手把手教你使用Flask轻松部署机器学习模型(附代码&链接) | CSDN博文精选
Posted AI科技大本营
tags:
篇首语:本文由小常识网(cha138.com)小编为大家整理,主要介绍了手把手教你使用Flask轻松部署机器学习模型(附代码&链接) | CSDN博文精选相关的知识,希望对你有一定的参考价值。
作者 | Abhinav Sagar
翻译 | 申利彬
校对 | 吴金笛
来源 | 数据派THU(ID:DatapiTHU)

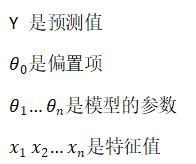
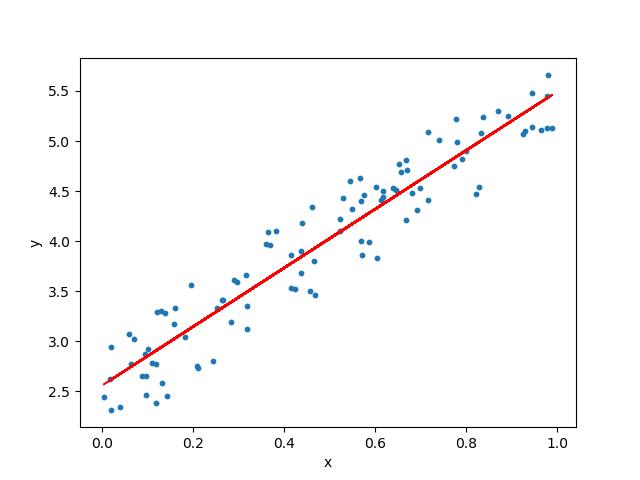
-
容易上手使用 -
内置开发工具和调试工具 -
集成单元测试功能 -
平稳的请求调度 -
详尽的文档
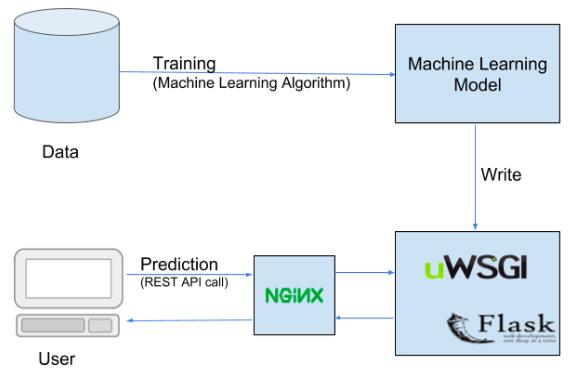
<!DOCTYPE html>
<html ><head>
<meta charset="UTF-8">
<title>Deployment Tutorial 1</title>
<link href='https://fonts.googleapis.com/css?family=Pacifico' rel='stylesheet' type='text/css'>
<link href='https://fonts.googleapis.com/css?family=Arimo' rel='stylesheet' type='text/css'>
<link href='https://fonts.googleapis.com/css?family=Hind:300' rel='stylesheet' type='text/css'>
<link href='https://fonts.googleapis.com/css?family=Open+Sans+Condensed:300' rel='stylesheet' type='text/css'>
<link rel="stylesheet" href="{{ url_for('static', filename='css/style.css') }}"></head><body style="background: #000;">
<div><h1>Sales Forecasting
</h1>
<!-- Main Input For Receiving Query to our ML -->
<form action="{{ url_for('predict')}}"method="post">
<input type="text" name="rate" placeholder="rate" required="required" />
<input type="text" name="sales in first month" placeholder="sales in first month" required="required" />
<input type="text" name="sales in second month" placeholder="sales in second month" required="required" />
<button type="submit" class="btn btn-primary btn-block btn-large">Predict sales in third month</button>
</form>
<br>
<br> {{ prediction_text }}
</div>
</body>
</html>
@import url(https://fonts.googleapis.com/css?family=Open+Sans);
html { width: 100%; height:100%; overflow:hidden;
}body {width: 100%;height:100%;font-family: 'Helvetica';background: #000;
color: #fff;
font-size: 24px;
text-align:center;
letter-spacing:1.4px;}.login {position: absolute;
top: 40%;
left: 50%;
margin: -150px 0 0 -150px;
width:400px;
height:400px;}
login h1 { color: #fff;
text-shadow: 0 0 10px rgba(0,0,0,0.3);
letter-spacing:1px;
text-align:center;
}input {width: 100%;
margin-bottom: 10px;
background: rgba(0,0,0,0.3);
border: none;
outline: none;
padding: 10px;
font-size: 13px;
color: #fff;
text-shadow: 1px 1px 1px rgba(0,0,0,0.3);
border: 1px solid rgba(0,0,0,0.3);
border-radius: 4px;
box-shadow: inset 0 -5px 45px rgba(100,100,100,0.2), 0 1px 1px rgba(255,255,255,0.2);
-webkit-transition: box-shadow .5s ease;
-moz-transition: box-shadow .5s ease;
-o-transition: box-shadow .5s ease;
-ms-transition: box-shadow .5s ease;
transition: box-shadow .5s ease;
}
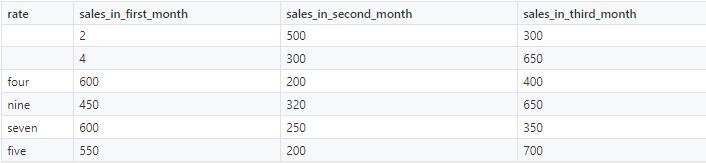
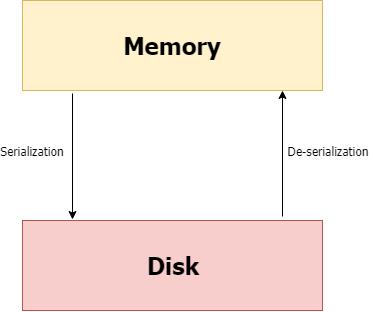
import numpy as np
import matplotlib.pyplot as plt
import pandas as pd
import pickle
dataset = pd.read_csv('sales.csv')
dataset['rate'].fillna(0, inplace=True)
dataset['sales_in_first_month'].fillna(dataset['sales_in_first_month'].mean(), inplace=True)
X = dataset.iloc[:, :3]
def convert_to_int(word):
word_dict = {'one':1, 'two':2, 'three':3, 'four':4, 'five':5, 'six':6, 'seven':7, 'eight':8,
'nine':9, 'ten':10, 'eleven':11, 'twelve':12, 'zero':0, 0: 0}
return word_dict[word]
X['rate'] = X['rate'].apply(lambda x : convert_to_int(x))
y = dataset.iloc[:, -1]
from sklearn.linear_model import LinearRegression
regressor = LinearRegression()
regressor.fit(X, y)
pickle.dump(regressor, open('model.pkl','wb'))
model = pickle.load(open('model.pkl','rb'))
print(model.predict([[4, 300, 500]]))
import numpy as np
from flask import Flask, request, jsonify, render_template
import pickle
app = Flask(__name__)model = pickle.load(open('model.pkl', 'rb'))
@app.route('/')
def home():
return render_template('index.html')
@app.route('/predict',methods=['POST'])
def predict():
int_features = [int(x) for x in request.form.values()]
final_features = [np.array(int_features)]
prediction = model.predict(final_features)
output = round(prediction[0], 2)
return render_template('index.html', prediction_text='Sales should
be $ {}'.format(output))
@app.route('/results',methods=['POST'])
def results():
data = request.get_json(force=True)
prediction = model.predict([np.array(list(data.values()))])
output = prediction[0]
return jsonify(output)
if __name__ == "__main__":
app.run(debug=True)
import requests
url = 'http://localhost:5000/results'
r = requests.post(url,json={'rate':5,
'sales_in_first_month':200, 'sales_in_second_month':400})
print(r.json()) Results
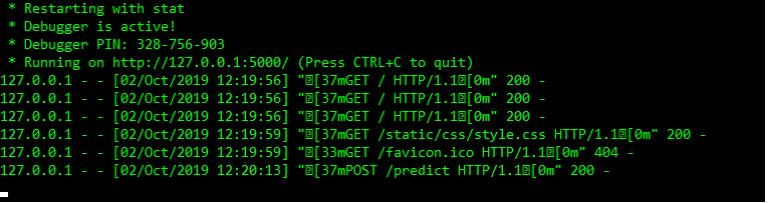
扫码查看原文
▼▼▼
(*本文为AI科技大本营转载文章,转载请联系原作者)
◆
精彩推荐
◆
推荐阅读
你点的每个“在看”,我都认真当成了AI
以上是关于手把手教你使用Flask轻松部署机器学习模型(附代码&链接) | CSDN博文精选的主要内容,如果未能解决你的问题,请参考以下文章