基于pytorch的CNN猫狗图分
Posted dai _ tu
tags:
篇首语:本文由小常识网(cha138.com)小编为大家整理,主要介绍了基于pytorch的CNN猫狗图分相关的知识,希望对你有一定的参考价值。
1.所需模块
2.前提知识
3.CNN简要
4.基本框架
5.代码
.
1.所需的模块
相关的作用在用到的时候单独讲。
import numpy as np
import matplotlib.pyplot as plt
import torch
import os
from PIL import Image
from torch.autograd import Variable
import torch.nn as nn
import torch.nn.functional as F
import torchvision.transforms as transforms
from torch.utils.data import DataLoader as DataLoader
import torch.utils.data
2.前提知识
1.训练集:(training data set):以下简称 Trset, Trset 类似于为了让计算机记住某些特征,而存储的一些带有标记的数据的一个集合。
2.测试集 : (testing data set ):以下简称 Teset, 用于检测 一数据是什么类别的集合。
3.激励函数的作用 (这里不多讲,csdn上面有很多)
4.简单神经网络构架
3.CNN简要:
CNN(Convolutional Neural Networks),卷积神经网络,以卷积的基本操作而命名,简单点的主要分3个部分:输入层(Input), 卷积层(Conv),池化层(Pool), 和 全连层(FC)
根据上图:
输入层:根据第一层Conv(Conv1),该层数据一共有3层,故Conc1的输入层是1个3通道的图片,事实也是这样,彩图是3通道(RGB)(3个feature map)的,灰图则1个通道(1 个 feature map);并且没每个像素点的范围为[0,255](像素点)。一般图片的数据形式则是 [h* w* c] ,其中对应的字母分别为 图片的 高,宽,通道数。
卷积层:
用于取特征,由卷积核对输入层图像进行卷积操作以提取图像特征。另外:卷积核(下图移动的部分):1个卷积核生成1个feature map,即卷积输出的图像通道数与卷积核的个数一致,卷积核的尺寸为(S×S×C×N),其中C表示卷积核深度,必须与输入层图像的通道数一致。
卷积的演示:
浅显易懂吧
池化层:
主要用于图像下采样,降低图像分辨率,减少区域内图像的特征数。本文用的池化方法为max pooling,max pooling就是在池化核大小区域内选择最大的数值作为输出结果。
池化的演示:
全连层:
用于分类的操作,若卷积后的图像尺寸为(h×w×c),需分成n类,则全连层的作用为将[h×w×c]的矩阵转换成[n×1]的矩阵。
4.基本框架:
准备数据:将数据集中的数据整理成程序代码可识别读取的形式。
搭建网络:利用PyTorch提供的API搭建设计的网络。
训练网络:把1中准备好的数据送入2中搭建的网络中进行训练,获得网络各节点权值参数(model)。
测试网络:导入3中获取的参数,并输入网络一个数据,然后评估网络的输出结果。
代码实现。
代码前言:准备数据之前,先吧同一文件夹下的 data文件准备好,data文件包train 和test文件,其中train 里的文件要命名为 cat(dog).x.jpg ,test里的从0排序就行了。
5.代码
getdata的代码如下:
import os #文件操作模块
import torch
import torch.utils.data as data #用于继承一个父类(data.Dataset)里的函数
from PIL import Image
import torchvision.transforms as Trans #在定义图片转换的格式时,会用到相关的函数
img_size = 200 #设置图片尺寸
tran = Trans.Compose([Trans.Resize(img_size), Trans.CenterCrop([img_size, img_size]), Trans.ToTensor()]) #封装, 对后面读取的图片的格式转换
class DogsVSCatsDataset(data.Dataset):
def __init__(self, mode, dir):
self.data_size = 0 #数据集的大小
self.img_list=[] #用于存图
self.img_label =[]#标签
self.trans=tran #转换的属性设置
self.mode =mode #下面打开集的模式
if self.mode =='train':
dir += '/train/' #更新地址
for file in os.listdir(dir): #遍历
self.img_list.append(dir+file) #存图
self.data_size += 1
name = file.split(sep='.') #将 该文件名拆分,便于判断是cat还是dog
label_x =0
if name[0] =='cat':
label_x =1
self.img_label.append(label_x)#设置入相对于的标签;cat:1; dog:0
elif self.mode == 'test':
dir +='/test/'
for file in os.listdir(dir):#同理
self.img_list.append(dir+file)
self.data_size +=1
self.img_label.append(2)#无意义的标签
else:
print("没有这个mode")
def __getitem__(self,item): #获取数据
if self.mode =='train':
img =Image.open(self.img_list[item])
label_y = self.img_label[item]
return self.trans(img), torch.LongTensor([label_y]) #返回该图片的地址和标签
elif self.mode=='test':
img =Image.open(self.img_list[item])
return self.trans(img)
else:
print("None")
def __len__(self):
return self.data_size
network代码如下
:
import torch
import torch.nn.functional as F #激励函数模块
import torch.nn as nn
import torch.utils.data as data
class Net(nn.Module): #继承nn.Module 里的东西
def __init__(self):
super(Net,self).__init__()
self.conv1 = torch.nn.Conv2d(3, 16,3, padding=1) #2个卷积层,Conv2d用于图片的卷积,还有Conv3d是用于视频的。
self.conv2 = torch.nn.Conv2d(16, 16, 3,padding=1)
self.fc1 = torch.nn.Linear(50*50*16, 128)#这里的50*50*16要根据后面 池化层的定义确定
self.fc2 = torch.nn.Linear(128, 64)
self.fc3 = torch.nn.Linear(64,2) #分为2类
def forward(self, x):
x = self.conv1(x) #卷积
x = F.relu(x)
x = F.max_pool2d(x, 2) #池化
x =self.conv2(x)
x = F.relu(x)
x = F.max_pool2d(x, 2)
x = x.view(x.size()[0], -1)
x = F.relu(self.fc1(x)) #全连接
x = F.relu(self.fc2(x))
x = self.fc3(x)
return F.softmax(x, dim=1)
上面的代码中,Conv2中的pading等参数作用,可以看看这篇blog
train 代码如下:
from getdata import DogsVSCatsDataset as DVCD
from torch.utils.data import DataLoader as DataLoader
from network import Net
import torch
from torch.autograd import Variable
import torch.nn as nn
dataset_dir = './data/' # 数据集路径
model_dir = './model/' # 网络参数保存位置
workers = 10 # 线程数量
batch_size = 16 # 一次训练所选取的样本数
lr = 0.001 # 学习率
nepoch = 1 #训练的次数
def train():
datafile = DVCD('train', dataset_dir) # 实例化
dataloader = DataLoader(datafile, batch_size=batch_size, shuffle=True, num_workers=workers, drop_last=True) #封装
print('Dataset loaded! length of train set is 0'.format(len(datafile)))
model = Net() # 实例化一个网络
model = nn.DataParallel(model) #传入
model.train() # 训练模式
optimizer = torch.optim.Adam(model.parameters(), lr=lr) # Adam优化器
Lossfuc = torch.nn.CrossEntropyLoss() # loss计算方法,cross entropy,交叉熵
cnt = 0 # 训练图片数量
for epoch in range(nepoch):
for img, label in dataloader:
img, label = Variable(img), Variable(label) # 将数据放置在PyTorch的Variable节点中
out = model(img)
loss = Lossfuc(out, label.squeeze()) # 计算损失
loss.backward()
optimizer.step()
optimizer.zero_grad()
cnt += 1
print('Epoch:0,Frame:1, train_loss 2'.format(epoch, cnt*batch_size, loss/batch_size))
torch.save(model.state_dict(), '0/model.pth'.format(model_dir)) # 训练所有数据后,保存网络的参数
if __name__ == '__main__':
train()
test 的代码如下:
from getdata import DogsVSCatsDataset as DVCD #test里的基本框架和train里的差不多,这里不细讲
from network import Net
from torch.autograd import Variable
import numpy as np
import torch.nn.functional as F
import torch
import torch.nn as nn
import matplotlib.pyplot as plt
from PIL import Image
import os
os.environ["KMP_DUPLICATE_LIB_OK"] = "TRUE" #防止打印图片出错
data_dir = './data/'
model_file = './model/model.pth'
N = 10
model =Net()
model = nn.DataParallel(model)
model.load_state_dict(torch.load(model_file))
model.eval()
datafile = DVCD('test', data_dir)
index = np.random.randint(0, datafile.data_size, 1)[0]
img = datafile.__getitem__(index)
img = img.unsqueeze(0)
img = Variable(img)
out =model(img)
out = F.softmax(out, dim=1)
print(out)
if out[0, 0]>out[0, 1]:
print("the picture is a cat")
else:
print("the picture is a dog")
img = Image.open(datafile.img_list[index])
plt.figure('image')
plt.imshow(img)
plt.show()
效果图:
mood:
没有谁忘不了谁吧
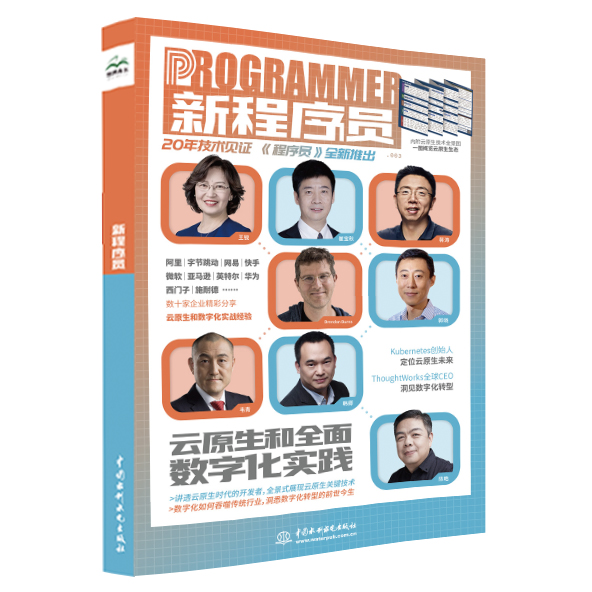

以上是关于基于pytorch的CNN猫狗图分的主要内容,如果未能解决你的问题,请参考以下文章
图像分类猫狗分类实战—基于pytorch框架的迁移学习(ResNet50模型实现分类实战)
基于pytorch使用实现CNN 如何使用pytorch构建CNN卷积神经网络