文献速递 | 基于内窥镜图像的卷积神经网络在幽门螺杆菌感染诊断中的应用
Posted 达达科研圈
tags:
篇首语:本文由小常识网(cha138.com)小编为大家整理,主要介绍了文献速递 | 基于内窥镜图像的卷积神经网络在幽门螺杆菌感染诊断中的应用相关的知识,希望对你有一定的参考价值。
文章题目:Application of Convolutional Neural Networks in the Diagnosis of Helicobacter pylori Infection Based on Endoscopic Images
研究人员:Satoki Shichijo, et al.
发表时间:2017.11
期刊名称:EBioMedicine
影响因子:5.736
【1】核心亮点
1. 为了评估CNN在基于内窥镜图像识别幽门螺杆菌感染方面的作用,我们构建了一个基于人工智能的诊断系统,该系统使用30000张内窥镜图像进行训练。
2. 为了评估所构建的CNN的诊断准确性,并与内窥镜医师进行比较,我们准备了一个单独的测试数据集。
【2】思路与方法
A 22-layer, deep CNN was pre-trained and fine-tuned on a dataset of 32,208 images either positive or negative for H. pylori (first CNN). Another CNN was trained using images classified according to 8 anatomical locations (secondary CNN). A separate test data set (11,481 images from 397 patients) was evaluated by the CNN, and 23 endoscopists, independently.
一个22层的深层CNN被预先训练并微调在一个32208张幽门螺杆菌阳性或阴性图像的数据集上(第一个CNN)。另一个CNN使用根据8个解剖位置分类的图像进行训练(次级CNN)。CNN和23名内窥镜医师独立评估了一个单独的测试数据集(来自397名患者的11481张图像)。
【3】摘要
The role of artificial intelligence in the diagnosis of Helicobacter pylori gastritis based on endoscopic images has not been evaluated. We constructed a convolutional neural network (CNN), and evaluated its ability to diagnose H. pylori infection.The sensitivity, specificity, accuracy, and diagnostic time were 81.9%, 83.4%, 83.1%, and 198s, respectively, for the first CNN, and 88.9%, 87.4%, 87.7%, and 194s, respectively, for the secondary CNN. These values for the 23 endoscopists were 79.0%, 83.2%, 82.4%, and 230±65min (85.2%, 89.3%, 88.6%, and 253±92min by 6 board-certified endoscopists), respectively. The secondary CNN had a significantly higher accuracy than endoscopists (by 5.3%; 95% CI, 0.3-10.2).
人工智能在基于内镜图像诊断幽门螺杆菌性胃炎中的作用尚未评估。我们构建了一个卷积神经网络(CNN),并对其诊断幽门螺杆菌的能力进行了评估传染病第一次CNN的敏感性、特异性、准确性和诊断时间分别为81.9%、83.4%、83.1%和198s,第二次CNN的敏感性、特异性、准确性和诊断时间分别为88.9%、87.4%、87.7%和194s。23名内窥镜医师的这些值分别为79.0%、83.2%、82.4%和230±65min(6名委员会认证的内窥镜医师分别为85.2%、89.3%、88.6%和253±92min)。二级CNN的准确率明显高于内窥镜医师(5.3%;95%CI,0.3-10.2)。
【4】图表
Fig.1 Representative endoscopic images of Helicobacter pylori-positive, and –negative stomach
图1 幽门螺杆菌阳性和阴性胃的典型内镜图像
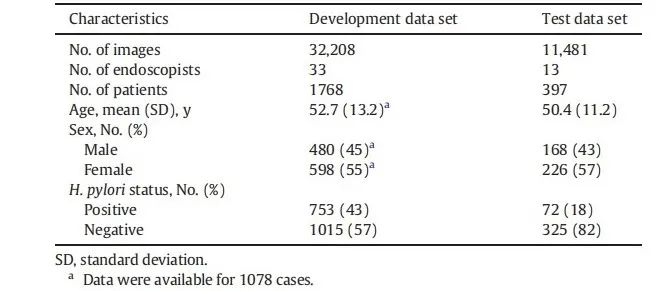
Tabel.1 Baseline characteristics
表1 基线特征
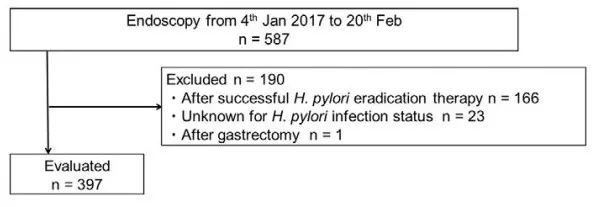
Fig.2 Patient recruitment flflowchart.
图2 患者招募流程图

Table.2 Diagnostic accuracy: CNN vs. endoscopists
表2 诊断准确性:CNN与内镜医师
Fig.3 Receiver operating curves for CNN trained by uncategorized data and prediction of the endoscopists
图3 未分类数据训练的CNN受试者工作曲线与内窥镜医师的预测
Fig.4 Receiver operating curves for CNN trained by categorized data.
图4 分类数据训练的CNN受试者工作曲线
【5】不足
1. 在胃窦部有轻微改变的胃中,基于正常胃体粘膜的诊断可能导致误诊。
2. 研究包括由鼻内窥镜获得的存档图像,这些图像与经口内窥镜或实时成像获得的图像相比信息较少。
【6】启发
1. 较短的筛查时间,以及CNN无疲劳,可能使内镜检查后立即提供结果。
2. 研究结果表明,基于CNN的筛查系统具有足够的敏感性和特异性,可以应用于临床,并有可能显著减少内镜医师的工作量。
参考文献
Application of Convolutional Neural Networks in the Diagnosis of Helicobacter pylori Infection Based on Endoscopic Images, EBioMedicine, Satoki Shichijo, et al, 2017 Nov;25:106-111.doi: 10.1016
采编:杨航 审核:谢茜荣
以上是关于文献速递 | 基于内窥镜图像的卷积神经网络在幽门螺杆菌感染诊断中的应用的主要内容,如果未能解决你的问题,请参考以下文章
文献阅读:基于双中心迁移学习的卷积神经网络在乳腺癌DCE-MRI分子亚型预测中的应用
文献阅读:基于双中心迁移学习的卷积神经网络在乳腺癌DCE-MRI分子亚型预测中的应用
文献阅读:基于双中心迁移学习的卷积神经网络在乳腺癌DCE-MRI分子亚型预测中的应用