch.Size([1, num_nodes])
return x
6 训练模型
for epoch in range(n_epochs):
train(epoch, optimizer, trn_loader, model, device)
def train(epoch, optimizer, train_loader, model, device):
model.train()
losses = []
for data in train_loader:
data = data.to(device)
xhat = model(data)#预测的各个点的速度
## -> (batch_size, num_nodes)
x = data.x.reshape(xhat.shape)
nz = x > 0#保留观测集的那些点
loss = F.mse_loss(xhat[nz], x[nz], reduction='sum') / nz.sum().item()
optimizer.zero_grad()
loss.backward()
optimizer.step()
#老三部曲
losses.append(loss.item())
print(f"Epoch is {epoch}, Training Loss is {np.mean(losses):.5f}")
'''
Epoch is 0, Training Loss is 55.03807
Epoch is 1, Training Loss is 29.84954
Epoch is 2, Training Loss is 21.36361
Epoch is 3, Training Loss is 19.08718
Epoch is 4, Training Loss is 18.11195
Epoch is 5, Training Loss is 18.60411
Epoch is 6, Training Loss is 17.49593
Epoch is 7, Training Loss is 17.83597
Epoch is 8, Training Loss is 17.09360
Epoch is 9, Training Loss is 17.26834
Epoch is 10, Training Loss is 17.15905
Epoch is 11, Training Loss is 16.93761
Epoch is 12, Training Loss is 16.54925
Epoch is 13, Training Loss is 16.65559
Epoch is 14, Training Loss is 16.71426
Wall time: 12min 2s
'''
以上是关于论文阅读笔记《Stochastic Grounded Action Transformation for Robot Learning in Simulation》的主要内容,如果未能解决你的问题,请参考以下文章
pytorch 笔记: 复现论文 Stochastic Weight Completion for Road Networks using Graph Convolutional Networks(代
论文笔记:Stochastic Weight Completion for Road Networks using Graph Convolutional Networks
SWA(Stochastic Weight Averaging)实验
机器学习笔记:随机深度网络 stochastic depth