学习笔记——sklearn监督学习:回归(简单数学知识罗列)
Posted 别呀
tags:
篇首语:本文由小常识网(cha138.com)小编为大家整理,主要介绍了学习笔记——sklearn监督学习:回归(简单数学知识罗列)相关的知识,希望对你有一定的参考价值。
文章目录
一、数学优化
损失函数
损失函数(Loss function)是用来估量你模型的预测值与真实值的不一致程度,它是一个非负实值函数,通常用。损失函数越小,模型的鲁棒性就越好。损失函数是经验风险函数的核心部分,也是结构风险函数的重要组成部分。模型的风险结构包括了风险项和正则项,通常如下所示:
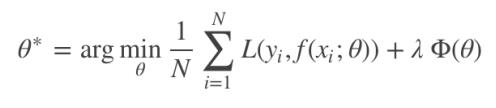
其中,前面的均值函数表示的是经验风险函数,L 代表的是损失函数,后面的 Φ是正则化项(regularizer)或者叫惩罚项(penalty term),它可以是 L1,也可以是L2,或者其他的正则函数。整个式子表示的意思是找到使目标函数最小时的θ值。
常见的损失误差有五种:
1. 0-1 损失(黑色)
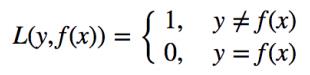
3. 铰链损失(Hinge Loss):主要用于支持向量机(SVM) 中
4. 互熵损失 (Cross Entropy Loss,Softmax Loss):用于 Logistic 回归与 Softmax 分类中(红色);

5. 平方损失(Square Loss):主要是最小二乘法(OLS)中(略);

6. 指数损失(Exponential Loss) :主要用于 Adaboost 算法中(蓝色);
SoftMax 损失
逻辑回归并没有求似然函数的极值,而是把极大化当做是一种思想,进而推导出它的经验风险函数为:最小化负的似然函数(即axF(y,f(x))→min−F(y,f(x)))maxF(y,f(x))→min−F(y,f(x)))。从损失函数的视角来看,它就成了 Softmax 损失函数了。
log 损失函数的标准形式:

利用已知的样本分布,找到最有可能(即最大概率)导致这种分布的参数值;或者说什么样的参数才能使我们观测到目前这组数据的概率最大。
二、回归分析
考虑广义线性模型:

其中 X 表示样本集,W 为待拟合的参数

1、最小二乘回归
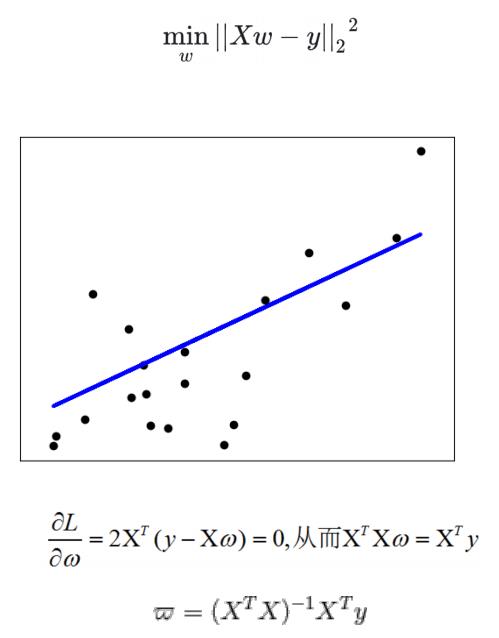
2、岭回归
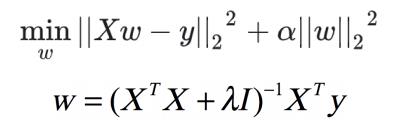
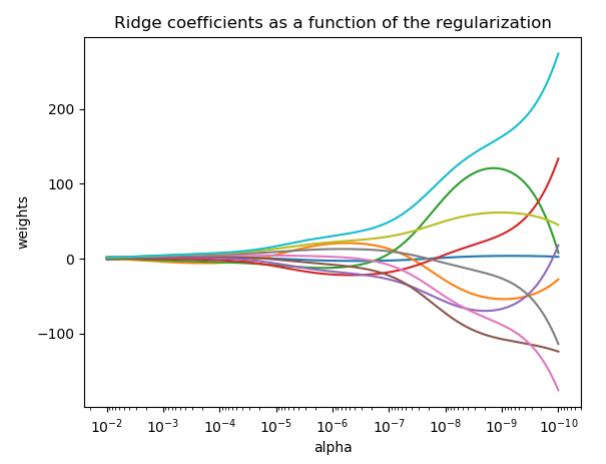
3、Logistics 回归

4、Lasso 回归

三、实例
1、Linear Regression
import matplotlib.pyplot as plt
import numpy as np
from sklearn import datasets, linear_model
from sklearn.metrics import mean_squared_error, r2_score
# Load the diabetes dataset
diabetes = datasets.load_diabetes()
# Use only one feature
diabetes_X = diabetes.data[:, np.newaxis, 2]
# Split the data into training/testing sets
diabetes_X_train = diabetes_X[:-20]
diabetes_X_test = diabetes_X[-20:]
# Split the targets into training/testing sets
diabetes_y_train = diabetes.target[:-20]
diabetes_y_test = diabetes.target[-20:]
# Create linear regression object
regr = linear_model.LinearRegression()
# Train the model using the training sets
regr.fit(diabetes_X_train, diabetes_y_train)
# Make predictions using the testing set
diabetes_y_pred = regr.predict(diabetes_X_test)
# The coefficients
print('Coefficients: \\n', regr.coef_)
# The mean squared error
print("Mean squared error: %.2f"
% mean_squared_error(diabetes_y_test, diabetes_y_pred))
# Explained variance score: 1 is perfect prediction
print('Variance score: %.2f' % r2_score(diabetes_y_test, diabetes_y_pred))
# Plot outputs
plt.scatter(diabetes_X_test, diabetes_y_test, color='black')
plt.plot(diabetes_X_test, diabetes_y_pred, color='blue', linewidth=3)
plt.xticks(())
plt.yticks(())
plt.show()
结果:
('Coefficients: \\n', array([938.23786125]))
Mean squared error: 2548.07
Variance score: 0.47
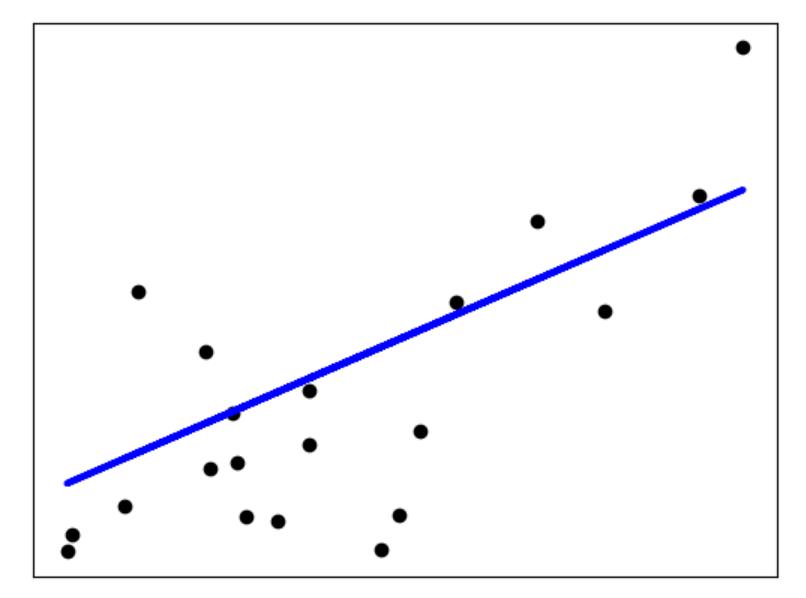
2、Ridge Regression
import numpy as np
import matplotlib.pyplot as plt
from sklearn import linear_model
# X is the 10x10 Hilbert matrix
X = 1. / (np.arange(1, 11) + np.arange(0, 10)[:, np.newaxis])
y = np.ones(10)
# #####################################################################
# Compute paths
n_alphas = 200
alphas = np.logspace(-10, -2, n_alphas)
coefs = []
for a in alphas:
ridge = linear_model.Ridge(alpha=a, fit_intercept=False)
ridge.fit(X, y)
coefs.append(ridge.coef_)
# ####################################################################
# Display results
ax = plt.gca()
ax.plot(alphas, coefs)
ax.set_xscale('log')
ax.set_xlim(ax.get_xlim()[::-1]) # reverse axis
plt.xlabel('alpha')
plt.ylabel('weights')
plt.title('Ridge coefficients as a function of the regularization')
plt.axis('tight')
plt.show()
结果:
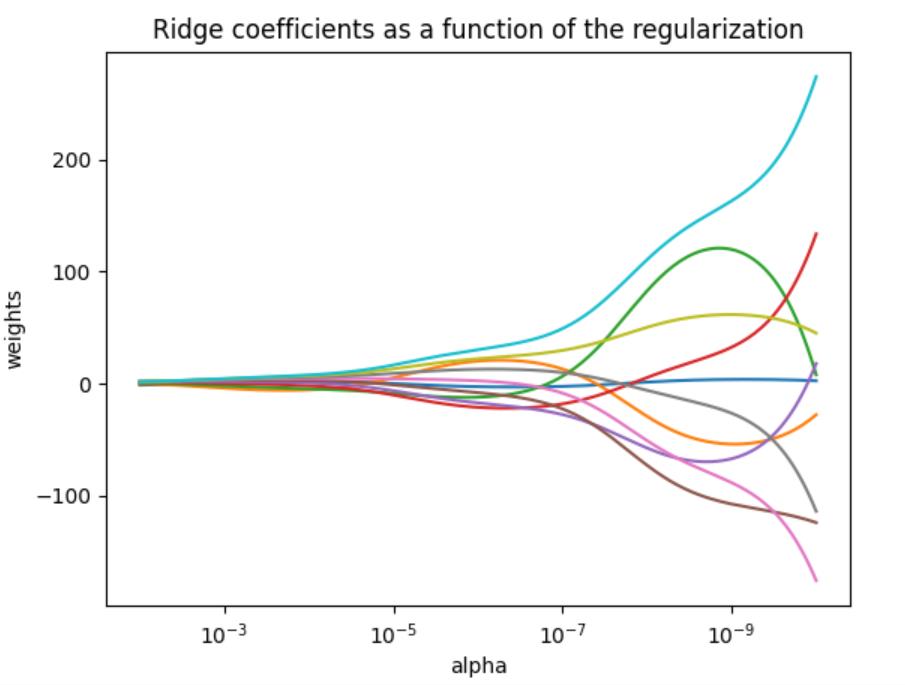
3、Lasso
import numpy as np
import matplotlib.pyplot as plt
from sklearn.metrics import r2_score
# #############################################################################
# Generate some sparse data to play with
np.random.seed(42)
n_samples, n_features = 50, 200
X = np.random.randn(n_samples, n_features)
coef = 3 * np.random.randn(n_features)
inds = np.arange(n_features)
np.random.shuffle(inds)
coef[inds[10:]] = 0 # sparsify coef
y = np.dot(X, coef)
# add noise
y += 0.01 * np.random.normal(size=n_samples)
# Split data in train set and test set
n_samples = X.shape[0]
X_train, y_train = X[:n_samples // 2], y[:n_samples // 2]
X_test, y_test = X[n_samples // 2:], y[n_samples // 2:]
# #############################################################################
# Lasso
from sklearn.linear_model import Lasso
alpha = 0.1
lasso = Lasso(alpha=alpha)
y_pred_lasso = lasso.fit(X_train, y_train).predict(X_test)
r2_score_lasso = r2_score(y_test, y_pred_lasso)
print(lasso)
print("r^2 on test data : %f" % r2_score_lasso)
plt.plot(lasso.coef_, color='gold', linewidth=2,
label='Lasso coefficients')
plt.plot(coef, '--', color='navy', label='original coefficients')
plt.legend(loc='best')
plt.title("Lasso R^2: %f"
% (r2_score_lasso))
plt.show()
结果:
Lasso(alpha=0.1)
r^2 on test data : 0.385982
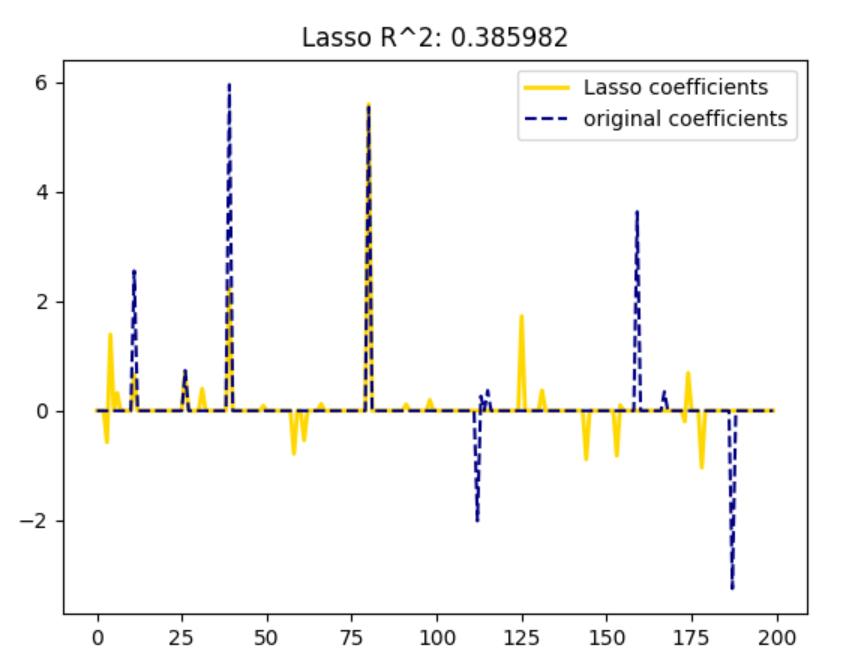
4、Logistics Regression
import numpy as np
import matplotlib.pyplot as plt
from sklearn.linear_model import LogisticRegression
from sklearn import datasets
# import some data to play with
iris = datasets.load_iris()
X = iris.data[:, :2] # we only take the first two features.
Y = iris.target
logreg = LogisticRegression(C=1e5, solver='lbfgs', multi_class='multinomial')
# Create an instance of Logistic Regression Classifier and fit the data.
logreg.fit(X, Y)
# Plot the decision boundary. For that, we will assign a color to each
# point in the mesh [x_min, x_max]x[y_min, y_max].
x_min, x_max = X[:, 0].min() - .5, X[:, 0].max() + .5
y_min, y_max = X[:, 1].min() - .5, X[:, 1].max() + .5
h = .02 # step size in the mesh
xx, yy = np.meshgrid(np.arange(x_min, x_max, h), np.arange(y_min, y_max, h))
Z = logreg.predict(np.c_[xx.ravel(), yy.ravel()])
# Put the result into a color plot
Z = Z.reshape(xx.shape)
plt.figure(1, figsize=(4, 3))
plt.pcolormesh(xx, yy, Z, cmap=plt.cm.Paired)
# Plot also the training points
plt.scatter(X[:, 0], X[:, 1], c=Y, edgecolors='k', cmap=plt.cm.Paired)
plt.xlabel('Sepal length')
plt.ylabel('Sepal width')
plt.xlim(xx.min(), xx.max())
plt.ylim(yy.min(), yy.max())
plt.xticks(())
plt.yticks(())
plt.show()
结果:
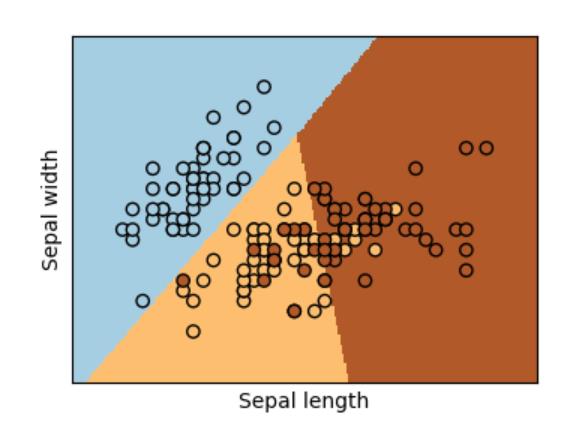
以上是关于学习笔记——sklearn监督学习:回归(简单数学知识罗列)的主要内容,如果未能解决你的问题,请参考以下文章
机器学习 sklearn 监督学习 回归算法 岭回归 Ridge Regression
机器学习 sklearn 监督学习 回归算法 岭回归 Ridge Regression