优化求解蜻蜓算法求解多目标优化问题matlab源码
Posted Matlab走起
tags:
篇首语:本文由小常识网(cha138.com)小编为大家整理,主要介绍了优化求解蜻蜓算法求解多目标优化问题matlab源码相关的知识,希望对你有一定的参考价值。
一、简介
蜻蜓算法(Dragonfly Algorithm)是由Seyedali Mirjalili在2015年提出的一种新兴群智能算法。Reynoldz指出三个关于蜂群体行为准则:分离度、对齐度与聚合度。分离度是指相邻个体间保持适当距离,以免碰撞;对齐度是指速度和方向与相邻个体对齐;聚合度是指个体飞向相邻区域中心。蜻蜓主要目标都是生存,Seyedali Mirjalili提出五个因素影响蜻蜓算法的位置更新:分离,列队,聚集,捕食,逃离。数学模型如下:
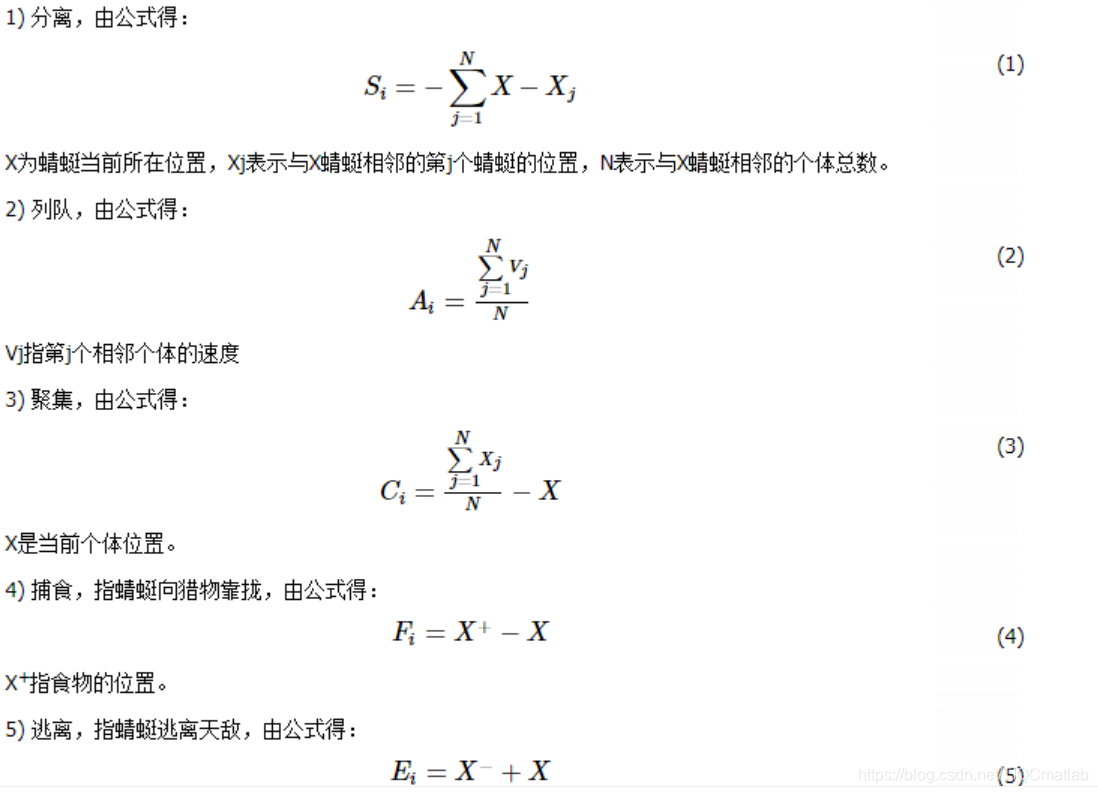
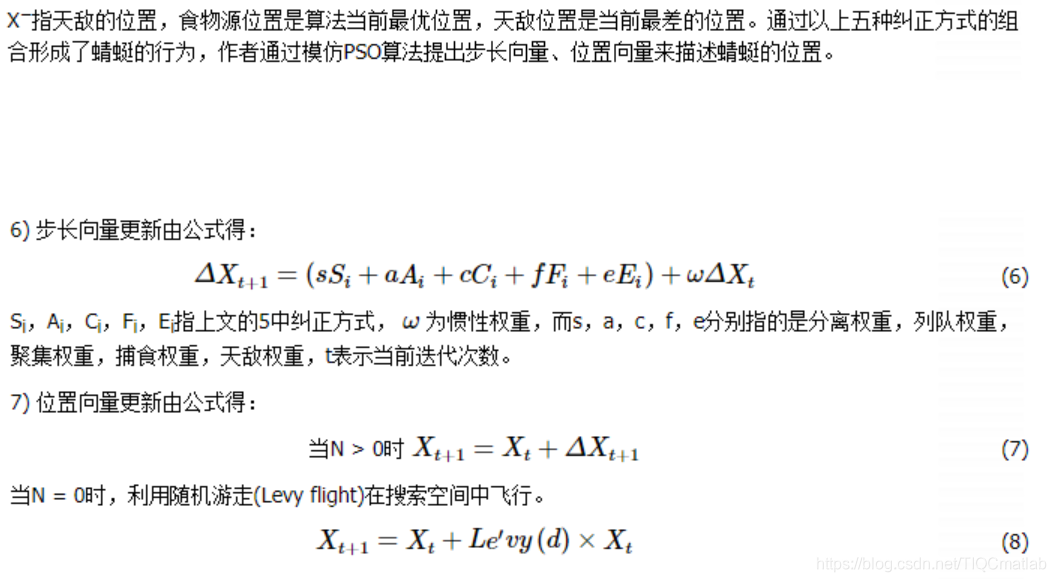
二、源代码
```c
%___________________________________________________________________%
% Multi-Objective Dragonfly Algorithm (MODA) source codes demo %
% version 1.0 %
% %
% Developed in MATLAB R2011b(7.13) %
% %
% Author and programmer: Seyedali Mirjalili %
% %
% e-Mail: ali.mirjalili@gmail.com %
% seyedali.mirjalili@griffithuni.edu.au %
% %
% Homepage: http://www.alimirjalili.com %
% %
% Main paper: %
% %
% S. Mirjalili, Dragonfly algorithm: a new meta-heuristic %
% optimization technique for solving single-objective, discrete, %
% and multi-objective problems, Neural Computing and Applications %
% DOI: http://dx.doi.org/10.1007/s00521-015-1920-1 %
%___________________________________________________________________%
clc;
clear;
close all;
% Change these details with respect to your problem%%%%%%%%%%%%%%
ObjectiveFunction=@ZDT1;
dim=5;
lb=0;
ub=1;
obj_no=2;
if size(ub,2)==1
ub=ones(1,dim)*ub;
lb=ones(1,dim)*lb;
end
%%%%%%%%%%%%%%%%%%%%%%%%%%%%%%%%%%%%%%%%%%%%%%%%%%%%%%%%%%%%%%%%%
% Initial parameters of the MODA algorithm
max_iter=100;
N=100;
ArchiveMaxSize=100;
Archive_X=zeros(100,dim);
Archive_F=ones(100,obj_no)*inf;
Archive_member_no=0;
r=(ub-lb)/2;
V_max=(ub(1)-lb(1))/10;
Food_fitness=inf*ones(1,obj_no);
Food_pos=zeros(dim,1);
Enemy_fitness=-inf*ones(1,obj_no);
Enemy_pos=zeros(dim,1);
X=initialization(N,dim,ub,lb);
fitness=zeros(N,2);
DeltaX=initialization(N,dim,ub,lb);
iter=0;
position_history=zeros(N,max_iter,dim);
for iter=1:max_iter
r=(ub-lb)/4+((ub-lb)*(iter/max_iter)*2);
w=0.9-iter*((0.9-0.2)/max_iter);
my_c=0.1-iter*((0.1-0)/(max_iter/2));
if my_c<0
my_c=0;
end
if iter<(3*max_iter/4)
s=my_c; % Seperation weight
a=my_c; % Alignment weight
c=my_c; % Cohesion weight
f=2*rand; % Food attraction weight
e=my_c; % Enemy distraction weight
else
s=my_c/iter; % Seperation weight
a=my_c/iter; % Alignment weight
c=my_c/iter; % Cohesion weight
f=2*rand; % Food attraction weight
e=my_c/iter; % Enemy distraction weight
end
for i=1:N %Calculate all the objective values first
Particles_F(i,:)=ObjectiveFunction(X(:,i)');
if dominates(Particles_F(i,:),Food_fitness)
Food_fitness=Particles_F(i,:);
Food_pos=X(:,i);
end
if dominates(Enemy_fitness,Particles_F(i,:))
if all(X(:,i)<ub') && all( X(:,i)>lb')
Enemy_fitness=Particles_F(i,:);
Enemy_pos=X(:,i);
end
end
end
[Archive_X, Archive_F, Archive_member_no]=UpdateArchive(Archive_X, Archive_F, X, Particles_F, Archive_member_no);
if Archive_member_no>ArchiveMaxSize
Archive_mem_ranks=RankingProcess(Archive_F, ArchiveMaxSize, obj_no);
[Archive_X, Archive_F, Archive_mem_ranks, Archive_member_no]=HandleFullArchive(Archive_X, Archive_F, Archive_member_no, Archive_mem_ranks, ArchiveMaxSize);
else
Archive_mem_ranks=RankingProcess(Archive_F, ArchiveMaxSize, obj_no);
end
Archive_mem_ranks=RankingProcess(Archive_F, ArchiveMaxSize, obj_no);
% Chose the archive member in the least population area as foods
% to improve coverage
index=RouletteWheelSelection(1./Archive_mem_ranks);
if index==-1
index=1;
end
Food_fitness=Archive_F(index,:);
Food_pos=Archive_X(index,:)';
% Chose the archive member in the most population area as enemies
% to improve coverage
index=RouletteWheelSelection(Archive_mem_ranks);
if index==-1
index=1;
end
Enemy_fitness=Archive_F(index,:);
Enemy_pos=Archive_X(index,:)';
for i=1:N
index=0;
neighbours_no=0;
clear Neighbours_V
clear Neighbours_X
% Find the neighbouring solutions
for j=1:N
Dist=distance(X(:,i),X(:,j));
if (all(Dist<=r) && all(Dist~=0))
index=index+1;
neighbours_no=neighbours_no+1;
Neighbours_V(:,index)=DeltaX(:,j);
Neighbours_X(:,index)=X(:,j);
end
end
% Seperation%%%%%%%%%%%%%%%%%%%%%%%%%%%%%%%%%%%%%%%%%%%%%%%%%%%
% Eq. (3.1)
S=zeros(dim,1);
if neighbours_no>1
for k=1:neighbours_no
S=S+(Neighbours_X(:,k)-X(:,i));
end
S=-S;
else
S=zeros(dim,1);
end
% Alignment%%%%%%%%%%%%%%%%%%%%%%%%%%%%%%%%%%%%%%%%%%%%%%%%%%%
% Eq. (3.2)
if neighbours_no>1
A=(sum(Neighbours_V')')/neighbours_no;
else
A=DeltaX(:,i);
end
% Cohesion%%%%%%%%%%%%%%%%%%%%%%%%%%%%%%%%%%%%%%%%%%%%%%%%%%%
% Eq. (3.3)
if neighbours_no>1
C_temp=(sum(Neighbours_X')')/neighbours_no;
else
C_temp=X(:,i);
end
C=C_temp-X(:,i);
% Attraction to food%%%%%%%%%%%%%%%%%%%%%%%%%%%%%%%%%%%%%%%%%
% Eq. (3.4)
Dist2Attraction=distance(X(:,i),Food_pos(:,1));
if all(Dist2Attraction<=r)
F=Food_pos-X(:,i);
iter;
else
F=0;
end
% Distraction from enemy%%%%%%%%%%%%%%%%%%%%%%%%%%%%%%%%%%%%%
% Eq. (3.5)
Dist=distance(X(:,i),Enemy_pos(:,1));
if all(Dist<=r)
E=Enemy_pos+X(:,i);
else
E=zeros(dim,1);
end
for tt=1:dim
if X(tt,i)>ub(tt)
X(tt,i)=lb(tt);
DeltaX(tt,i)=rand;
end
if X(tt,i)<lb(tt)
X(tt,i)=ub(tt);
DeltaX(tt,i)=rand;
end
end
if any(Dist2Attraction>r)
if neighbours_no>1
for j=1:dim
DeltaX(j,i)=w*DeltaX(j,i)+rand*A(j,1)+rand*C(j,1)+rand*S(j,1);
if DeltaX(j,i)>V_max
DeltaX(j,i)=V_max;
end
if DeltaX(j,i)<-V_max
DeltaX(j,i)=-V_max;
end
X(j,i)=X(j,i)+DeltaX(j,i);
end
```
三、运行结果
四、备注
完整代码或者代写添加QQ1575304183
以上是关于优化求解蜻蜓算法求解多目标优化问题matlab源码的主要内容,如果未能解决你的问题,请参考以下文章
多目标优化求解基于matlab粘菌算法MOSMA求解多目标优化问题含Matlab源码 2279期
多目标优化求解基于matlab飞蛾扑火算法 (NSMFO)求解多目标优化问题 含Matlab源码 2312期
多目标优化求解基于matlab飞蛾扑火算法 (NSMFO)求解多目标优化问题 含Matlab源码 2312期
多目标优化求解基于matlab遗传优化萤火虫算法求解多目标优化问题含Matlab源码 1484期