论文精选|基于深度神经网络的实时作业车间RFID订单完成时间预测
Posted JIM期刊
tags:
篇首语:本文由小常识网(cha138.com)小编为大家整理,主要介绍了论文精选|基于深度神经网络的实时作业车间RFID订单完成时间预测相关的知识,希望对你有一定的参考价值。
文章发表于JIM期刊,出版于2017年4月
链接:https://doi.org/10.1007/s10845-017-1325-3
摘要
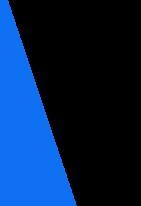
在传统的订单完成时间(OCT)预测方法中,经常会用到一些可变的、理想的生产数据(如在制品到达时间、所有工序的计划加工时间、每道工序的预计等待时间)。因此,对于OCT预测方法,虽然考虑了生产能力的动态性和车间的实时负荷情况,但预测时间总是与实际完工时间有很大的偏差。基于此,本文提出了一种新的基于订单和实时车间RFID数据的OCT预测方法。应用精确的RFID数据描述车间的实时负荷状况,并尝试从历史数据中挖掘RFID数据与OCT的映射关系。
首先,RFID设备捕捉加工工作站库存、出库的所有在制品的类型和等待列表信息,以及在工作站上正在加工的所有在制品的实时加工进度。其次,利用RFID数据,提出了一种实时作业车间负荷状态的描述模型,建立了基于订单和实时RFID数据组合的映射关系。最后,将深度神经网络的主要技术之一deep belief network(深度信念网络)应用于映射关系的挖掘。为了说明该方法的优越性,与基于BP网络的预测方法、基于多隐层BP网络的预测方法、基于主成分分析和BP网络的预测方法进行了对比实验。
文章导读
本文提出了一种基于RFID的作业车间实时负荷状况描述模型进行OCT预测。通过加工工作站在库存和出库的所有WIPs的类型和等待清单信息,以及加工工作站正在加工的所有WIPs的实时加工进度,来描述实时的负载情况。RFID系统不仅可以跟踪每一个订单的生产进度,还可以提供大量的历史生产数据。此外,利用DNN从历史生产数据中了解实时作业车间负荷状况与OCT之间的映射关系。
本文结合了以下三个方面的工作:(1)利用RFID数据描述作业车间的实时负荷状况;(2)根据WIPs的类型、等待序列、实时处理进度等信息对OCT进行预测;(3)将DNN引入OCT预测,解决了神经网络在大规模系统应用中的不足。
本文引入了深度信念网络deep belief network。为了建立OCT预测函数,可以将历史生产信息HI作为训练DBN的输入数据,包括订单信息、相应的作业车间负载条件。DBN的训练是通过逐个RBM单元的训练来完成的,如图1所示。
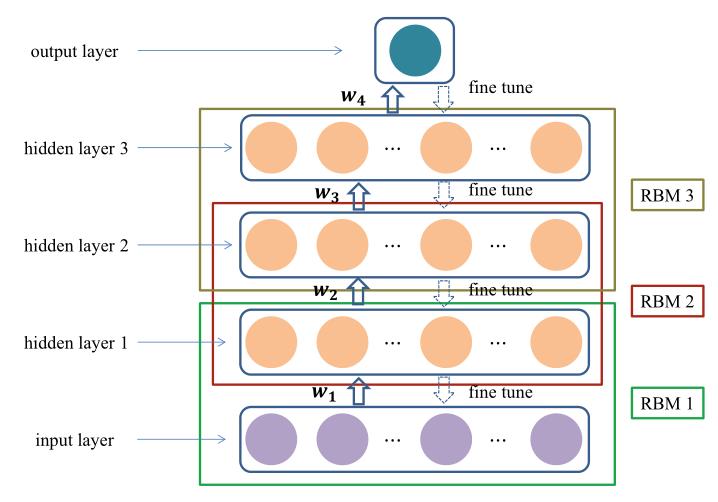
图1 DBN框架
这种训练方法代表了一种有效的学习方式,它结合了多个更简单的RBM模型,并按顺序学习。从训练过程可以看出逐层学习算法是无监督的。为了提高DBN模型的预测精度,在预训练后,采用BP训练算法对DBN进行训练。与每次只考虑一个RBM的预训练过程不同,BP同时考虑所有DBN层。BP使用实际完成时间AT进行DBN模型的训练。利用DBN模型输出PTV和实际完成量来计算训练误差。训练过程如图2所示。这里给出主要的对比实验训练结果。
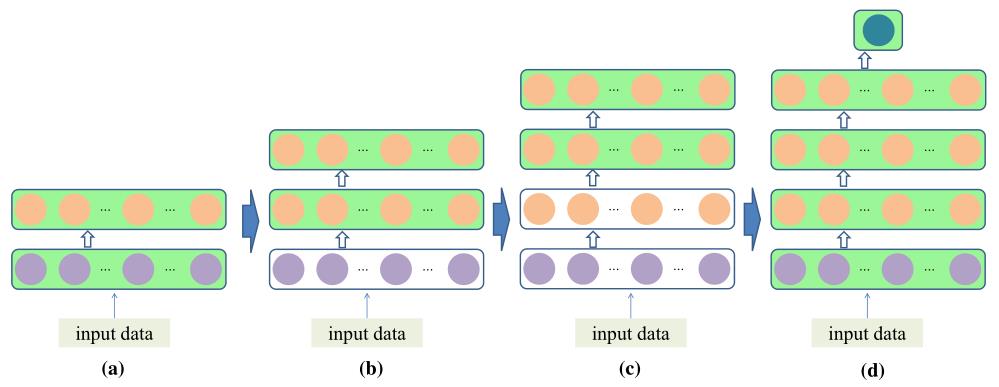
图2 DBN的训练过程
不同预测结果的统计分析
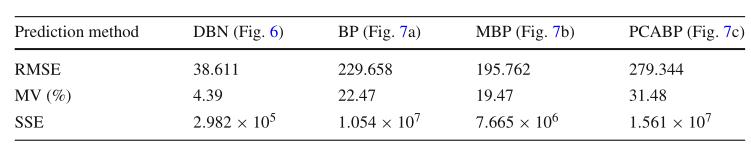
原文信息
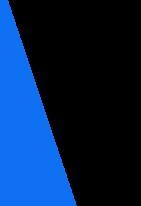
Abstract
In the traditional order completion time (OCT) prediction methods, some mutable and ideal production data (e.g., the arrival time of work in process (WIP), the planned processing time of all operations, and the expected waiting time per operation) are often used. Thus, the prediction time always deviates from the actual completion time dramatically even though the dynamicity of the production capacity and the real-time load conditions of job shop are considered in the OCT prediction method. On account of this, a new prediction method of OCT using the composition of order and real-time job shop RFID data is proposed in this article. It applies accurate RFID data to depict the real-time load conditions of job shop, and attempts to mine the mapping relationship between RFID data and OCT from historical data. Firstly, RFID devices capture the types and waiting list information of all WIPs which are in the in-stocks and out-stocks of machining workstations, and the real-time processing progress of all WIPs which are under machining at machining workstations. Secondly, a description model of real-time job shop load conditions is put forward by using the RFID data. Next, the mapping model based on the composition of order and real-time RFID data is established. Finally, deep belief network, which is one of the major technologies of deep neural networks, is applied to mine the mapping relationship. To illustrate the advantages of the proposed method, a numerical experiment compared with back-propagation (BP) network based prediction method, multi-hidden-layers BP network based prediction method and the principal components analysis and BP network based prediction method is conducted at last.
Keywords
Order completion time prediction
Deep neural networks
DBN
RFID
Cite this article as:
Wang, C., Jiang, P. Deep neural networks based order completion time prediction by using real-time job shop RFID data. J Intell Manuf 30, 1303–1318 (2019).
/本期编辑/
陈妤
上海交通大学 硕士在读
研究方向:复杂网络、系统优化
/本期审核/
肖智文
武汉科技大学 本科在读
工业工程
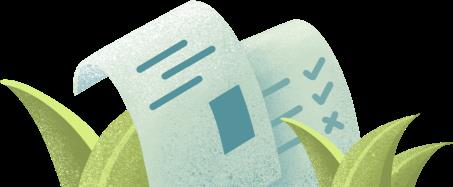
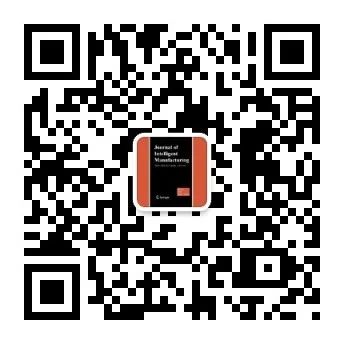
聚焦智能制造;传播学术观点;促进合作交流
左边点击“阅读原文”,跳转至原文链接
右边给我一朵小花花
以上是关于论文精选|基于深度神经网络的实时作业车间RFID订单完成时间预测的主要内容,如果未能解决你的问题,请参考以下文章
作业车间问题的调度学习:使用图神经网络(GNN)和强化学习(RL)的表示和策略学习