Pandas时间序列详解 | 轻松玩转Pandas
Posted AI派
tags:
篇首语:本文由小常识网(cha138.com)小编为大家整理,主要介绍了Pandas时间序列详解 | 轻松玩转Pandas相关的知识,希望对你有一定的参考价值。
教你学会 Pandas 不是我的目的,教你轻松玩转 Pandas 才是我的目的。我会通过一系列实例来带入 Pandas 的知识点,让你在学习 Pandas 的路上不再枯燥。
声明:我所写的轻松玩转 Pandas 教程都是免费的,如果对你有帮助,你可以持续关注我。
前文回顾:
内容目录
1. 基础概述
2. 转换时间戳
3. 生成时间戳范围
4. DatetimeIndex
5. DateOffset对象
6. 与时间序列相关的方法
6.1 移动
6.2 频率转换
6.3 重采样
# 导入相关库
import numpy as np
import pandas as pd
在做金融领域方面的分析时,经常会对时间进行一系列的处理。Pandas 内部自带了很多关于时间序列相关的工具,所以它非常适合处理时间序列。在处理时间序列的的过程中,我们经常会去做以下一些任务:
生成固定频率日期和时间跨度的序列
将时间序列整合或转换为特定频率
基于各种非标准时间增量(例如,在一年的最后一个工作日之前的5个工作日)计算“相对”日期,或向前或向后“滚动”日期
使用 Pandas 可以轻松完成以上任务。
基础概述
下面列出了 Pandas中 和时间日期相关常用的类以及创建方法。
类 | 备注 | 创建方法 |
---|---|---|
Timestamp | 时刻数据 | to_datetime,Timestamp |
DatetimeIndex | Timestamp的索引 | to_datetime,date_range,DatetimeIndex |
Period | 时期数据 | Period |
PeriodIndex | Period | period_range,PeriodIndex |
Pandas 中关于时间序列最常见的类型就是时间戳(Timestamp
)了,创建时间戳的方法有很多种,我们分别来看一看。
pd.Timestamp(2018, 5, 21)
Timestamp('2018-05-21 00:00:00')
pd.Timestamp("2018-5-21")
Timestamp('2018-05-21 00:00:00')
除了时间戳之外,另一个常见的结构是时间跨度(Period
)。
pd.Period("2018-01")
Period('2018-01', 'M')
pd.Period("2018-05", freq="D")
Period('2018-05-01', 'D')
Timestamp
和 Period
可以是索引。将Timestamp
和 Period
作为 Series
或 DataFrame
的索引后会自动强制转为为 DatetimeIndex
和 PeriodIndex
。
dates = [pd.Timestamp("2018-05-01"), pd.Timestamp("2018-05-02"), pd.Timestamp("2018-05-03"), pd.Timestamp("2018-05-04")]
ts = pd.Series(data=["Tom", "Bob", "Mary", "James"], index=dates)
ts.index
DatetimeIndex(['2018-05-01', '2018-05-02', '2018-05-03', '2018-05-04'], dtype='datetime64[ns]', freq=None)
periods = [pd.Period("2018-01"), pd.Period("2018-02"), pd.Period("2018-03"), pd.Period("2018-4")]
ts = pd.Series(data=["Tom", "Bob", "Mary", "James"], index=periods)
ts.index
PeriodIndex(['2018-01', '2018-02', '2018-03', '2018-04'], dtype='period[M]', freq='M')
转换时间戳
你可能会想到,我们经常要和文本数据(字符串)打交道,能否快速将文本数据转为时间戳呢?
答案是可以的,通过 to_datetime
能快速将字符串转换为时间戳。当传递一个Series时,它会返回一个Series(具有相同的索引),而类似列表的则转换为DatetimeIndex
。
pd.to_datetime(pd.Series(["Jul 31, 2018", "2018-05-10", None]))
0 2018-07-31
1 2018-05-10
2 NaT
dtype: datetime64[ns]
pd.to_datetime(["2005/11/23", "2010.12.31"])
DatetimeIndex(['2005-11-23', '2010-12-31'], dtype='datetime64[ns]', freq=None)
除了可以将文本数据转为时间戳外,还可以将 unix 时间转为时间戳。
pd.to_datetime([1349720105, 1349806505, 1349892905], unit="s")
DatetimeIndex(['2012-10-08 18:15:05', '2012-10-09 18:15:05',
'2012-10-10 18:15:05'],
dtype='datetime64[ns]', freq=None)
pd.to_datetime([1349720105100, 1349720105200, 1349720105300], unit="ms")
DatetimeIndex(['2012-10-08 18:15:05.100000', '2012-10-08 18:15:05.200000',
'2012-10-08 18:15:05.300000'],
dtype='datetime64[ns]', freq=None)
生成时间戳范围
有时候,我们可能想要生成某个范围内的时间戳。例如,我想要生成 "2018-6-26" 这一天之后的8天时间戳,如何完成呢?我们可以使用 date_range
和 bdate_range
来完成时间戳范围的生成。
pd.date_range("2018-6-26", periods=8)
DatetimeIndex(['2018-06-26', '2018-06-27', '2018-06-28', '2018-06-29',
'2018-06-30', '2018-07-01', '2018-07-02', '2018-07-03'],
dtype='datetime64[ns]', freq='D')
pd.bdate_range("2018-6-26", periods=8)
DatetimeIndex(['2018-06-26', '2018-06-27', '2018-06-28', '2018-06-29',
'2018-07-02', '2018-07-03', '2018-07-04', '2018-07-05'],
dtype='datetime64[ns]', freq='B')
可以看出,date_range
默认使用的频率是 日历日,而 bdate_range
默认使用的频率是 营业日。当然了,我们可以自己指定频率,比如,我们可以按周来生成时间戳范围。
pd.date_range("2018-6-26", periods=8, freq="W")
DatetimeIndex(['2018-07-01', '2018-07-08', '2018-07-15', '2018-07-22',
'2018-07-29', '2018-08-05', '2018-08-12', '2018-08-19'],
dtype='datetime64[ns]', freq='W-SUN')
DatetimeIndex
DatetimeIndex
的主要作用是之一是用作 Pandas 对象的索引,使用它作为索引除了拥有普通索引对象的所有基本功能外,还拥有简化频率处理的高级时间序列方法。
rng = pd.date_range("2018-6-24", periods=4, freq="W")
ts = pd.Series(range(len(rng)), index=rng)
ts
2018-06-24 0
2018-07-01 1
2018-07-08 2
2018-07-15 3
Freq: W-SUN, dtype: int32
# 通过日期访问数据
ts["2018-07-08"]
2
# 通过日期区间访问数据切片
ts["2018-07-08": "2018-07-22"]
2018-07-08 2
2018-07-15 3
Freq: W-SUN, dtype: int32
除了可以将日期作为参数,还可以将年份或者年份、月份作为参数来获取更多的数据。
# 传入年份
ts["2018"]
2018-06-24 0
2018-07-01 1
2018-07-08 2
2018-07-15 3
Freq: W-SUN, dtype: int32
# 传入年份和月份
ts["2018-07"]
2018-07-01 1
2018-07-08 2
2018-07-15 3
Freq: W-SUN, dtype: int32
除了可以使用字符串对 DateTimeIndex
进行索引外,还可以使用 datetime
(日期时间)对象来进行索引。
from datetime import datetime
ts[datetime(2018, 7, 8) : datetime(2018, 7, 22)]
2018-07-08 2
2018-07-15 3
Freq: W-SUN, dtype: int32
我们可以通过 Timestamp
或 DateTimeIndex
访问一些时间/日期的属性。这里列举一些常见的,想要查看所有的属性见官方链接:Time/Date Components(http://pandas.pydata.org/pandas-docs/stable/timeseries.html#time-date-components)
# 获取年份
ts.index.year
Int64Index([2018, 2018, 2018, 2018], dtype='int64')
# 获取星期几
ts.index.dayofweek
Int64Index([6, 6, 6, 6], dtype='int64')
# 获取一年中的几个第几个星期
ts.index.weekofyear
Int64Index([25, 26, 27, 28], dtype='int64')
DateOffset对象
DateOffset
从名称中就可以看出来是要做日期偏移的,它的参数与 dateutil.relativedelta
基本相同,工作方式如下:
from pandas.tseries.offsets import *
d = pd.Timestamp("2018-06-25")
d + DateOffset(weeks=2, days=5)
Timestamp('2018-07-14 00:00:00')
除了可以使用 DateOffset
完成上面的功能外,还可以使用偏移量实例来完成。
d + Week(2) + Day(5)
Timestamp('2018-07-14 00:00:00')
与时间序列相关的方法
在做时间序列相关的工作时,经常要对时间做一些移动/滞后、频率转换、采样等相关操作,我们来看下这些操作如何使用吧。
移动
如果你想移动或滞后时间序列,你可以使用 shift
方法。
ts.shift(2)
2018-06-24 NaN
2018-07-01 NaN
2018-07-08 0.0
2018-07-15 1.0
Freq: W-SUN, dtype: float64
可以看到,Series 所有的值都都移动了 2 个距离。如果不想移动值,而是移动日期索引,可以使用 freq
参数,它可以接受一个 DateOffset
类或其他 timedelta
类对象或一个 offset 别名,所有别名详细介绍见:Offset Aliases(http://pandas.pydata.org/pandas-docs/stable/timeseries.html#offset-aliases)。
ts.shift(2, freq=Day())
2018-06-26 0
2018-07-03 1
2018-07-10 2
2018-07-17 3
Freq: W-TUE, dtype: int32
可以看到,现在日期索引移动了 2 天的间隔。通过 tshift
同样可以达到相同的效果。
ts.tshift(2, freq=Day())
2018-06-26 0
2018-07-03 1
2018-07-10 2
2018-07-17 3
Freq: W-TUE, dtype: int32
频率转换
频率转换可以使用 asfreq
函数来实现。下面演示了将频率由周转为了天。
ts.asfreq(Day())
2018-06-24 0.0
2018-06-25 NaN
2018-06-26 NaN
2018-06-27 NaN
2018-06-28 NaN
2018-06-29 NaN
2018-06-30 NaN
2018-07-01 1.0
2018-07-02 NaN
2018-07-03 NaN
2018-07-04 NaN
2018-07-05 NaN
2018-07-06 NaN
2018-07-07 NaN
2018-07-08 2.0
2018-07-09 NaN
2018-07-10 NaN
2018-07-11 NaN
2018-07-12 NaN
2018-07-13 NaN
2018-07-14 NaN
2018-07-15 3.0
Freq: D, dtype: float64
聪明的你会发现出现了缺失值,因此 Pandas 为你提供了 method
参数来填充缺失值。几种不同的填充方法参考 中 fillna
介绍。
ts.asfreq(Day(), method="pad")
2018-06-24 0
2018-06-25 0
2018-06-26 0
2018-06-27 0
2018-06-28 0
2018-06-29 0
2018-06-30 0
2018-07-01 1
2018-07-02 1
2018-07-03 1
2018-07-04 1
2018-07-05 1
2018-07-06 1
2018-07-07 1
2018-07-08 2
2018-07-09 2
2018-07-10 2
2018-07-11 2
2018-07-12 2
2018-07-13 2
2018-07-14 2
2018-07-15 3
Freq: D, dtype: int32
重采样
resample
表示根据日期维度进行数据聚合,可以按照分钟、小时、工作日、周、月、年等来作为日期维度,更多的日期维度见 Offset Aliases(http://pandas.pydata.org/pandas-docs/stable/timeseries.html#offset-aliases)。
这里我们先以月来作为时间维度来进行聚合。
# 求出每个月的数值之和
ts.resample("1M").sum()
2018-06-30 0
2018-07-31 6
Freq: M, dtype: int32
# 求出每个月的数值平均值
ts.resample("1M").mean()
2018-06-30 0
2018-07-31 2
Freq: M, dtype: int32
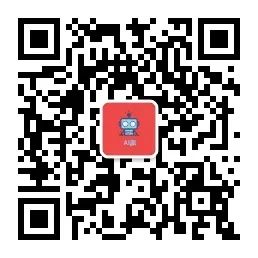
历史推荐:
以上是关于Pandas时间序列详解 | 轻松玩转Pandas的主要内容,如果未能解决你的问题,请参考以下文章
一个参数一个xlsx表,让你玩转Pandas中read_excel()表格读取!
pandas功能使用rename, reindex, set_index 详解