支持向量机原理及R语言实现
Posted Super越
tags:
篇首语:本文由小常识网(cha138.com)小编为大家整理,主要介绍了支持向量机原理及R语言实现相关的知识,希望对你有一定的参考价值。
简单的说可以理解为两个支持向量相连的中垂线。
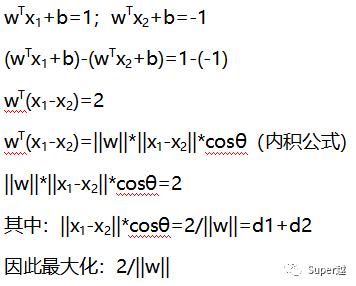
目标函数:minw,b (1/2*||w||2)
题外话:最优化问题
2、s.t.hi(x)=0 i=1,2,3....n
3、gi(x)≤0 i=1,2,3,4....n
回归正题:
第一步:求L(w,b,a)最小化。
第二步:对a 求极大值
目标函数:
核函数
【图片来源:网络】
支持向量机数学原理部分暂时讲到这里,如果想了解整个数学推导过程的可以去看一些机器学习的书籍,例如很多人推崇的西瓜书,李航的书籍讲得比较完整,也可以来问笔者,一起学习。若笔者上述原理中表达有错误的欢迎指出,下面是R语言实践部分。
R语言实现
pima.scale <- scale(pima[, 1:7]) %>% as_tibble() %>% bind_cols(type = pima$type)
pima.scale %>% melt(id.var = "type") %>% ggplot(aes(type, value)) + geom_boxplot(outlier.colour = "red") + facet_wrap(~variable,ncol =2)
pima.scale[,-8] %>% cor() %>% corrplot::corrplot.mixed()
检查样本数据分布是否均衡
table(pima.scale$type)
No Yes
355 177
按照三七分的方式划分训练集和测试集
set.seed(2020)
index <- sample(1:2,nrow(pima.scale),replace = TRUE,prob = c(0.7,0.3))
pima_train <- pima.scale[index==1,]
pima_text <- pima.scale[index==2,]
建模
svm(formula, data = NULL, ..., subset,na.action =na.omit, scale = TRUE)
svm(x, y = NULL, scale = TRUE, type = NULL,kernel ="radial", degree = 3,gamma = if (is.vector(x)) 1 else 1 / ncol(x),coef0 = 0, cost = 1, nu = 0.5,class.weights = NULL, cachesize = 40,tolerance = 0.001, epsilon = 0.1,shrinking = TRUE,cross = 0, probability = FALSE, fitted = TRUE,..., subset, na.action = na.omit)
tune.svm(x, y = NULL, data = NULL, degree = NULL, gamma = NULL, coef0 = NULL,cost = NULL, nu = NULL, class.weights = NULL, epsilon = NULL, ...)
best.svm(x, tunecontrol = tune.control(), ...)
线性核函数
#线性核函数
svm.linear <- tune.svm(type~.,data = pima_train,kernel="linear",cost=c(0.01,0.05,0.1,0.5,1,5,10))
summary(svm.linear)
Parameter tuning of ‘svm’:
- sampling method: 10-fold cross validation
- best parameters:
cost
0.01
- best performance: 0.2125178
- Detailed performance results:
cost error dispersion
1 0.01 0.2125178 0.07063750
2 0.05 0.2312945 0.07294707
3 0.10 0.2313656 0.08695672
4 0.50 0.2207681 0.08315716
5 1.00 0.2234708 0.08907731
6 5.00 0.2261024 0.08817271
7 10.00 0.2261024 0.08817271
svm.linear_pred <- predict(svm.linear$best.model,newdata = pima_text)
flag <- table(svm.linear_pred,pima_text$type) %>% print(.)
svm.linear_pred No Yes
No 95 27
Yes 7 26
#计算准确率
accu.svm.linear <- (sum(diag(flag))/nrow(pima_text)) %>% print(.)
[1] 0.7806452
svm.poly <- tune.svm(type~.,data = pima_train,kernel="polynomial",degree = c(2:5),coef0 = c(seq(0,3,by=0.5)))
summary(svm.poly)
Parameter tuning of ‘svm’:
- sampling method: 10-fold cross validation
- best parameters:
degree coef0
2 0.5
- best performance: 0.2386913
- Detailed performance results:
degree coef0 error dispersion
1 2 0.0 0.3128023 0.04506974
2 3 0.0 0.2492888 0.04119076
**************展示部分**************
27 4 3.0 0.2996444 0.07481871
28 5 3.0 0.3339972 0.07827219
svm.poly_pred <- predict(svm.poly$best.model,newdata = pima_text)
flag <- table(svm.poly_pred,pima_text$type) %>% print(.)
svm.poly_pred No Yes
No 94 29
Yes 8 24
计算准确率
accu.svm.poly <- (sum(diag(flag))/nrow(pima_text)) %>% print(.)
[1] 0.7612903
#径向基核函数
svm.radial <- tune.svm(type~.,data = pima_train,kernel="radial",gamma = c(seq(0.5,3,by=0.5)))
summary(svm.radial)
Parameter tuning of ‘svm’:
- sampling method: 10-fold cross validation
- best parameters:
gamma
0.5
- best performance: 0.283357
- Detailed performance results:
gamma error dispersion
1 0.5 0.2833570 0.08014833
2 1.0 0.3016358 0.10943430
3 1.5 0.3152205 0.09980037
4 2.0 0.3285917 0.11007829
5 2.5 0.3285917 0.10479007
6 3.0 0.3285917 0.10479007
svm.radial_pred <- predict(svm.radial$best.model,newdata = pima_text)
flag <- table(svm.radial_pred,pima_text$type) %>% print(.)
svm.radial_pred No Yes
No 93 30
Yes 9 23
> #计算准确率
> accu.svm.radial <- (sum(diag(flag))/nrow(pima_text)) %>% print(.)
[1] 0.7483871
svm.sigmoid <- tune.svm(type~.,data = pima_train,kernel="sigmoid",gamma = c(0.1,0.5,1,2,3),coef0 = c(0.1,0.5,1,2,3))
summary(svm.sigmoid)
Parameter tuning of ‘svm’:
- sampling method: 10-fold cross validation
- best parameters:
gamma coef0
0.1 2
- best performance: 0.2440256
- Detailed performance results:
gamma coef0 error dispersion
1 0.1 0.1 0.2466572 0.03934200
2 0.5 0.1 0.3020626 0.04304675
**************展示部分**************
24 2.0 3.0 0.3285917 0.08414808
25 3.0 3.0 0.3127312 0.06223051
svm.sigmoid_pred <- predict(svm.sigmoid$best.model,newdata = pima_text)
flag <- table(svm.sigmoid_pred,pima_text$type) %>% print(.)
svm.sigmoid_pred No Yes
No 93 24
Yes 9 29
#计算准确率
accu.svm.sigmoid <- (sum(diag(flag))/nrow(pima_text)) %>% print(.)
[1] 0.7870968
tibble(linear=accu.svm.linear,polynomial=accu.svm.poly,radial=accu.svm.radial,sigmoid=accu.svm.sigmoid) %>% print()
# A tibble: 1 x 4
linear polynomial radial sigmoid
<dbl> <dbl> <dbl> <dbl>
1 0.781 0.761 0.748 0.787
weights <- c(2.5,2)
names(weights) <- c("Yes","No")
model <- svm(type~.,data = pima_train,kernel="sigmoid",gamma=0.1,coef0=2,class.weights = weights)
pred <- predict(model, newdata = pima_text)
flag <- table(pred,pima_text$type) %>% print(.)
pred No Yes
No 93 22
Yes 9 31
#计算准确率
accu <- (sum(diag(flag))/nrow(pima_text)) %>% print(.)
[1] 0.8
R完整代码
#清除缓存
rm(list=ls())
gc()
#加载数据包
library(MASS)
library(dplyr)
library(reshape2)
library(ggplot2)
library(e1071)
#描述性分析
pima <- rbind(Pima.tr, Pima.te)
str(pima)
table(pima$type)
pima.scale <- scale(pima[, 1:7]) %>% as_tibble() %>% bind_cols(type = pima$type)
pima.scale %>% melt(id.var = "type") %>% ggplot(aes(type, value)) + geom_boxplot(outlier.colour = "red") + facet_wrap(~variable,ncol =2)
pima.scale[,-8] %>% cor() %>% corrplot::corrplot.mixed()
table(pima.scale$type)
#划分训练集和测试集
set.seed(2020)
index <- sample(1:2,nrow(pima.scale),replace = TRUE,prob = c(0.7,0.3))
pima_train <- pima.scale[index==1,]
pima_text <- pima.scale[index==2,]
dim(pima_train)
dim(pima_text)
#线性核函数
svm.linear <- tune.svm(type~.,data = pima_train,kernel="linear",cost=c(0.01,0.05,0.1,0.5,1,5,10))
summary(svm.linear)
svm.linear_pred <- predict(svm.linear$best.model,newdata = pima_text)
flag <- table(svm.linear_pred,pima_text$type) %>% print(.)
#计算准确率
accu.svm.linear <- (sum(diag(flag))/nrow(pima_text)) %>% print(.)
#多项式核函数
svm.poly <- tune.svm(type~.,data = pima_train,kernel="polynomial",degree = c(2:5),coef0 = c(seq(0,3,by=0.5)))
summary(svm.poly)
svm.poly_pred <- predict(svm.poly$best.model,newdata = pima_text)
flag <- table(svm.poly_pred,pima_text$type) %>% print(.)
#计算准确率
accu.svm.poly <- (sum(diag(flag))/nrow(pima_text)) %>% print(.)
#径向基核函数
svm.radial <- tune.svm(type~.,data = pima_train,kernel="radial",gamma = c(seq(0.5,3,by=0.5)))
summary(svm.radial)
svm.radial_pred <- predict(svm.radial$best.model,newdata = pima_text)
flag <- table(svm.radial_pred,pima_text$type) %>% print(.)
#计算准确率
accu.svm.radial <- (sum(diag(flag))/nrow(pima_text)) %>% print(.)
#S函数核函数
svm.sigmoid <- tune.svm(type~.,data = pima_train,kernel="sigmoid",gamma = c(0.1,0.5,1,2,3),coef0 = c(0.1,0.5,1,2,3))
summary(svm.sigmoid)
svm.sigmoid_pred <- predict(svm.sigmoid$best.model,newdata = pima_text)
flag <- table(svm.sigmoid_pred,pima_text$type) %>% print(.)
#计算准确率
accu.svm.sigmoid <- (sum(diag(flag))/nrow(pima_text)) %>% print(.)
#四种核函数准确率整合
tibble(linear=accu.svm.linear,polynomial=accu.svm.poly,radial=accu.svm.radial,sigmoid=accu.svm.sigmoid) %>% print()
#加入权重
weights <- c(2.5,2)
names(weights) <- c("Yes","No")
model <- svm(type~.,data = pima_train,kernel="sigmoid",gamma=0.1,coef0=2,class.weights = weights)
pred <- predict(model, newdata = pima_text)
flag <- table(pred,pima_text$type) %>% print(.)
#计算准确率
accu <- (sum(diag(flag))/nrow(pima_text)) %>% print(.)
感谢您的支持
以上是关于支持向量机原理及R语言实现的主要内容,如果未能解决你的问题,请参考以下文章