TensorRT 与 TensorFlow 1.7 集成
Posted TensorFlow
tags:
篇首语:本文由小常识网(cha138.com)小编为大家整理,主要介绍了TensorRT 与 TensorFlow 1.7 集成相关的知识,希望对你有一定的参考价值。
上个月,我们宣布 NVIDIA® TensorRTTM 与 TensorFlow 集成。TensorRT 这个库针对推理优化了深度学习模型,并为在生产环境中的 GPU 上部署创建了一个运行时。它为 TensorFlow 带来了多项 FP16 和 INT8 优化,并且可以自动选择平台特定的内核,从而在 GPU 上进行推理期间最大程度增大吞吐量并尽可能减少延迟时间。
我们对全新的集成工作流程非常兴奋,因为新工作流程可以简化在 TensorFlow 内使用 TensorRT 的方式,并展现世界一流的性能。在测试中,我们发现与仅运行 TensorFlow 相比,ResNet-50 在使用 NVIDIA Volta Tensor 内核的 TensorFlow-TensorRT 集成上的速度提升了 8 倍,延迟时间仅为 7 ms。
TensorFlow 内的子图优化
现在,在 TensorFlow 1.7 中,TensorRT 可以优化兼容的子图并让 TensorFlow 执行其他工作。通过这种方式,用户可以利用丰富的 TensorFlow 功能集快速开发模型,在执行推理时又能使用 TensorRT 获得强大的优化。如果您已在使用 TensorRT 与 TensorFlow 模型,那么一定知道必须手动导入某些不受支持的 TensorFlow 层,导入过程有时非常耗时。
从工作流程角度来说,您需要让 TensorRT 优化 TensorFlow 的子图并将每个子图替换为 TensorRT 优化节点。这一步的输出是一个冻结图,这个冻结图可以像之前一样用于 TensorFlow。
在推理期间,TensorFlow 将为所有支持的区域执行图,并调用 TensorRT 来执行 TensorRT 优化节点。举例来说,如果您的图有 3 个段(A、B 和 C)。段 B 将被 TensorRT 优化并由一个节点替换。在推理期间,TensorFlow 先执行 A,然后调用 TensorRT 执行 B,之后 TensorFlow 将执行 C。
用于优化 TensorRT 的新添加 TensorFlow API 将获取冻结的 TensorFlow 图,向子图应用优化,并向 TensorFlow 发回一个应用了优化的 TensorRT 推理图。请参见下面的代码示例。
# Reserve memory for TensorRT inference engine gpu_options = tf.GPUOptions(per_process_gpu_memory_fraction = number_between_0_and_1) ... trt_graph = trt.create_inference_graph( input_graph_def = frozen_graph_def, outputs = output_node_name, max_batch_size=batch_size, max_workspace_size_bytes=workspace_size, precision_mode=precision) # Get optimized graph
per_process_gpu_memory_fraction 参数定义允许 TensorFlow 使用的 GPU 内存比例,剩余内存供 TensorRT 使用。应在首次启动 TensorFlow-TensorRT 过程时设置此参数。例如,如果值为 0.67,系统将为 TensorFlow 分配 67% 的 GPU 内存,剩余 33 % 的 GPU 内存将用于 TensorRT 引擎。
create_inference_graph 函数将获取冻结的 TensorFlow 图并返回一个带 TensorRT 节点的优化图。我们来看一下函数的参数:
input_graph_def: 冻结的 TensorFlow 图
outputs: 带有输出节点名称的字符串列表,例如 ["resnet_v1_50/predictions/Reshape_1"]
max_batch_size: 整型,输入批次的大小,例如 16
max_workspace_size_bytes: 整型,可用于 TensorRT 的最大 GPU 内存大小
precision_mode:字符串,允许的值为“FP32”、“FP16”或“INT8”
举例来说,如果 GPU 的内存为 12GB,要向 TensorRT 引擎分配大约 4GB,请将 per_process_gpu_memory_fraction 参数设为 (12 - 4) / 12 = 0.67,并将 max_workspace_size_bytes 参数设为 4000000000。
让我们向 ResNet-50 应用新 API 并查看优化模型在 TensorBoard 中的样子。运行示例的完整代码位于此处 。左侧图像为没有 TensorRT 优化的 ResNet-50,右侧为优化之后的 ResNet-50。在这种情况下,图的大部分都被 TensorRT 优化并由一个节点(突出显示)替换。
优化的 INT8 推理性能
TensorRT 能够获取以单精度 (FP32) 和半精度 (FP16) 训练的模型,并对它们进行转换,用于在降低的精度和并尽可能减小准确性损失的条件下通过 INT8 量化进行部署。INT8 模型的计算速度更快,并且对带宽的要求更低,不过,由于可用动态范围减小,神经网络权重和激活的表示存在挑战。
为了解决这个问题,TensorRT 使用了一个校准过程,这个过程可以在使用有限 8 位整型表示估算 FP32 网络时尽可能减小信息损失。利用新集成,在通过 TensorRT 优化 TensorFlow 图后,您可以将图传递到 TensorRT,按照下面所示进行校准。
trt_graph=trt.calib_graph_to_infer_graph(calibGraph)
推理工作流程剩余的上方部分保持不变。这一步的输出是一个冻结图,如上文所述,它将由 TensorFlow 执行。
在 NVIDIA Volta GPU 上自动使用 Tensor 内核
TensorRT 在 VOLTA GPU 中的 Tensor 内核上运行半精度 TensorFlow 模型来进行推理。Tensor 内核比单精度数学管道的吞吐量高 8 倍。与更高精度的 FP32 和 FP64 相比,半精度(也称为 FP16)数据可以减少神经网络的内存使用。这样可以训练和部署更大的网络,FP16 数据传输速度比 FP32 或 FP64 传输速度快。
每个 Tensor 内核都执行 D = A x B + C,其中 A、B、C 和 D 为矩阵。A 和 B 为半精度 4x4 矩阵,而 D 和 C 可以是半精度或单精度 4x4 矩阵。V100 上 Tensor 内核的峰值性能比双精度 (FP64) 大约快一个数量级 (10x),比单精度 (FP32) 大约快 4 倍。
可用性
我们对此次发布非常兴奋,并且将与 NVIDIA 继续紧密合作以增强此集成。我们期望新解决方案可以确保尽可能高的性能,同时保持 TensorFlow 的易用性和灵活性。随着 TensorRT 支持的网络越来越多,您将自然而然地从更新中受益,不用对您的代码进行任何更改。
要获取新的解决方案,在 TensorFlow 1.7 发布后,您可以使用标准的 pip 安装过程:
pip install tensorflow-gpu r1.7
在此之前,请从此处找到详细的安装说明:https://github.com/tensorflow/tensorflow/tree/r1.7/tensorflow/contrib/tensorrt
试用这个版本,并告诉我们您的想法!
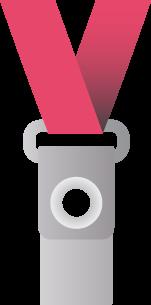
Be a Tensorflower
以上是关于TensorRT 与 TensorFlow 1.7 集成的主要内容,如果未能解决你的问题,请参考以下文章
无法在 tensorflow r1.14 中导入“tensorflow.contrib.tensorrt”