基于神经网络与支持向量机模型的隧道液化引起隆起位移预测
Posted UndergroundSpace期刊
tags:
篇首语:本文由小常识网(cha138.com)小编为大家整理,主要介绍了基于神经网络与支持向量机模型的隧道液化引起隆起位移预测相关的知识,希望对你有一定的参考价值。
点击蓝字关注地下空间
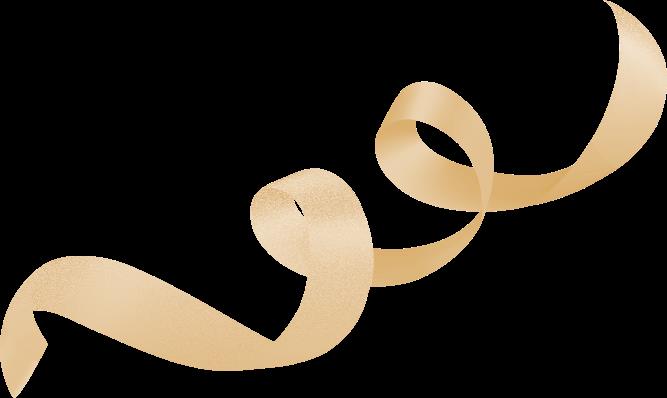
Neural network and support vector machine models for the prediction of the liquefaction-induced uplift displacement of tunnels
基于神经网络与支持向量机模型的隧道液化引起隆起位移预测
Underground Space, Available online 9 January 2020
https://doi.org/10.1016/j.undsp.2019.12.002
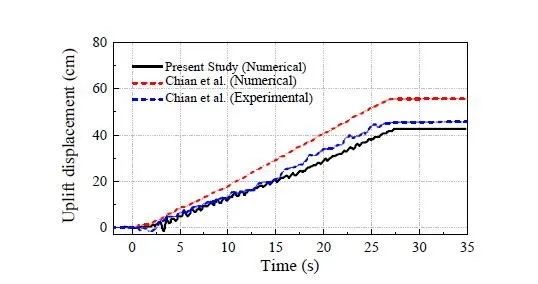
Comparison between the results of the simulation and centrifuge measurements
模拟结果与离心试验结果对比
Authors
作者
Gang Zheng(a,b,c), Wenbin Zhang(a,b), Wengang Zhang(d), Haizuo Zhou(a,b,c✉*), Pengbo Yang(a,b)
a. School of Civil Engineering, Tianjin University, Tianjin 300072, China
b. Key Laboratory of Coast Civil Structure Safety, Tianjin University, Ministry of Education, Tianjin 300072, China
c. State Key Laboratory of Hydraulic Engineering Simulation and Safety, Tianjin University, Tianjin 300072, China
d. National Joint Engineering Research Center of Geohazards Prevention in Reservoir Area Environment, School of Civil Engineering, Chongqing University, Chongqing 400045, China
✉ zhzrobby@163.com
Abstract
摘要
Tunnels buried in liquefiable deposits are vulnerable to liquefaction-induced uplift damage during earthquakes. This paper presents support vector machine (SVM) and artificial neural network (ANN) models for predicting the liquefaction-induced uplift displacement of tunnels based on artificial databases generated by the finite difference method (FDM). The performance of the SVM and ANN models are assessed using statistical parameters, including the coefficient of determination (R2), the mean absolute error (MAE), and the root mean squared error (RMSE). The applications for the above-mentioned approach for predicting the uplift displacement of tunnels are compared and discussed. A relative importance analysis is adopted to quantify the sensitivity of each input variable. The precision of the presented models is demonstrated using the centrifuge test results available from previous studies.
在地震期间,埋于可液化沉积物中的隧道容易受到液化引起的隆起破坏。基于采用有限差分法(FDM)生成的人工数据库,本文提出了一个支持向量机(SVM)和人工神经网络(ANN)模型,以预测由于液化引起的隧道隆起位移。使用统计参数以评估SVM和ANN模型性能,包括决定系数(R2)、平均绝对误差(MAE)和均方根误差(RMSE)。对比讨论了上述方法在预测隧道隆起位移预测中的应用。采用相对重要性分析量化了每个输入变量的灵敏度。采用既有研究提供的离心试验结果验证了提出模型的精度。
Keywords
关键词
artificial neural network; support vector machine; liquefaction; uplift displacement
人工神经网络;支持向量机;液化;隆起位移
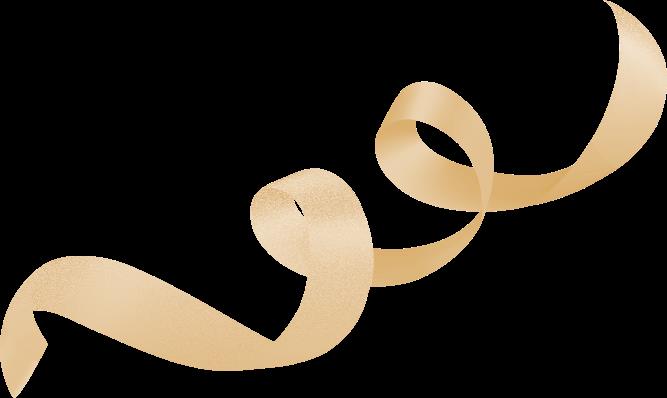
Underground Space
点击阅读原文查看文章详情
以上是关于基于神经网络与支持向量机模型的隧道液化引起隆起位移预测的主要内容,如果未能解决你的问题,请参考以下文章