PyTorch Lecture 07: Wide and Deep
Posted 努力奋斗-不断进化
tags:
篇首语:本文由小常识网(cha138.com)小编为大家整理,主要介绍了PyTorch Lecture 07: Wide and Deep相关的知识,希望对你有一定的参考价值。
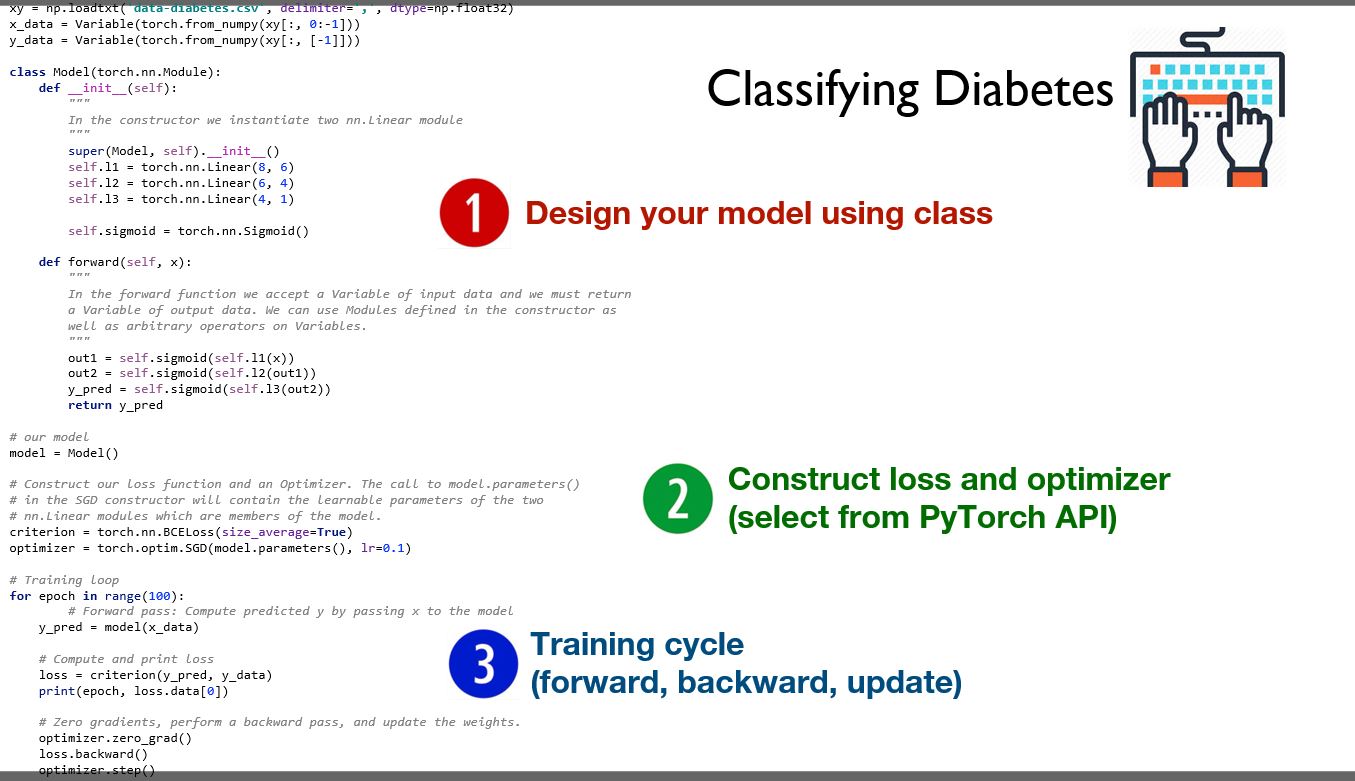
import torch
from torch.autograd import Variable
import numpy as np
xy = np.loadtxt('./data/diabetes.csv.gz', delimiter=',', dtype=np.float32)
x_data = Variable(torch.from_numpy(xy[:, 0:-1]))
y_data = Variable(torch.from_numpy(xy[:, [-1]]))
print(xy[:, 0:-1].shape) # 看输入数据的大小
print(xy[:, [-1]].shape) # 看输入数据的大小
class Model(torch.nn.Module):
def __init__(self):
"""
In the constructor we instantiate two nn.Linear module
"""
super(Model, self).__init__()
self.l1 = torch.nn.Linear(8, 6)
self.l2 = torch.nn.Linear(6, 4)
self.l3 = torch.nn.Linear(4, 1)
self.sigmoid = torch.nn.Sigmoid()
def forward(self, x):
out1 = self.sigmoid(self.l1(x))
out2 = self.sigmoid(self.l2(out1))
y_pred = self.sigmoid(self.l3(out2))
return y_pred
# Our model
model = Model()
# Construct our loss function and an Optimizer. The call to model.parameters()
# in the SGD constructor will contain the learnable parameters of the two
# nn.Linear modules which are members of the model.
criterion = torch.nn.BCELoss(size_average=True)
optimizer = torch.optim.SGD(model.parameters(), lr=0.1)
# Training loop
for epoch in range(100):
y_pred=model(x_data)
# Compute and print loss
loss=criterion(y_pred,y_data)
print(epoch,loss.data[0])
# Zero gradients,perform a backward pass,and update the weights
optimizer.zero_grad()
loss.backward()
optimizer.step()
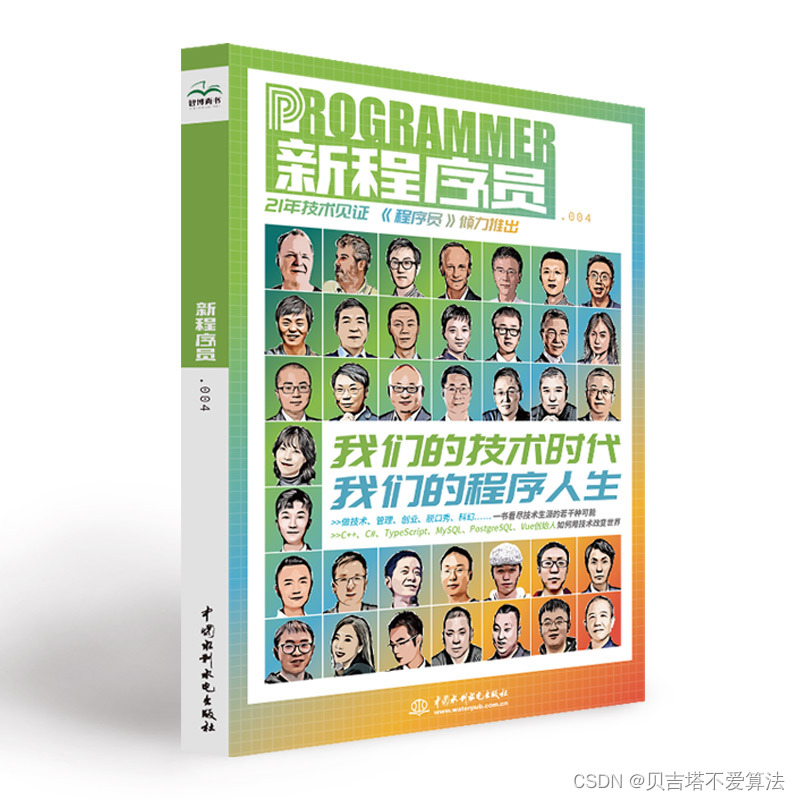

以上是关于PyTorch Lecture 07: Wide and Deep的主要内容,如果未能解决你的问题,请参考以下文章
[PyTorch]PyTorch中模型的参数初始化的几种方法(转)