Python自然语言处理工具小结
Posted
tags:
篇首语:本文由小常识网(cha138.com)小编为大家整理,主要介绍了Python自然语言处理工具小结相关的知识,希望对你有一定的参考价值。
<‘ + ‘script>Python自然语言处理工具小结
作者:白宁超
2016年11月21日21:45:26
1 Python 的几个自然语言处理工具
- NLTK:NLTK 在用 Python 处理自然语言的工具中处于领先的地位。它提供了 WordNet 这种方便处理词汇资源的借口,还有分类、分词、除茎、标注、语法分析、语义推理等类库。
- Pattern:Pattern 的自然语言处理工具有词性标注工具(Part-Of-Speech Tagger),N元搜索(n-gram search),情感分析(sentiment analysis),WordNet。支持机器学习的向量空间模型,聚类,向量机。
-
TextBlob:TextBlob 是一个处理文本数据的 Python 库。提供了一些简单的api解决一些自然语言处理的任务,例如词性标注、名词短语抽取、情感分析、分类、翻译等等。
- Gensim:Gensim 提供了对大型语料库的主题建模、文件索引、相似度检索的功能。它可以处理大于RAM内存的数据。作者说它是“实现无干预从纯文本语义建模的最强大、最高效、最无障碍的软件。
- PyNLPI:它的全称是:Python自然语言处理库(Python Natural Language Processing Library,音发作: pineapple) 这是一个各种自然语言处理任务的集合,PyNLPI可以用来处理N元搜索,计算频率表和分布,建立语言模型。他还可以处理向优先队列这种更加复杂的数据结构,或者像 Beam 搜索这种更加复杂的算法。
- spaCy:这是一个商业的开源软件。结合Python和Cython,它的自然语言处理能力达到了工业强度。是速度最快,领域内最先进的自然语言处理工具。
- Polyglot:Polyglot 支持对海量文本和多语言的处理。它支持对165种语言的分词,对196中语言的辨识,40种语言的专有名词识别,16种语言的词性标注,136种语言的情感分析,137种语言的嵌入,135种语言的形态分析,以及69中语言的翻译。
- MontyLingua:MontyLingua 是一个自由的、训练有素的、端到端的英文处理工具。输入原始英文文本到 MontyLingua ,就会得到这段文本的语义解释。适合用来进行信息检索和提取,问题处理,回答问题等任务。从英文文本中,它能提取出主动宾元组,形容词、名词和动词短语,人名、地名、事件,日期和时间,等语义信息。
- BLLIP Parser:BLLIP Parser(也叫做Charniak-Johnson parser)是一个集成了产生成分分析和最大熵排序的统计自然语言工具。包括 命令行 和 python接口 。
- Quepy:Quepy是一个Python框架,提供将自然语言转换成为数据库查询语言。可以轻松地实现不同类型的自然语言和数据库查询语言的转化。所以,通过Quepy,仅仅修改几行代码,就可以实现你自己的自然语言查询数据库系统。GitHub:https://github.com/machinalis/quepy
- HanNLP:HanLP是由一系列模型与算法组成的Java工具包,目标是普及自然语言处理在生产环境中的应用。不仅仅是分词,而是提供词法分析、句法分析、语义理解等完备的功能。HanLP具备功能完善、性能高效、架构清晰、语料时新、可自定义的特点。文档使用操作说明:Python调用自然语言处理包HanLP 和 菜鸟如何调用HanNLP
2 OpenNLP:进行中文命名实体识别
OpenNLP是Apach下的Java自然语言处理API,功能齐全。如下给大家介绍一下使用OpenNLP进行中文语料命名实体识别的过程。
首先是预处理工作,分词去听用词等等的就不啰嗦了,其实将分词的结果中间加上空格隔开就可以了,OpenNLP可以将这样形式的的语料照处理英文的方式处理,有些关于字符处理的注意点在后面会提到。
其次我们要准备各个命名实体类别所对应的词库,词库被存在文本文档中,文档名即是命名实体类别的TypeName,下面两个function分别是载入某类命名实体词库中的词和载入命名实体的类别。
/** * 载入词库中的命名实体 * * @param nameListFile * @return * @throws Exception */ public static List<String> loadNameWords(File nameListFile) throws Exception { List<String> nameWords = new ArrayList<String>(); if (!nameListFile.exists() || nameListFile.isDirectory()) { System.err.println("不存在那个文件"); return null; } BufferedReader br = new BufferedReader(new FileReader(nameListFile)); String line = null; while ((line = br.readLine()) != null) { nameWords.add(line); } br.close(); return nameWords; } /** * 获取命名实体类型 * * @param nameListFile * @return */ public static String getNameType(File nameListFile) { String nameType = nameListFile.getName(); return nameType.substring(0, nameType.lastIndexOf(".")); }
因为OpenNLP要求的训练语料是这样子的:
XXXXXX<START:Person>????<END>XXXXXXXXX<START:Action>????<END>XXXXXXX
被标注的命名实体被放在<START><END>范围中,并标出了实体的类别。接下来是对命名实体识别模型的训练,先上代码:
import java.io.File; import java.io.FileOutputStream; import java.io.IOException; import java.io.StringReader; import java.util.Collections; import opennlp.tools.namefind.NameFinderME; import opennlp.tools.namefind.NameSample; import opennlp.tools.namefind.NameSampleDataStream; import opennlp.tools.namefind.TokenNameFinderModel; import opennlp.tools.util.ObjectStream; import opennlp.tools.util.PlainTextByLineStream; import opennlp.tools.util.featuregen.AggregatedFeatureGenerator; import opennlp.tools.util.featuregen.PreviousMapFeatureGenerator; import opennlp.tools.util.featuregen.TokenClassFeatureGenerator; import opennlp.tools.util.featuregen.TokenFeatureGenerator; import opennlp.tools.util.featuregen.WindowFeatureGenerator; /** * 中文命名实体识别模型训练组件 * * @author ddlovehy * */ public class NamedEntityMultiFindTrainer { // 默认参数 private int iterations = 80; private int cutoff = 5; private String langCode = "general"; private String type = "default"; // 待设定的参数 private String nameWordsPath; // 命名实体词库路径 private String dataPath; // 训练集已分词语料路径 private String modelPath; // 模型存储路径 public NamedEntityMultiFindTrainer() { super(); // TODO Auto-generated constructor stub } public NamedEntityMultiFindTrainer(String nameWordsPath, String dataPath, String modelPath) { super(); this.nameWordsPath = nameWordsPath; this.dataPath = dataPath; this.modelPath = modelPath; } public NamedEntityMultiFindTrainer(int iterations, int cutoff, String langCode, String type, String nameWordsPath, String dataPath, String modelPath) { super(); this.iterations = iterations; this.cutoff = cutoff; this.langCode = langCode; this.type = type; this.nameWordsPath = nameWordsPath; this.dataPath = dataPath; this.modelPath = modelPath; } /** * 生成定制特征 * * @return */ public AggregatedFeatureGenerator prodFeatureGenerators() { AggregatedFeatureGenerator featureGenerators = new AggregatedFeatureGenerator( new WindowFeatureGenerator(new TokenFeatureGenerator(), 2, 2), new WindowFeatureGenerator(new TokenClassFeatureGenerator(), 2, 2), new PreviousMapFeatureGenerator()); return featureGenerators; } /** * 将模型写入磁盘 * * @param model * @throws Exception */ public void writeModelIntoDisk(TokenNameFinderModel model) throws Exception { File outModelFile = new File(this.getModelPath()); FileOutputStream outModelStream = new FileOutputStream(outModelFile); model.serialize(outModelStream); } /** * 读出标注的训练语料 * * @return * @throws Exception */ public String getTrainCorpusDataStr() throws Exception { // TODO 考虑入持久化判断直接载入标注数据的情况 以及增量式训练 String trainDataStr = null; trainDataStr = NameEntityTextFactory.prodNameFindTrainText( this.getNameWordsPath(), this.getDataPath(), null); return trainDataStr; } /** * 训练模型 * * @param trainDataStr * 已标注的训练数据整体字符串 * @return * @throws Exception */ public TokenNameFinderModel trainNameEntitySamples(String trainDataStr) throws Exception { ObjectStream<NameSample> nameEntitySample = new NameSampleDataStream( new PlainTextByLineStream(new StringReader(trainDataStr))); System.out.println("**************************************"); System.out.println(trainDataStr); TokenNameFinderModel nameFinderModel = NameFinderME.train( this.getLangCode(), this.getType(), nameEntitySample, this.prodFeatureGenerators(), Collections.<String, Object> emptyMap(), this.getIterations(), this.getCutoff()); return nameFinderModel; } /** * 训练组件总调用方法 * * @return */ public boolean execNameFindTrainer() { try { String trainDataStr = this.getTrainCorpusDataStr(); TokenNameFinderModel nameFinderModel = this .trainNameEntitySamples(trainDataStr); // System.out.println(nameFinderModel); this.writeModelIntoDisk(nameFinderModel); return true; } catch (Exception e) { // TODO Auto-generated catch block e.printStackTrace(); return false; } } }
注:
- 参数:iterations是训练算法迭代的次数,太少了起不到训练的效果,太大了会造成过拟合,所以各位可以自己试试效果;
- cutoff:语言模型扫描窗口的大小,一般设成5就可以了,当然越大效果越好,时间可能会受不了;
- langCode:语种代码和type实体类别,因为没有专门针对中文的代码,设成“普通”的即可,实体的类别因为我们想训练成能识别多种实体的模型,于是设置为“默认”。
说明:
- prodFeatureGenerators()方法用于生成个人订制的特征生成器,其意义在于选择什么样的n-gram语义模型,代码当中显示的是选择窗口大小为5,待测命名实体词前后各扫描两个词的范围计算特征(加上自己就是5个),或许有更深更准确的意义,请大家指正;
- trainNameEntitySamples()方法,训练模型的核心,首先是将如上标注的训练语料字符串传入生成字符流,再通过NameFinderME的train()方法传入上面设定的各个参数,订制特征生成器等等,关于源实体映射对,就按默认传入空Map就好了。
源代码开源在:https://github.com/Ailab403/ailab-mltk4j,test包里面对应有完整的调用demo,以及file文件夹里面的测试语料和已经训练好的模型。
3 StanfordNLP:实现中文命名实体识别
使用Stanford Word Segmenter and Stanford Named Entity Recognizer (NER)实现中文命名实体识别 - 以家为家,以乡为乡,以国为国,以天下为天下 - 博客频道 - CSDN.NET
1、分词介绍
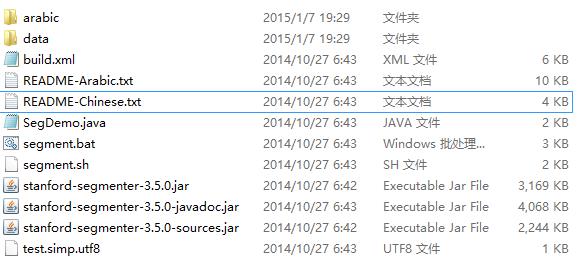
2、NER介绍
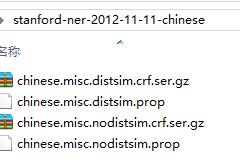
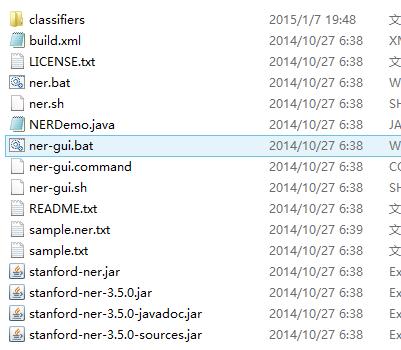
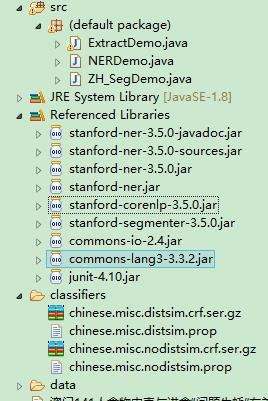

import edu.stanford.nlp.ie.AbstractSequenceClassifier; import edu.stanford.nlp.ie.crf.CRFClassifier; import edu.stanford.nlp.ling.CoreLabel; /** * * <p> * ClassName ExtractDemo * </p> * <p> * Description 加载NER模块 * */ public class ExtractDemo { private static AbstractSequenceClassifier<CoreLabel> ner; public ExtractDemo() { InitNer(); } public void InitNer() { String serializedClassifier = "classifiers/chinese.misc.distsim.crf.ser.gz"; // chinese.misc.distsim.crf.ser.gz if (ner == null) { ner = CRFClassifier.getClassifierNoExceptions(serializedClassifier); } } public String doNer(String sent) { return ner.classifyWithInlineXML(sent); } public static void main(String args[]) { String str = "我 去 吃饭 , 告诉 李强 一声 。"; ExtractDemo extractDemo = new ExtractDemo(); System.out.println(extractDemo.doNer(str)); System.out.println("Complete!"); } }
import java.io.File; import java.io.IOException; import java.util.Properties; import org.apache.commons.io.FileUtils; import edu.stanford.nlp.ie.crf.CRFClassifier; import edu.stanford.nlp.ling.CoreLabel; /** * * <p> * Description 使用Stanford CoreNLP进行中文分词 * </p> * */ public class ZH_SegDemo { public static CRFClassifier<CoreLabel> segmenter; static { // 设置一些初始化参数 Properties props = new Properties(); props.setProperty("sighanCorporaDict", "data"); props.setProperty("serDictionary", "data/dict-chris6.ser.gz"); props.setProperty("inputEncoding", "UTF-8"); props.setProperty("sighanPostProcessing", "true"); segmenter = new CRFClassifier<CoreLabel>(props); segmenter.loadClassifierNoExceptions("data/ctb.gz", props); segmenter.flags.setProperties(props); } public static String doSegment(String sent) { String[] strs = (String[]) segmenter.segmentString(sent).toArray(); StringBuffer buf = new StringBuffer(); for (String s : strs) { buf.append(s + " "); } System.out.println("segmented res: " + buf.toString()); return buf.toString(); } public static void main(String[] args) { try { String readFileToString = FileUtils.readFileToString(new File("澳门141人食物中毒与进食“问题生蚝”有关.txt")); String doSegment = doSegment(readFileToString); System.out.println(doSegment); ExtractDemo extractDemo = new ExtractDemo(); System.out.println(extractDemo.doNer(doSegment)); System.out.println("Complete!"); } catch (IOException e) { e.printStackTrace(); } } }
注意一定是JDK 1.8+的环境,最后输出结果如下:
以上是关于Python自然语言处理工具小结的主要内容,如果未能解决你的问题,请参考以下文章
Python-turtle库知识小结(python绘图工具)