从未看过如此详细的深度学习推荐系统应用详解,读它!
Posted AI科技大本营
tags:
篇首语:本文由小常识网(cha138.com)小编为大家整理,主要介绍了从未看过如此详细的深度学习推荐系统应用详解,读它!相关的知识,希望对你有一定的参考价值。
作者丨gongyouliu
编辑丨zandy
来源 | 大数据与人工智能(ID:ai-big-data)
【导读】2016年DeepMind开发的AlphaGo在围棋对决中战胜了韩国九段选手李世石,一时成为轰动全球的重大新闻,被全球多家媒体大肆报道。AlphaGo之所以取得这么大的成功,这其中最重要的技术之一是深度学习技术。经过这几年的发展,深度学习技术已经在图像分类、语音识别、自然语言处理等领域取得突破性进展,甚至在某些方面(如图像分类等)超越了人类专家的水平。深度学习技术驱动了第三次人工智能浪潮的到来。
鉴于深度学习技术的巨大威力,它被学术界、产业界尝试应用于各类业务及应用场景,包括计算机视觉、语音识别、自然语言处理、搜索、推荐、广告等等。2016年YouTube发表论文将深度学习应用于视频推荐取得了非常好的效果,自此之后,深度学习技术在推荐系统上的应用遍地开花,各种论文、学术交流、产业应用层出不穷。国际著名的推荐系统会议RecSys从2016开始专门组织关于深度学习的会议,深度学习在推荐圈中越来越受到重视。
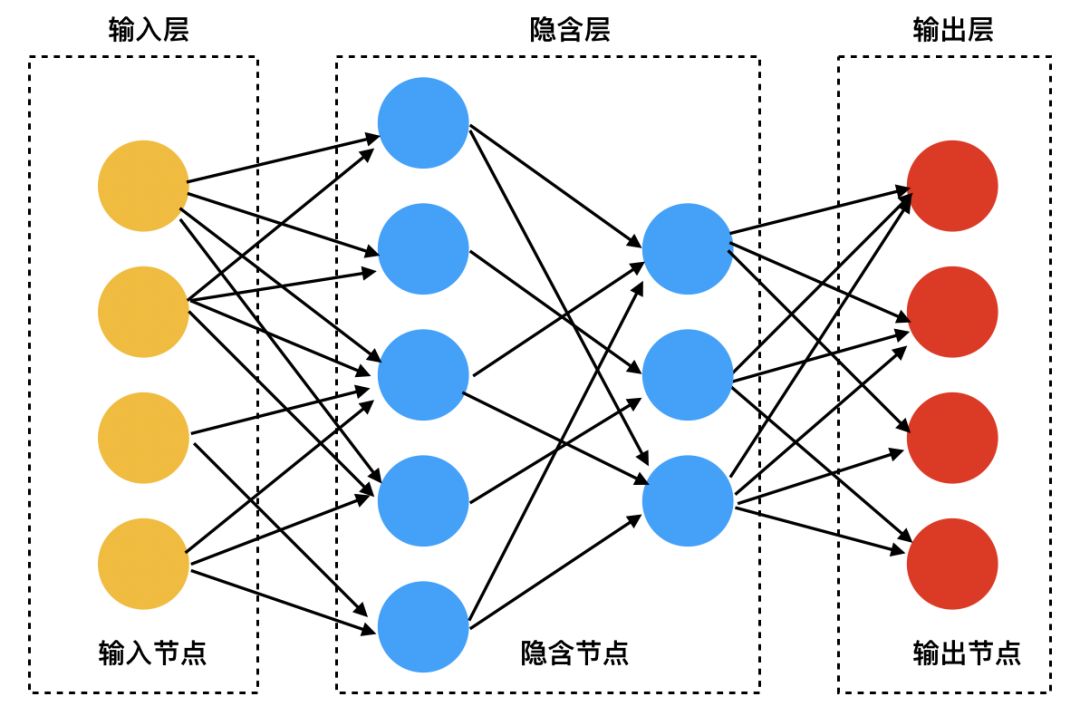












(1) 推荐作为评分预测问题
(3) 推荐作为分类问题
三、几种用于推荐系统的嵌入方法的算法原理介绍
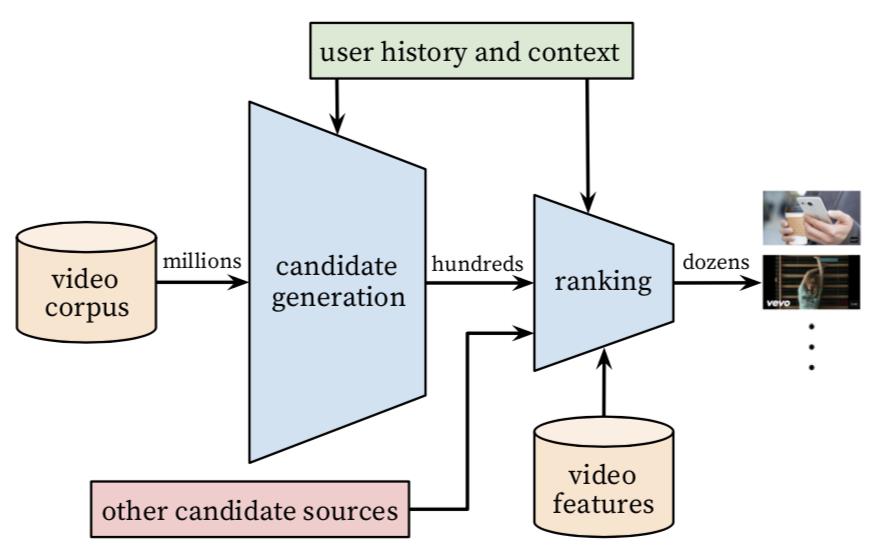
1. 候选集生成
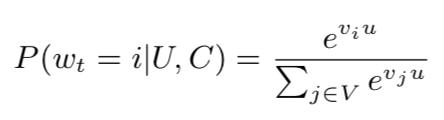
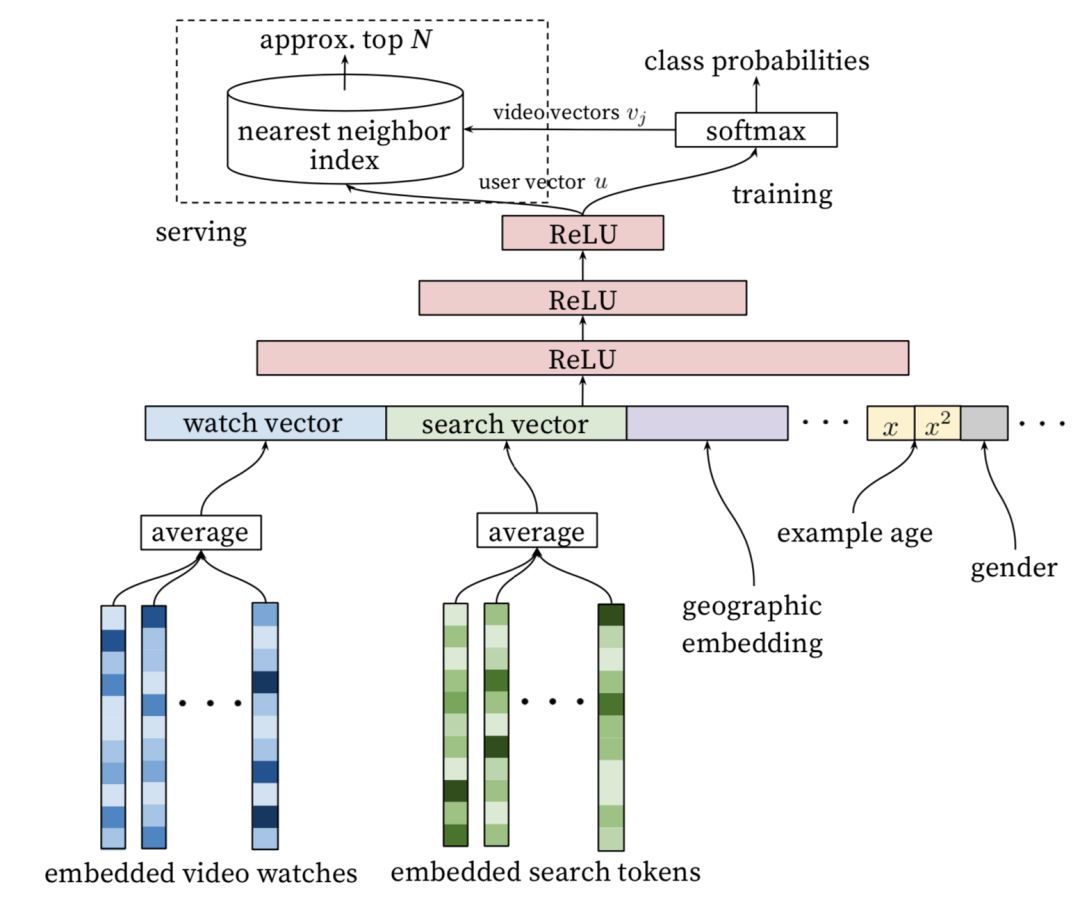



2. 候选集排序
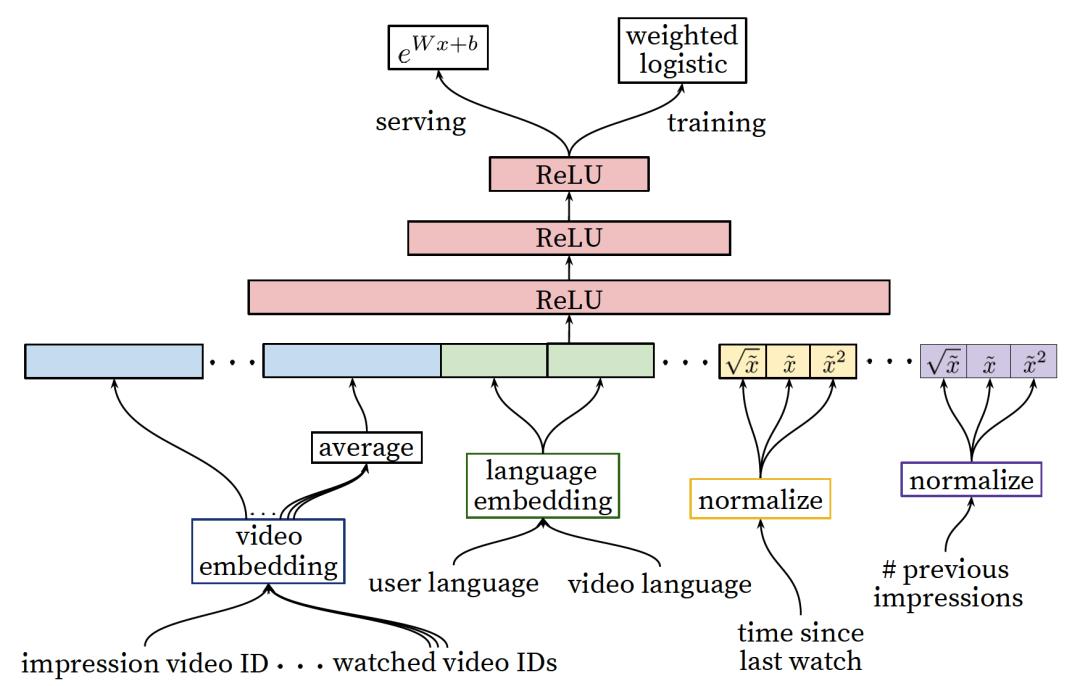


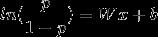
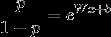




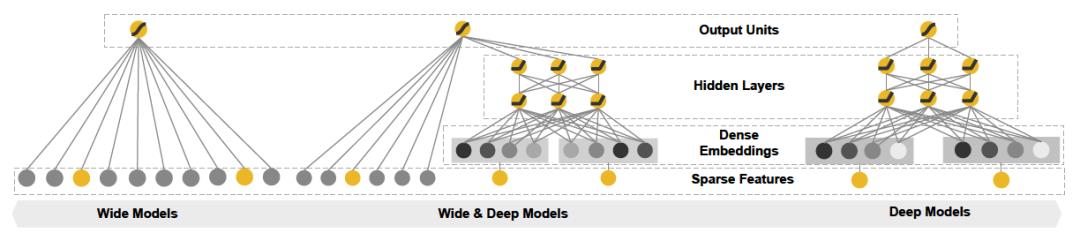




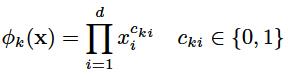



















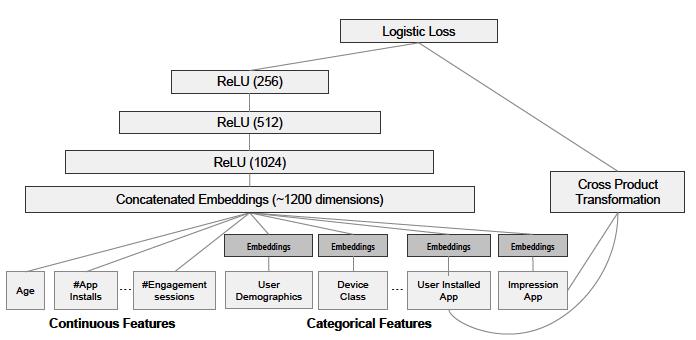
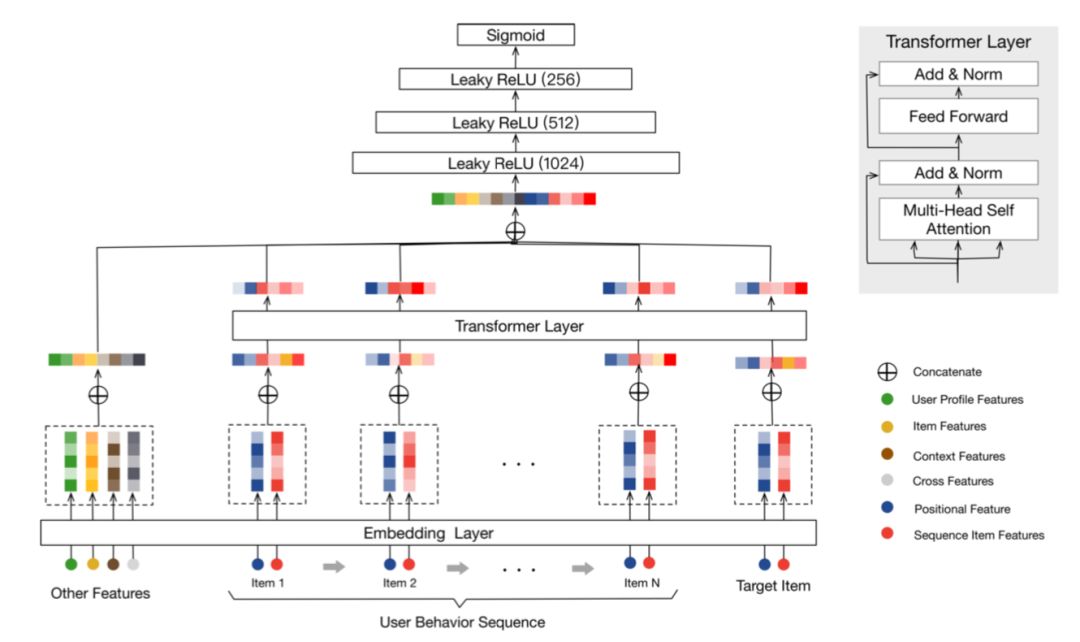
(1) 构建兴趣树
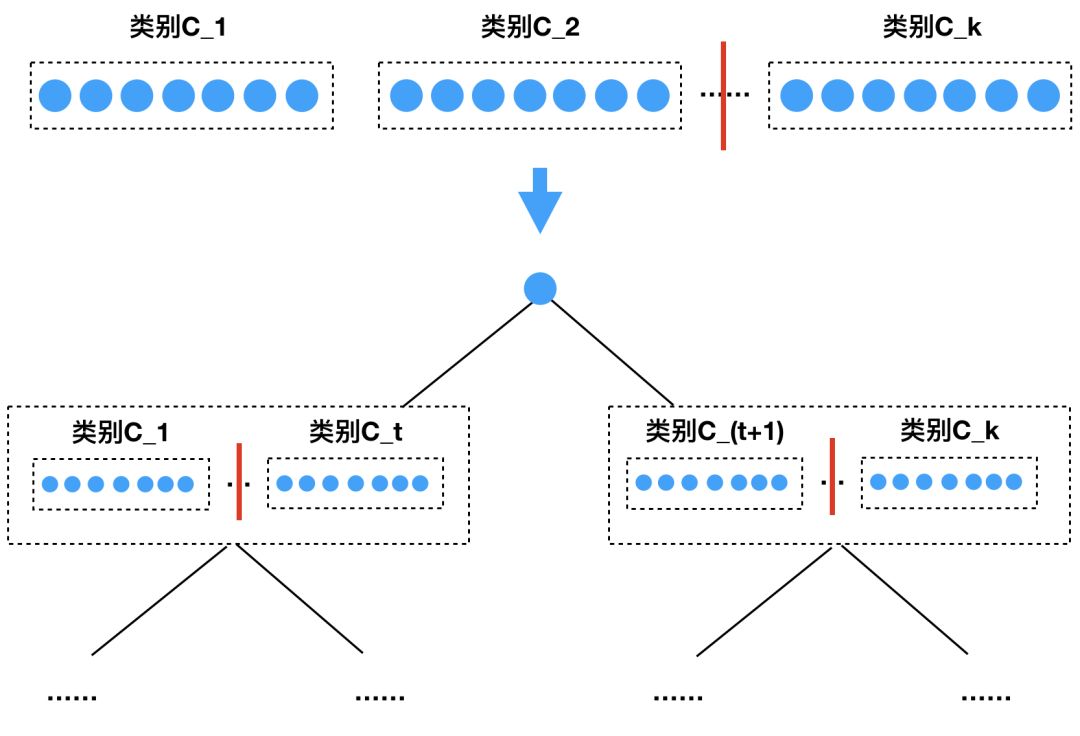
(2) 学习兴趣树叶子节点的嵌入表示










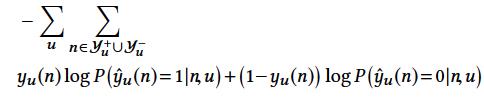
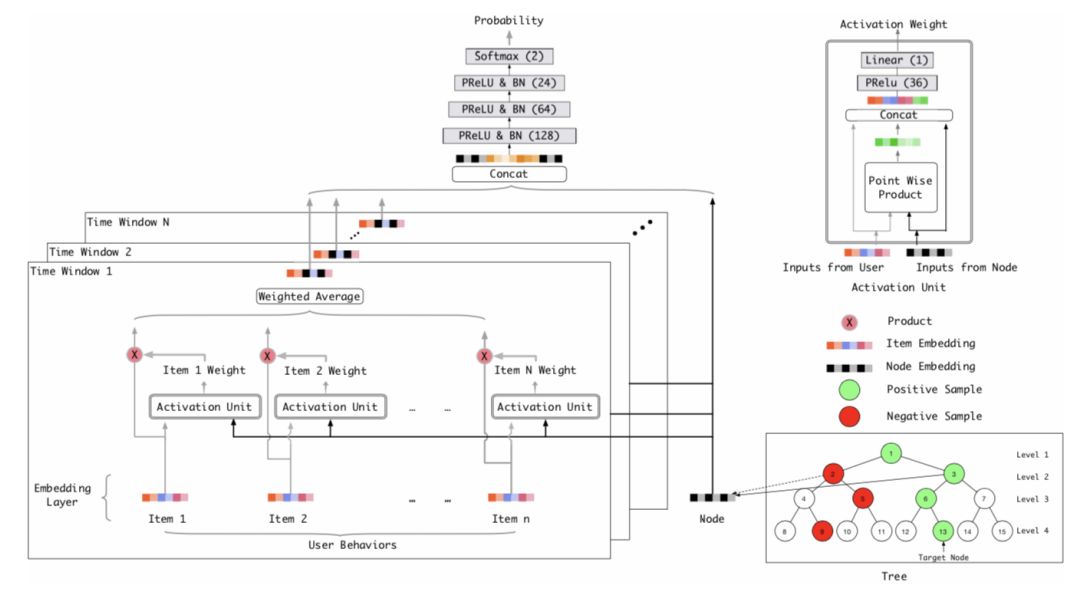
(3) 从树中检索出topN最喜欢的商品
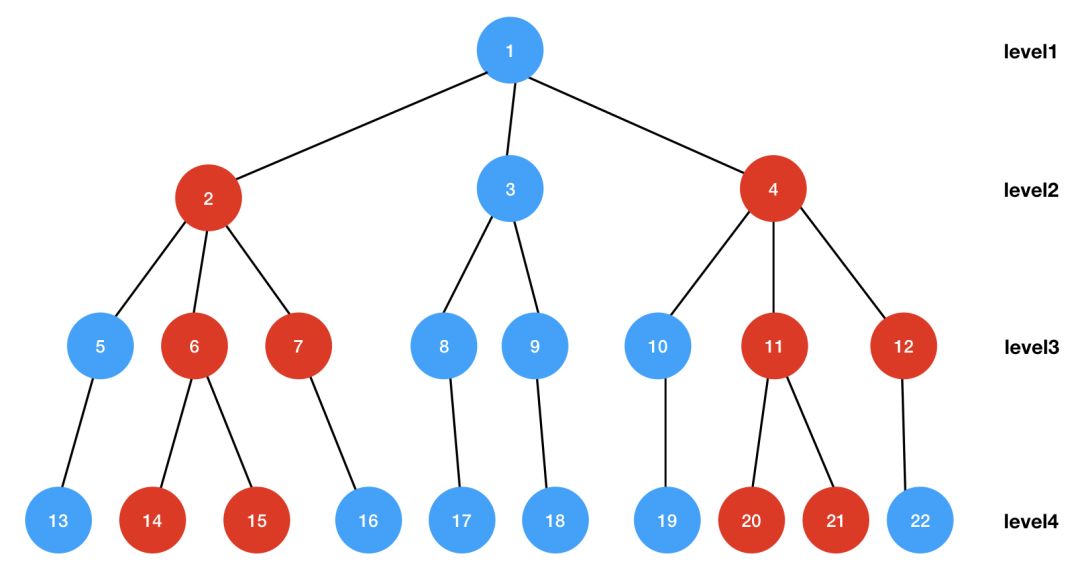
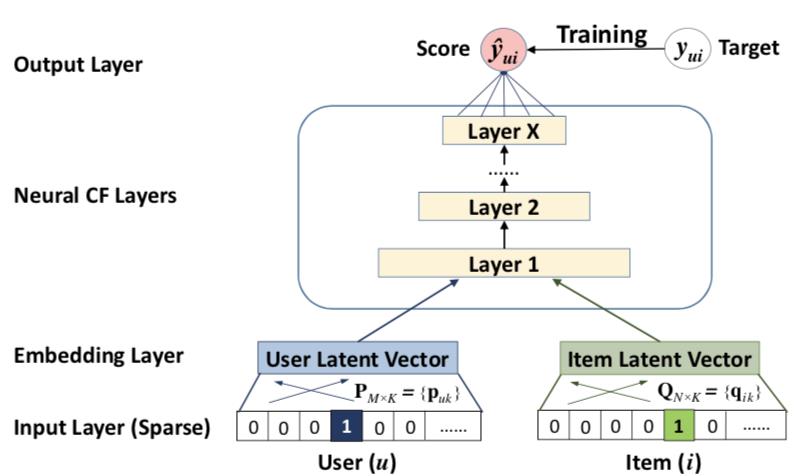






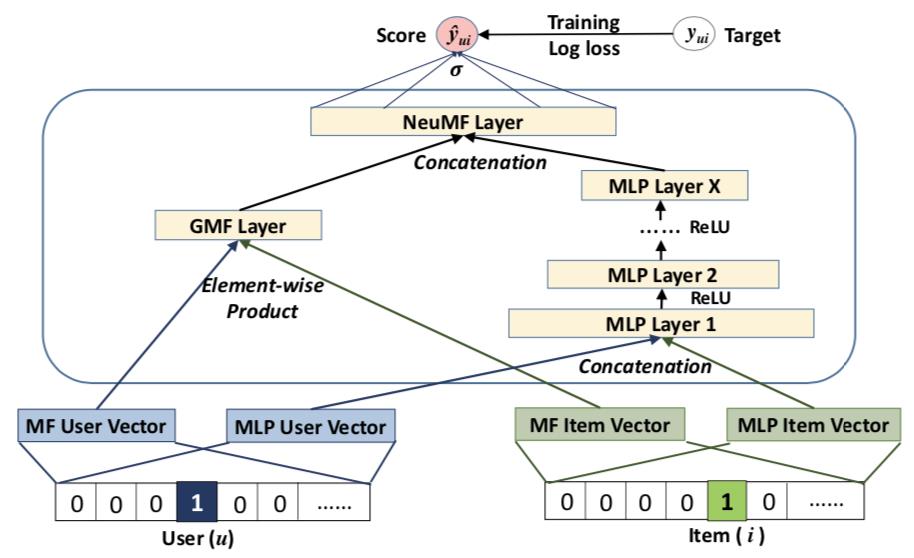
四、开源深度学习框架&推荐算法介绍
1.Tensorflow(Keras)
2.PyTorch(Caffe)
五、深度学习技术应用于推荐系统的优缺点及挑战
1. 优点
(1) 更加精准的推荐
(2) 可以减少人工特征工程的投入
(3) 可以方便整合附加信息(side information)
2. 缺点与挑战
(1) 需要大量的样本数据来训练可用的深度学习模型
(2) 需要大量的硬件资源进行训练
(3) 对技术要求相对较高,人才比较紧缺
(4) 跟团队现有的软件架构适配,工程实现有一定难度
(5) 深度学习模型可解释性不强
(6) 调参过程冗长复杂
六、深度学习推荐系统工程实施建议
深度学习的效果真的有那么好吗?
团队是否合适引入深度学习推荐技术
(1) 产品所在阶段及产品定位
(2) 是否有相关技术人员
(3) 深度学习相关硬件资源
(4) 其他的沉默成本
怎么打通深度技术相关技术栈与团队现有技术栈之间的脉络
从经典成熟的模型、从跟公司业务接近的模型开始尝试
七、深度学习推荐系统的未来发展
1. 算法模型维度
2. 工程维度
3. 应用场景维度
4. 数据维度
5. 产品呈现与交互维度
总结
参考文献
[1943] A Logical Calculus of Ideas Immanent in Nervous Activity
[2017] On the Origin of Deep Learning
[1986] Learning Representations by Back-Propagating
[1989] Multilayer feedforward networks are universal approximators
[深度学习-综述文章 2019] Deep Learning based Recommender System- A Survey and New Perspectives
[2007] Restricted Boltzmann Machines for Collaborative Filtering
[YouTube 2016] Deep Neural Networks for YouTube Recommendations
[Google 2016] Wide & Deep Learning for Recommender Systems
Deep content-based musicrecommendation
Improving Content-based and Hybrid Music Recommendation using Deep Learning
[2017 阿里] Deep Interest Network for Click-Through Rate Prediction
[2018 阿里] Deep Interest Evolution Network for Click-Through Rate Prediction
[2019 阿里] Behavior Sequence Transformer for E-commerce Recommendation in Alibaba
[2019 阿里] Deep Session Interest Network for Click-Through Rate Prediction
[2019 阿里] Multi-Interest Network with Dynamic Routing for Recommendation at Tmall
[2018 阿里] Learning Tree-based Deep Model for Recommender Systems
[网易] Personal Recommendation Using Deep Recurrent Neural Networks in NetEase
[2019 facebook] Deep Learning Recommendation Model for Personalization and Recommendation Systems
[2017 Google] Neural Collaborative Filtering
[2019 腾讯] Real-time Attention Based Look-alike Model for Recommender System
[2018 Airbnb] Applying Deep Learning To Airbnb Search
A Multi-View Deep Learning Approach for Cross Domain User Modeling in Recommendation Systems
[2015] Collaborative Deep Learning for Recommender Systems
[2015] Deep Collaborative Filtering via Marginalized Denoising Auto-encoder
[2016] A Neural Autoregressive Approach to Collaborative Filtering
[2016] Deep Learning over Multi-field Categorical Data – A Case Study on User Response Prediction
[2017 华为诺亚实验室] DeepFM- A Factorization-Machine based Neural Network for CTR Prediction
[2017 携程] A Hybrid Collaborative Filtering Model with Deep Structure for Recommender Systems
[2018] DKN- Deep Knowledge-Aware Network for News Recommendation
DeepPlaylist- Using Recurrent Neural Networks to Predict Song Similarity
The application of Deep Learning in Collaborative Filtering
[2016] Improved Recurrent Neural Networks for Session-based Recommendations
[2016] Session-based Recommendations with Recurrent Neural Networks
[2017] Contextual Sequence Modeling for Recommendation with Recurrent Neural Networks
[2017] Improving Session Recommendation with Recurrent Neural Networks by Exploiting Dwell Time
[2017] Inter-Session Modeling for Session-Based Recommendation
[2017] Personalizing Session-based Recommendations with Hierarchical Recurrent Neural Networks
[2017] Recurrent Latent Variable Networks for Session-BasedRecommendation
[2017] Recurrent Neural Networks with Top-k Gains for Session-based Recommendations
Collaborative Memory Network for Recommendation Systems
[2019 Google] Neural Input Search for Large Scale Recommendation Models
[2018 Google] Efficient Neural Architecture Search via Parameters Sharing
[2019] Are We Really Making Much Progress? A Worrying Analysis of Recent Neural Recommendation Approaches
[2017] Attention is all you need
[2018] Bert: Pre-training of deep bidirectional transformers for language understanding
[2018 京东] Deep Reinforcement Learning for List-wise Recommendations
[2019] TF-Ranking- Scalable TensorFlow Library for Learning-to-Rank
[2018 Pinterest] Graph Convolutional Neural Networks for Web-Scale Recommender Systems
(*本文为AI科技大本营转载文章,转载请联系原作者)
◆
精彩推荐
◆
开幕倒计时5天|2019 中国大数据技术大会(BDTC)即将震撼来袭!豪华主席阵容及百位技术专家齐聚,十余场精选专题技术和行业论坛,超强干货+技术剖析+行业实践立体解读。6.6 折票限时特惠(立减1400元)倒计时 2 天,学生票仅 599 元!
你点的每个“在看”,我都认真当成了AI
以上是关于从未看过如此详细的深度学习推荐系统应用详解,读它!的主要内容,如果未能解决你的问题,请参考以下文章