论文阅读:You Only Look Once: Unified, Real-Time Object Detection
Posted chenxp2311
tags:
篇首语:本文由小常识网(cha138.com)小编为大家整理,主要介绍了论文阅读:You Only Look Once: Unified, Real-Time Object Detection相关的知识,希望对你有一定的参考价值。
Preface
今天详细的看一下 CVPR 2016 年这篇:You Only Look Once: Unified, Real-Time Object Detection。另外,这篇的作者也有Ross B. Girshick。
这篇 Paper 的项目主页在这里:http://pjreddie.com/darknet/yolo/
注:这篇今年 CVPR 2016 年的检测文章 YOLO,我之前写过这篇文章的解读。但因为不小心在 Markdown 编辑器中编辑时删除了。幸好同组的伙伴转载了我的,我就直接考过来了,重新发一下。以后得给自己的博文留个备份。
abstract
这篇文章提出了一个新的物体检测的方法:You Only Look Once(YOLO)。
之前的物体检测方法通常都转变为了一个分类问题,如 R-CNN、Fast R-CNN 等等。另外,关于对 R-CNN、Fast R-CNN、Faster R-CNN 这一系列方法,知乎上有个特别好的帖子:如何评价rcnn、fast-rcnn和faster-rcnn这一系列方法?
而这篇文章将检测变为一个 regression problem,YOLO 从输入的图像,仅仅经过一个 neural network,直接得到 bounding boxes 以及每个 bounding box 所属类别的概率。正因为整个的检测过程仅仅有一个网络,所以它可以直接 end-to-end 的优化。
YOLO 结构十分的快,标准的 YOLO 版本每秒可以实时地处理 45 帧图像。一个较小版本:Fast YOLO,可以每秒处理 155 帧图像,它的 mAP(mean Average Precision) 依然可以达到其他实时检测算法的两倍。
同时相比较于其他的 state-of-art detection systems。尽管 YOLO 的定位更容易出错,这里的 定位出错,即是指 coordinate errors。
但是 YOLO 有更少的 false-positive,文章这里提到了一个词:background errors,背景误差。这里所谓的 背景误差 即是指 False Positive。在这篇 Paper 的Assigned Reviewer 里,有 Reviewer 提到了这个问题:
On overall, the paper reads well, even if some terms such as IOU (I guess it’s the abbreviation of intersection over union but it would be better to say it as it’s not a standard abbreviation) or “background errors” (I’m not really sure of the meaning of this expression. Are they False Positive? If yes, it should be better to use False Positive instead).
![]()
最后,YOLO 可以学习到物体的更加泛化的特征,在将 YOLO 用到其他领域的图像时(如 artwork 的图像上),其检测效果要优于 DPM、R-CNN 这类方法。
Introduction
现在的 detection systems 将物体检测问题,最后会转变成一个分类问题。在检测中,detection systems 采用一个 classifier 去评估一张图像中,各个位置一定区域的 window 或 bounding box 内,是否包含一个物体?包含了哪种物体?
一些 detection systems,如 Deformable Parts Models(DPM),采用的是 sliding window 的方式去检测。
最近的 R-CNN、Fast R-CNN 则采用的是 region proposals 的方法,先生成一些可能包含待检测物体的 potential bounding box,再通过一个 classifier 去判断每个 bounding box 里是否包含有物体,以及物体所属类别的 probability 或者 confidence。这种方法的 pipeline 需要经过好几个独立的部分,所以检测速度很慢,也难以去优化,因为每个独立的部分都需要单独训练。
本文将 object detection 的框架设计为一个 regression problem。直接从图像像素到 bounding box 以及 probabilities。这个 YOLO 系统如图看了一眼图像就能 predict 是否存在物体,他们在哪个位置,所以也才叫 You Only Look Once。
YOLO 的 idea 十分简单,如 Figure 1:
将图像输入单独的一个 CNN 网络,就会 predict 出 bounding boxes,以及这些 bounding boxes 所属类别的概率。YOLO 用一整幅图像来训练,同时可以直接优化 detection performance。
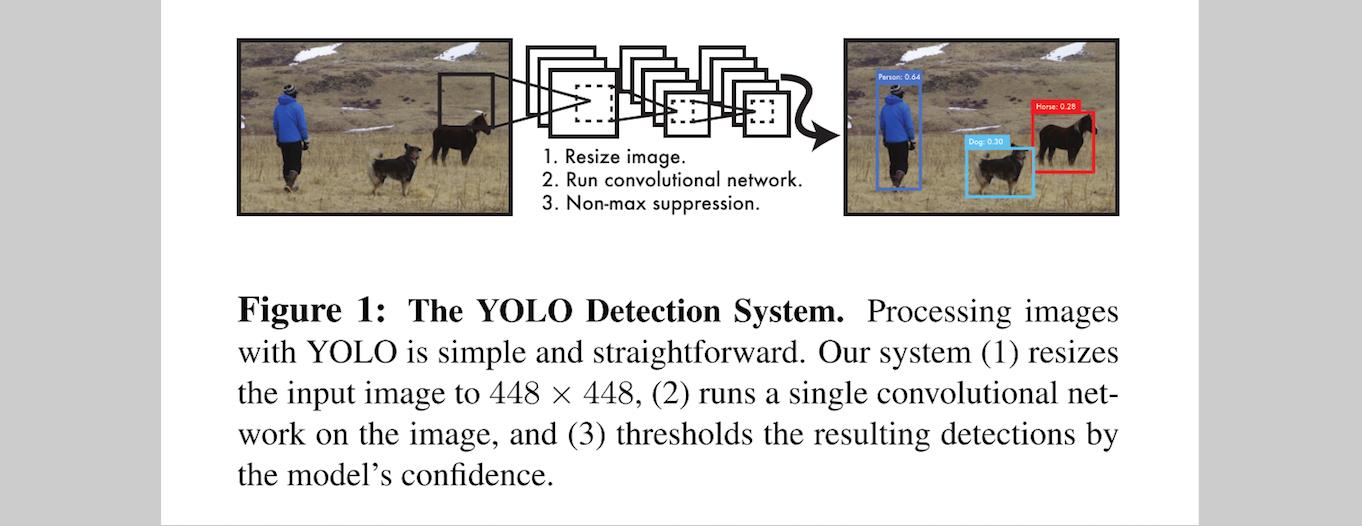
这样的统一的架构,对比之前如 R-CNN、Fast R-CNN 的 pipeline,有以下几点好处:
(1)YOLO 检测系统非常非常的快。受益于将 detection 架构设计成一个 regression problem,以及简单的 pipeline。在 Titan X 上,不需要经过批处理,标准版本的 YOLO 系统可以每秒处理 45 张图像;YOLO 的极速版本可以处理 150 帧图像。这就意味着 YOLO 可以以小于 25 毫秒延迟的处理速度,实时地处理视频。同时,YOLO 实时检测的mean Average Precision(mAP) 是其他实时检测系统的两倍。
(2)YOLO 在做 predict 的时候,YOLO 使用的是全局图像。与 sliding window 和 region proposals 这类方法不同,YOLO 一次“看”一整张图像,所以它可以将物体的整体(contextual)的 class information 以及 appearance information 进行 encoding。目前最快最好的Fast R-CNN ,较容易误将图像中的 background patches 看成是物体,因为它看的范围比较小。YOLO 的 background errors 比 Fast R-CNN 少一半多。
(3)YOLO 学到物体更泛化的特征表示。当在自然场景图像上训练 YOLO,再在 artwork 图像上去测试 YOLO 时,YOLO 的表现甩 DPM、R-CNN 好几条街。YOLO 模型更能适应新的 domain。
Unified Detection
YOLO 检测系统,先将输入图像分成 S×S 个 grid(栅格),如果一个物体的中心掉落在一个 grid cell 内,那么这个 grid cell 就负责检测这个物体。
每一个 grid cell 预测 B 个 bounding boxes,以及这些 bounding boxes 的得分:score。这个 score 反应了模型对于这个 grid cell 中预测是否含有物体,以及是这个物体的可能性是多少。正式的公式: Pr(Object)∗IOUtruthpred 。如果这个 cell 中不存在一个 object,则 score 应该为 0 ;否则的话,score 则为 predicted box 与 ground truth 之间的 IoU(intersection over union)。
本文中的每一个 bounding box 包含了 5 个 predictions: x,y,w,h,confidence ,坐标 (x,y) 代表了 bounding box 的中心与 grid cell 边界的相对值。width、height 则是相对于整幅图像的预测值。confidence 就是 IoU 值。
每一个 grid cell 还要预测 C 个 conditional class probability(条件类别概率): Pr(Classi|Object) 。这个 C 基于 gird cell 包含了哪个物体(所以为 conditional probabilities)。不管 grid cell 中包含的 boxes 有多少 B ,每个 grid cell 只 predict 每个类别的 conditional probabilities。
在测试阶段,将每个 grid cell 的 conditional class probabilities 与每个 bounding box 的 confidence 相乘:
上面得到每个 bounding box 的具体类别的 confidence score。这样就把 bounding box 中预测的 class 的 probability,以及 bounding box 与 object 契合的有多好,都进行了 encoding。
将 YOLO 用于 PASCAL VOC 数据集时:
- 本文使用的 S=7 ,即将一张图像分为 7×7=49 个 grid cells
- 每一个 grid cell 预测 B=2 个 boxes(每个 box 是 (x,y,w,h,confidence) , 5 个数值)
- 同时,PASCAL 数据集中有 20 个类别,则,上面的 C=20
因此,最后的 prediction 是
7×7×30
的 tensor
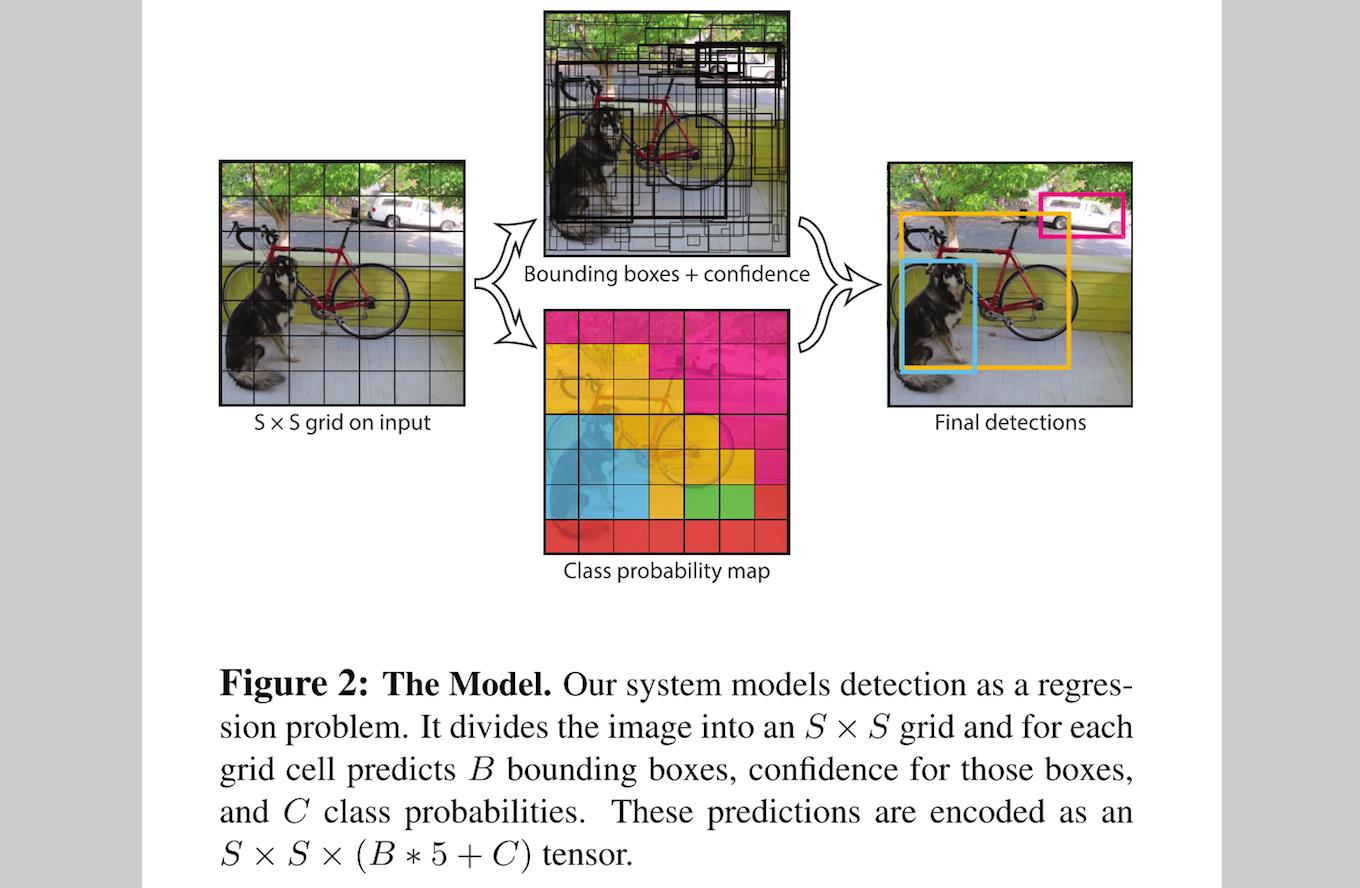
Network Design
YOLO 仍是 CNN 的经典形式,开始是 convolutional layers 提取特征,再是 fully connected layers 进行 predict 结果:probabilities 以及 coordinates。
YOLO 的 CNN 结构取自两篇论文:GoogLeNet、Network in Network. YOLO 有
24
个卷积层,随后就是全连接层。不像 GoogLeNet 中使用的 inception modules,YOLO 采用了 Network in Network 中的结构,在
3×3
卷积层之后,跟着一个
1×1
的层。如下图 Figure 3 所示:

网络结构,更详细的如下表所示:
Layers | Parameters |
---|---|
Input Data | Images Size: 448×448 |
Convolution | num_filters: 64 , kernel size: 7×7 , stride: 2 |
Max Pooling | kernel size: 2×2 , stride: 2 |
Convolution | num_filters: 192 , kernel size: 3×3 , stride: 1 |
Max Pooling | kernel size: 2×2 , stride: 2 |
Convolution | num_filters: 128 , kernel size: 1×1 , stride: 1 |
Convolution | num_filters: 256 |