如何用Keras自定义层?
Posted
tags:
篇首语:本文由小常识网(cha138.com)小编为大家整理,主要介绍了如何用Keras自定义层?相关的知识,希望对你有一定的参考价值。
我用keras搭建的模型 想在里面增加一层 用来提取每张图片上像素的最大值和最小值 作为传统特征 与神经网络提取的深度特征进行特征融合 目前我是想用keras提供的lambda层来实现 但是lambda层的输入是一个(batch,224,224,3) 4维向量 我应该怎么从这个4维向量中提取出每张图片的传统特征? 如何定义lambda里面的功能函数?
new_layer2=Lambda(spatial_min, x : x)(input) 参考技术A 回答
您好根据您的描述,答案是如下所示:Keras层的骨架。只需要实现三个方法即可:build(input_shape): 这是你定义权重的地方。这个方法必须设self.built = True,可以通过调用super([Layer], self).build()完成。build,这个函数用来确立这个层都有哪些参数,哪些参数是可训练的哪些参数是不可训练的。call(x): 这里是编写层的功能逻辑的地方。你只需要关注传入call的第一个参数:输入张量,除非你希望你的层支持masking。这个函数在调用层对象时自动使用,里面就是该层的计算逻辑,或计算图了。显然,这个层的核心应该是一段符号式的输入张量到输出张量的计算过程。compute_output_shape(input_shape): 如果你的层更改了输入张量的形状,你应该在这里定义形状变化的逻辑,这让Keras能够自动推断各层的形状。
提问你好,我想知道的是keras模型的自定义层的名称如何在结构上获取?
回答使用了自定义的层,如Lambda()# 文本相似度评估方式def exponent_neg_manhattan_distance(sent_left, sent_middle, sent_right): \'\'\'基于曼哈顿空间距离计算两个字符串语义空间表示相似度计算\'\'\' return ((K.exp(-K.sum(K.abs(sent_left - sent_middle), axis=1, keepdims=True)) - K.exp(-K.sum(K.abs(sent_left - sent_right), axis=1, keepdims=True))) + 1) / 2def bilstm_siamese_model():‘’‘搭建孪生网络’’’#可以在这里调参embedding_layer = Embedding(VOCAB_SIZE + 1,EMBEDDING_DIM,weights=[embedding_matrix],input_length=MAX_LENGTH,trainable=True, #原本为Falsemask_zero=True)
输入层left_input = Input(shape=(MAX_LENGTH,), dtype=‘float32’, name=“left_x”) #(?, 25)middle_input = Input(shape=(MAX_LENGTH,), dtype=‘float32’, name=“middle_x”) #(?, 25)right_input = Input(shape=(MAX_LENGTH,), dtype=‘float32’, name=‘right_x’)#嵌入层encoded_left = embedding_layer(left_input) #(?, 25, 300)encoded_middle = embedding_layer(middle_input) #(?, 25, 300)encoded_right = embedding_layer(right_input)
提问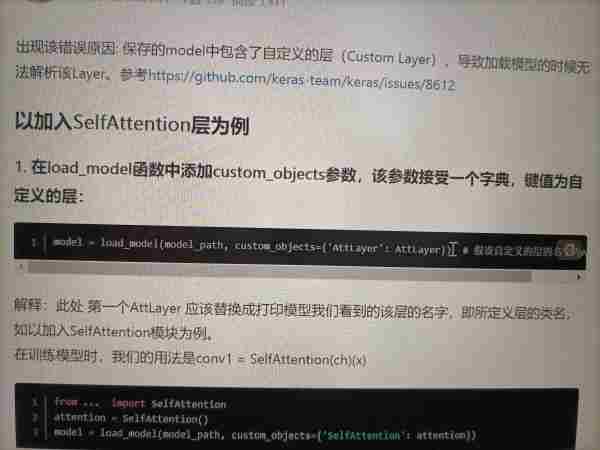
我的意思是从模型结构上得知其自定义层的名称
回答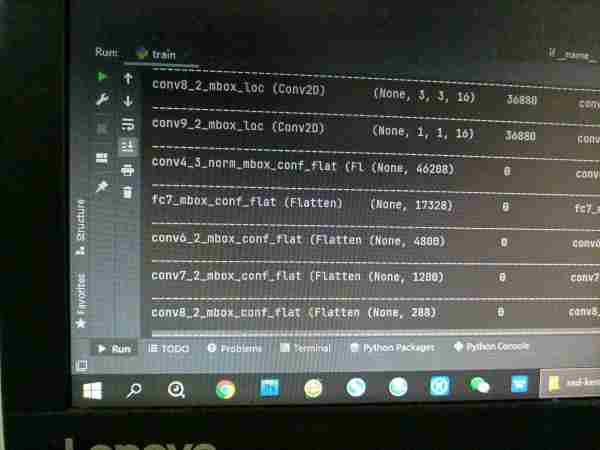
如何在 Keras 中实现具有动态形状的自定义输出层?
【中文标题】如何在 Keras 中实现具有动态形状的自定义输出层?【英文标题】:How to implement custom output layer with dynamic shape in Keras? 【发布时间】:2020-11-06 07:43:05 【问题描述】:我想用 Tensorflow 2.0 后端在 Keras 中实现 YOLO-tiny。我想制作一个新的自定义 YoloLayer,它对前一层的输出执行非最大抑制,并制作形状为 (batch_size, num, 6)
的张量,其中 num
是找到的多个预测,每个预测显示为 [x, y, w, h, prob, class]
。我还在__init__()
方法中设置了self.trainable = False
。这是我的call
方法:
def call(self, inputs, **kwargs):
predictions = inputs[...,:5]
x = tf.math.add(self.cols, tf.nn.sigmoid(predictions[...,0])) / self.grid_size # x
y = tf.math.add(self.rows, tf.nn.sigmoid(predictions[...,1])) / self.grid_size # y
w = tf.multiply(self.anchors_w, tf.math.exp(predictions[...,2])) / self.grid_size # w
h = tf.multiply(self.anchors_h, tf.math.exp(predictions[...,3])) / self.grid_size # h
c = tf.nn.sigmoid(predictions[...,4]) # confidence
bounds = tf.stack([x, y, w, h], -1)
classes = inputs[...,5:]
probs = tf.multiply(tf.nn.softmax(classes), tf.expand_dims(c, axis=-1))
prob_mask = tf.greater(probs, self.threshold)
suppressed_indices = tf.where(prob_mask)
suppressed_probs = tf.gather_nd(probs, suppressed_indices[...,:3])
suppressed_boxes = tf.gather_nd(bounds, suppressed_indices[...,:3])
box_coords = tf.stack([
suppressed_boxes[...,1] - suppressed_boxes[...,3] / 2., #y1
suppressed_boxes[...,0] - suppressed_boxes[...,2] / 2., #x1
suppressed_boxes[...,1] + suppressed_boxes[...,3] / 2., #y2
suppressed_boxes[...,0] + suppressed_boxes[...,2] / 2., #x2
], axis=-1)
out = tf.TensorArray(tf.float32, size=0, dynamic_size=True)
for i in range(tf.shape(inputs)[0]):
image_out = tf.TensorArray(tf.float32, size=self.classes)
for c in range(self.classes):
class_probs = suppressed_probs[i,:,c]
indices = tf.image.non_max_suppression(box_coords[i], class_probs, 10,
iou_threshold=self.nms_threshold,
score_threshold=self.threshold)
if tf.size(indices) > 0:
final_probs = tf.expand_dims(tf.gather(class_probs, indices), axis=-1)
final_boxes = tf.gather(suppressed_boxes[i], indices)
class_vec = tf.ones((tf.shape(final_probs)[0], 1)) * c
image_out.write(c, tf.concat([final_boxes, final_probs, class_vec], axis=1))
image_out = image_out.concat()
out.write(i, image_out)
out = out.stack()
return out
然后,model.summary()
返回:
Model: "sequential_1"
_________________________________________________________________
Layer (type) Output Shape Param #
=================================================================
...
_________________________________________________________________
yolo_layer (YoloLayer) (None, None, 6) 0
=================================================================
...
我为此模型加载了预训练的权重并运行model.predict
,但输出给了我一个错误:
InvalidArgumentError: Tried to stack elements of an empty list with non-fully-defined element_shape: [?,6]
[[node sequential_1/yolo_layer/TensorArrayV2Stack/TensorListStack (defined at <ipython-input-2-fbae137dd1a2>:96) ]] [Op:__inference_predict_function_4604]
我还在没有 YoloLayer 的情况下运行了这个模型,并使用相同的功能但单独修改了它的输出,它工作正常,但它没有使用占位符。我应该怎么做才能做到这一点?
【问题讨论】:
【参考方案1】:好的,我自己发现的。所要做的就是:
outputs = outputs.write(out_idx, image_out)
【讨论】:
以上是关于如何用Keras自定义层?的主要内容,如果未能解决你的问题,请参考以下文章
如何为层中的每个节点为 Keras relu 函数分配自定义 alpha?