Elasticsearch:高级数据类型介绍
Posted Elastic 中国社区官方博客
tags:
篇首语:本文由小常识网(cha138.com)小编为大家整理,主要介绍了Elasticsearch:高级数据类型介绍相关的知识,希望对你有一定的参考价值。
在我之前的文章 “Elasticsearch:一些有趣的数据类型”,我已经介绍了一下很有趣的数据类型。在今天的文章中,我再进一步介绍一下高级的数据类型,虽然这里的数据类型可能和之前的一些数据类型有所重复。即便如此,我希望能从另外的一个方面来描述这些数据类型。希望大家能在自己的应用中熟练地运用这些数据类型。
Geopoint(geo_point)数据类型
我们中的大多数人可能在圣诞节期间使用过智能设备来查找最近的餐馆的位置,或者询问过 GPS 导航到我们奶奶家的方向。 Elasticsearch 开发了一种专门的数据类型 geo_point 用于捕获地点的位置。
位置数据表示为 geo_point 数据类型,表示经度和纬度。 我们可以使用它来确定餐厅、学校、高尔夫球场等的地址。
下面显示的代码清单演示了名为 restaurants 的索引的模式定义。 它保护带有名称和地址的餐馆。 值得注意的是,地址字段被定义为 geo_point 数据类型:
# A restaurants index with address declared as geo_point
PUT restaurants
"mappings":
"properties":
"name":
"type": "text"
,
"address":
"type": "geo_point"
现在我们有了一个索引,让我们索引一个示例餐厅(位于伦敦的虚构 Sticky Fingers),其位置以经度和纬度形式提供(列表如下):
# Indexing a restaurant - the location is provided as lon and lat
PUT restaurants/_doc/1
"name": "Sticky Fingers",
"address":
"lon": "0.1278",
"lat": "51.5074"
在上面的代码片段中,餐厅的地址以经度 (lon) 和纬度 (lat) 对的形式提供。 还有其他方法可以提供这些输入,我们稍后会介绍。上面的位置使用 Elastic Maps 可以显示如下:
我们无法搜索和获取周边位置内的餐厅。 我们可以使用 geo_bounding_box 查询来搜索涉及地理地址的数据。 它需要输入 top_left 和 bottom_right 点来围绕我们的兴趣点创建一个框起来的区域,如下图所示
我们使用 lon(经度)和 lat(纬度)对(地址位置指向伦敦)为该查询提供上限和下限。我们编写 geo_bounding_box 查询以矩形的形式提供地址,其中 top_left 和 bottom_right 坐标以纬度和经度提供,如下面的清单所示
# Listing to Fetch the restaurants around a geographical location
GET restaurants/_search?filter_path=**.hits
"query":
"geo_bounding_box":
"address":
"top_left":
"lon": "0",
"lat": "52"
,
"bottom_right":
"lon": "1",
"lat": "50"
此查询获取我们的餐厅,因为地理边界框包含我们的餐厅:
"hits":
"hits": [
"_index": "restaurants",
"_id": "1",
"_score": 1,
"_source":
"name": "Sticky Fingers",
"address":
"lon": "0.1278",
"lat": "51.5074"
]
正如我之前提到的,我们可以提供各种格式的位置信息,而不仅仅是纬度和经度:例如,数组或字符串。 下表提供了创建位置数据的方法和示例:
更多关于 geo_point 的搜索,请参考:
-
Elastic:开发者上手指南 中的 “Maps” 章节
对象数据类型 - object data type
我们经常以分层方式查找数据,例如一封电子邮件,其中包含顶级字段,如 subject、to 和 from 字段以及用于保存附件的内部对象,如下面的代码片段所示:
"to:":"johndoe@johndoe.com",
"subject":"Testing Object Type",
"attachments":
"filename":"file1.txt",
"filetype":"confidential"
JSON 允许我们创建这样的分层对象:一个包含在其他对象中的对象。 为了表示这种对象层次结构,Elasticsearch 有一种特殊的数据类型来表示对象的层次结构 —— 对象类型。 在上面的示例中,由于附件(attachments)包含其他属性,我们将其归类为对象本身,因此属于对象类型。 attachments 对象中的 filename 和 filetype 这两个属性可以分别建模为 text 和 text 字段。 有了这些信息,我们就可以创建一个映射定义,如下面的清单所示:
# Defining the attatchments as object type. Though we can set the type as object speficially, Elasticsearch is clever enough to deduce it as an object type when it sees hierarchical data sets. Hence we can omit declaring the object type
PUT emails
"mappings":
"properties":
"to":
"type": "text"
,
"subject":
"type": "text"
,
"attachments":
"type":"object",
"properties":
"filename":
"type": "text"
,
"filetype":
"type": "text"
attachments 字段是一个对象,因为它封装了其他两个字段。 虽然我们已经明确提到类型是 object,但 Elasticsearch 并不期望我们这样做。 每当遇到具有分层数据的字段时,它将字段的数据类型设置为 object。我们其实甚至可以省去 "type": "object" 这一行。
模式成功执行后,我们可以通过调用 GET emails/_mapping 命令来检索它(清单如下):
GET emails/_mapping
# The mapping schema for emails
# The attachments type is not listed (inferred as object by Elasticsearch!)
"emails" :
"mappings" :
"properties" :
"attachments" :
"properties" :
"filename" : "type" : "text",
"filetype" : "type" : "text"
,
"subject" : "type" : "text",
"to" : "type" : "text"
虽然所有其他字段都显示其关联的数据类型,但 attachments 不会。 内部对象的对象类型由 Elasticsearch 默认推断。 让我们索引一个电子邮件文档,下面给出的清单显示了查询:
#Indexing an email document
PUT emails/_doc/1
"to:": "johndoe@johndoe.com",
"subject": "Testing Object Type",
"attachments":
"filename": "file1.txt",
"filetype": "confidential"
现在我们已经用文档准备好我们的电子邮件索引,我们可以在内部对象字段上发出匹配搜索查询(我们将在接下来的文章中了解搜索查询)以获取相关文档(并证明我们的观点),如清单如下:
# Searching for an email based on the attachment name
GET emails/_search?filter_path=**.hits
"query":
"match":
"attachments.filename": "file1.txt"
上面命令的响应为:
"hits":
"hits": [
"_index": "emails",
"_id": "1",
"_score": 0.5753642,
"_source":
"to:": "johndoe@johndoe.com",
"subject": "Testing Object Type",
"attachments":
"filename": "file1.txt",
"filetype": "confidential"
]
这将从我们的 Elasticsearch 返回文档,因为 filename 与我们在 Elasticsearch 中的文档相匹配。
虽然对象类型非常简单,但它们有一个局限性:内部对象被扁平化并且不存储为单独的文档。 此操作的缺点是从数组索引的对象之间的关系丢失。 好消息是我们有另一种称为嵌套(nested)数据的数据类型来解决这个问题。
遗憾的是,由于篇幅所限,我无法在此处介绍对象的局限性 —— 你可以在 “Elasticsearch: object 及 nested 数据类型” 做更进一步的阅读。
嵌套数据类型 - nested data type
嵌套数据类型是对象类型的特殊形式,其中维护文档中对象数组之间的关系。
以我们的电子邮件和附件为例,这次让我们将附件字段定义为 nested 数据类型,而不是让 Elasticsearch 将其派生为对象类型。 这需要通过将附件字段声明为 nested 数据类型来创建模式。 该模式显示在下面给出的清单中:
# Creating the attachments field as nested datatype
PUT emails_nested
"mappings":
"properties":
"attachments":
"type": "nested",
"properties":
"filename":
"type": "keyword"
,
"filetype":
"type": "text"
我们已经创建了一个模式(schema)定义,所以我们需要做的就是索引一个文档。 下面给出的清单正是这样做的:
# Indexing a document with attachments
PUT emails_nested/_doc/1
"attachments": [
"filename": "file1.txt",
"filetype": "confidential"
,
"filename": "file2.txt",
"filetype": "private"
]
一旦该文档被成功索引,拼图的最后一块就是搜索。 下面的清单将演示为获取文档而编写的搜索查询——标准是带有 file1.txt 附件的电子邮件和 private 分别作为文件名及其分类类型。 这种组合不存在,因此结果必须为空,这与交叉搜索数据的 object 不同。
# This query shoulnd't return resutls as we don't have file name as "file1.txt" and type as "private" data (look at the document above)
GET emails_nested/_search
"query":
"nested":
"path": "attachments",
"query":
"bool":
"must": [
"match":
"attachments.filename": "file1.txt"
,
"match":
"attachments.filetype": "private"
]
上面清单中的查询正在搜索一个名为 file1.txt 的文件,该文件具有不存在的 private 分类(查看我们之前索引的文档)。 此查询没有返回任何文档,这正是我们所期望的。 file1.txt 的分类是 confidential 的而不是 private 的,因此它不匹配。 因此,当 nested 类型表示内部对象数组时,单个对象将作为隐藏文档进行存储和索引。
Nested 数据类型非常擅长尊重关联和关系,因此如果我们需要创建一个对象数组,其中每个对象都必须被视为一个单独的对象,nested 数据类型将成为我们的朋友。
更多阅读,请参阅 “Elasticsearch: object 及 nested 数据类型”。
没有数组类型
当我们谈到数组时,有趣的是,Elasticsearch 中没有数组数据类型。 但是,我们可以为任何字段设置多个值,从而将字段表示为一个数组。 例如,具有一个 name 字段的文档可以从单个值更改为数组:"name": "John Doe" 到 "name": ["John Smith", "John Doe"] 只需添加一个列表数据值到字段。 创建数组时必须考虑一个重点:不能将数组与各种类型混在一起。 例如,你不能像这样声明 name 字段:"name": ["John Smith", 13, "Neverland"]。 这是非法的,因为该字段由多种类型组成,是不允许的。
Flattened(扁平化)数据类型
到目前为止,我们已经研究了对从 JSON 文档解析的各个字段建立索引。 在分析和存储时,每个字段都被视为一个单独且独立的字段。 然而,有时我们可能不需要将所有子字段作为单独的字段进行索引,从而让它们通过分析过程。 想一想聊天系统上的聊天消息流、现场足球比赛中的评论、医生记录病人的病痛等等。 我们可以将这种数据作为一个大 blob 加载,而不是显式声明每个字段(或动态派生)。 Elasticsearch 为此提供了一种称为扁平化的特殊数据类型。
flattened 数据类型以一个或多个子字段的形式保存信息,每个子字段的值作为关键字索引。 也就是说,没有一个值被视为文本字段,因此不经过文本分析过程。更多关分析方面的知识,请参考文章 “Elasticsearch: analyzer”。
让我们考虑一个医生在咨询期间记录他/她的病人的运行笔记的例子。 该映射由两个字段组成:患者 name 和 doctor_notes - doctor_notes 字段被声明为 flattened 类型。 下面给出的清单提供了映射:
# Listing for Creating a mapping with flattened data type
PUT consultations
"mappings":
"properties":
"patient_name":
"type": "text"
,
"doctor_notes":
"type": "flattened"
任何声明为 flattened 的字段(及其子字段)都不会被分析。 即所有的值都被索引为 keyword。 让我们创建一个患者咨询文档(在下面列出)并为其编制索引:
# The consultation document with doctor’s notes
PUT consultations/_doc/1
"patient_name": "John Doe",
"doctor_notes":
"temperature": 103,
"symptoms": [
"chills",
"fever",
"headache"
],
"history": "none",
"medication": [
"Antibiotics",
"Paracetamol"
]
如你所见,doctor_notes 包含大量信息,但请记住我们并未在映射定义中创建这些内部字段。 由于doctor_notes是一个 flattened 的类型,所以所有的值都被索引为 keyword。
最后,我们使用医生笔记中的任何关键字搜索索引,如下所示:
# Searching for patients prescribed with paracetomol
GET consultations/_search?filter_path=**.hits
"query":
"match":
"doctor_notes": "Paracetamol"
上面命令返回的结果为:
"hits":
"hits": [
"_index": "consultations",
"_id": "1",
"_score": 0.44303042,
"_source":
"patient_name": "John Doe",
"doctor_notes":
"temperature": 103,
"symptoms": [
"chills",
"fever",
"headache"
],
"history": "none",
"medication": [
"Antibiotics",
"Paracetamol"
]
]
搜索 Paracetamol 将返回我们的 John Doe 的咨询文件。 你可以通过将匹配查询更改为任何字段来进行试验,例如:"doctor_notes": "chills" 或者甚至编写如下所示的复杂查询:
#An advanced query to fetch patients based on multiple search criteria
# Search for non-diabetic patients with headache and prescribed with antibiotcs
GET consultations/_search?filter_path=**.hits
"query":
"bool":
"must": ["match": "doctor_notes": "headache",
"match": "doctor_notes": "Antibiotics"],
"must_not": ["term": "doctor_notes": "value": "diabetics"]
在查询中,我们检查 headaches (头痛)和 antibiotics(抗生素),但患者不应该患有 diabetic(糖尿病)—— 查询返回 John Doe,因为他没有糖尿病但有头痛并且正在服用抗生素(快点好起来,Doe!)。
Flattened 的数据类型会派上用场,尤其是当我们期望有很多临时的字段并且必须事先为所有字段定义映射定义是不可行的时候。 请注意,flattened 字段的子字段始终是 keyword 类型。
更多关于 flattened 数据类型的内容,请阅读文章 “Elasticsearch:Flattened 数据类型映射”。
Join 数据类型
如果你来自关系数据库世界,你就会知道数据之间的关系 —— joins —— 支持父子关系。 然而,在 Elasticsearch 中,每个被索引的文档都是独立的,并且与该索引中的任何其他文档都没有关系。 Elasticsearch 对数据进行反规范化,以在索引和搜索操作期间提高速度和性能。 Elasticsearch 提供了一个 join 数据类型来考虑我们需要的父子关系。
考虑一个医患(一对多)关系的例子:一个医生可以有多个病人,每个病人被分配给一个医生。
让我们创建一个 doctors 索引,其中包含一个包含关系定义的模式。 要使用 join 数据类型处理父子关系,我们需要
- 创建一个 join 类型的字段和
- 通过提及 relations 的关系对象添加附加信息(例如,当前上下文中的医患关系)
如下命令准备具有模式定义的 doctors 索引:
# Creating an indx with join datatype - make sure you create a field with the name "relations"
PUT doctors
"mappings":
"properties":
"relationship":
"type": "join",
"relations":
"doctor": "patient"
一旦我们准备好模式并建立索引,我们就会索引两种类型的文档:一种代表 doctor(父),另一种代表 patient(子)。 这是医生的文件,其中提到了作为医生的关系:
#Indexing a doctor - make sure the relationship field is set to doctor type
PUT doctors/_doc/1
"name": "Dr Mary Montgomery",
"relationship":
"name": "doctor"
上面代码片段中值得注意的一点是关系对象将文档类型声明为 doctor。 name 属性必须是在 relations 标记下的映射模式中声明的父值 (doctor)。 一旦我们的住院医生 Mary Montgomery 医生准备就绪,下一步就是让两名患者与她联系。 以下查询(下面列出)执行此操作:
# Listing for Creating two patients for our doctor
PUT doctors/_doc/2?routing=mary
"name": "John Doe",
"relationship":
"name": "patient",
"parent": 1
PUT doctors/_doc/3?routing=mary
"name": "Mrs Doe",
"relationship":
"name": "patient",
"parent": 1
关系对象的值应设置为 patient(还记得模式中关系属性的父子部分吗?)并且应该为父对象分配关联医生的文档标识符(在我们的示例中为 ID 1)。
在处理父子关系时,我们还需要了解一件事。 父母和相关的孩子将被索引到同一个分片中,以避免多分片搜索开销。 由于文档应该共存,我们需要在 URL 中使用强制 routing 参数。 路由是一个函数,可以确定文档所在的分片。
最后,是时候搜索属于 ID 为 1 的医生的患者了。下面列表中的查询搜索与 Montgomery 医生相关的所有患者:
# Searching for all patients of Dr Montgomery
GET doctors/_search?filter_path=**.hits
"query":
"parent_id":
"type": "patient",
"id": 1
上面的响应为:
"hits":
"hits": [
"_index": "doctors",
"_id": "2",
"_score": 0.10536051,
"_routing": "mary",
"_source":
"name": "John Doe",
"relationship":
"name": "patient",
"parent": 1
,
"_index": "doctors",
"_id": "3",
"_score": 0.10536051,
"_routing": "mary",
"_source":
"name": "Mrs Doe",
"relationship":
"name": "patient",
"parent": 1
]
当我们希望获取属于医生的患者时,我们使用一个名为 parent_id 的搜索查询,该查询需要子类型(患者)和父 ID(Montgomery 医生文档 ID 为 1)。 该查询将返回 Montgomery 医生的患者 —— Doe 先生和夫人。
使用 join 数据类型并不是直截了当的,因为我们要求非关系数据存储引擎处理关系 —— 有点要求太多,所以只有在你必须的时候才使用 join 数据类型。
在 Elasticsearch 中实现父子关系会对性能产生影响。 如果你正在考虑文档关系,Elasticsearch 可能不是合适的工具,因此请谨慎使用此功能。
更多阅读,请参阅
Search as you type 数据类型
当我们在搜索栏中键入时,大多数搜索引擎会建议单词和短语。 这个功能有几个名字 —— 通常有几个名字:搜索即输入或预先输入或自动完成或建议。 Elasticsearch 提供了一种方便的数据类型 —— search_as_you_type —— 来支持这个特性。 在幕后,Elasticsearch 非常努力地确保标记为 search_as_you_type 的字段被索引以生成 n-gram,我们将在本节中看到它的实际应用。
n-gram 是给定大小的单词序列。 例如,如果单词是 “action”,则 3-ngram(大小为 3 的 ngram)是:["act", "cti","tio","ion"] 和 bi-grams(大小为 2)是: [“ac”、“ct”、“ti”、“io”、“on”] 等。
另一方面,edge n-gram 是每个单词的 n-gram,其中 n-gram 的开头锚定到单词的开头。 以 “action” 这个词为例,边 n-gram 产生:["a","ac","act","acti","actio","action"]。
另一方面,Shingles 是单词 n-gram。 例如 “Elasticsearch in Action” 这句话会输出:["Elasticsearch", "Elasticsearch in", "Elasticsearch in Action", "in", "in Action", "Action"]
比如说,我们被要求支持对 books 索引的预输入查询,即,当用户开始在搜索栏中逐字输入书名时,我们应该能够根据他/她输入的字母推荐这本书 .
首先,我们需要创建一个模式,其中所讨论的字段是 search_as_you_type 数据类型。 下面的列表提供了这个映射模式:
# Mapping schema for technical books with the title defined as search_as_you_type datatype
PUT tech_books
"mappings":
"properties":
"title":
"type": "search_as_you_type"
我们现在索引几本书:
# Indexing few documents
PUT tech_books/_doc/1
"title": "I love Elasticsearch technology"
PUT tech_books/_doc/2
"title":"Elasticsearch is the most popular search engine in the world"
PUT tech_books/_doc/3
"title":"Elastic is the company behind Elasticsearch"
由于 title 字段的类型是 search_as_you_type 数据类型,因此 Elasticsearch 在根字段(title)之外创建了一组称为 n-gram 的子字段,如下表所示:
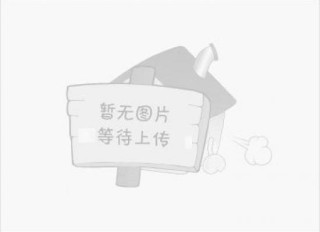
由于这些字段是为我们额外创建的,因此在该字段上搜索有望返回预输入建议,因为 n-gram 有助于有效地生成它们。
让我们创建搜索查询,如下面的清单所示。
# Searching in a search_as_you_type field and its subfields
GET tech_books/_search?filter_path=**.hits
"query":
"multi_match":
"query": "elas",
"type": "bool_prefix",
"fields": [
"title",
"title._2gram",
"title._3gram"
]
上面命令显示的结果是:
"hits":
"hits": [
"_index": "tech_books",
"_id": "1",
"_score": 1,
"_source":
"title": "Elasticsearch in Action"
,
"_index": "tech_books",
"_id": "3",
"_score": 1,
"_source":
"title": "Elastic Stack in Action"
]
此查询应返回所有的 3 个文档。 我们使用多重匹配查询,因为我们正在跨多个字段搜索一个值 —— title、title._2gram、title._3gram、title._index_prefix。
"hits":
"hits": [
"_index": "tech_books",
"_id": "1",
"_score": 1,
"_source":
"title": "I love Elasticsearch technology"
,
"_index": "tech_books",
"_id": "2",
"_score": 1,
"_source":
"title": "Elasticsearch is the most popular search engine in the world"
,
"_index": "tech_books",
"_id": "3",
"_score": 1,
"_source":
"title": "Elastic is the company behind Elasticsearch"
]
更多关于 search_as_you_type 的介绍,请参阅文章 “Elasticsearch:使用 search_analyzer 及 edge ngram 来实现 Search-As-You-Type”。
总结
在本文中,我们学习了高级数据类型,如 object、nested、flattened 以及其他如 geo_point 和 search_as_you_type。 有关其他数据类型的更多详细信息以及深入的讨论和代码示例,请详细参阅 “Elastic:开发者上手指南”。
ElasticSearch实战(十六)-高级数据字段类型
本文主要讲解ElasticSearch高级数据字段类型,帮助大家打好ElasticSearch基础。
——continue
以上是关于Elasticsearch:高级数据类型介绍的主要内容,如果未能解决你的问题,请参考以下文章
Elasticsearch:从实例中学习 nested 数据类型的 CRUD 及搜索
Elasticsearch:从实例中学习 nested 数据类型的 CRUD 及搜索