三维几何学习从零开始网格上的深度学习-2:卷积网络CNN篇(Pytorch)
Posted 大米粥哥哥
tags:
篇首语:本文由小常识网(cha138.com)小编为大家整理,主要介绍了三维几何学习从零开始网格上的深度学习-2:卷积网络CNN篇(Pytorch)相关的知识,希望对你有一定的参考价值。
本文参加新星计划人工智能(Pytorch)赛道:https://bbs.csdn.net/topics/613989052
从零开始网格上的深度学习-2:卷积网络CNN篇
引言
本文主要内容如下:
- 介绍网格上基于
面元素
的卷积操作 - 参考最新的CNN网络模块-
ConvNeXt
1:A ConvNet for the 2020s,构造网格分类网络
一、概述
1.1 卷积操作简述
卷积网络的核心:卷积操作
就是数据元素特征与周围元素特征加权求和的一个计算过程。由卷积层实现,包括步长、卷积核大小等参数。
详情可百度或参考2:python 关于CNN的一些思考-2022
1.2 网格上的面卷积
无论水密or非水密的网格,其上的面并不是规则排列的。但对于三角形网格来说,每个面周围存在三个面
,借助以上特性可对每个面构造1
×
\\times
× 4的卷积区域,然后借助Pytorch即可轻松将CNN应用到网格的面上,称其为面卷积
。
二、核心代码
2.1 面卷积
主要参考MeshCNN
3:A Network with an Edge中边卷积的代码即可,自己造的轮子没人家的好用…
- 让网格面及其邻面形成 ∣ F ∣ × |F| \\times ∣F∣× 4的矩阵结构, ∣ F ∣ |F| ∣F∣是面的个数。类似2D图像的长 × \\times ×宽
- 调用Pytorch中的Conv2d 或 Conv1d即可
class FaceConv(nn.Module):
"""
Face convolution with convolution region (参考 MeshCNN)
"""
def __init__(self, dim_in, dim_out, k, groups=1, bias=True):
super(FaceConv, self).__init__()
self.conv = nn.Conv2d(dim_in, dim_out, kernel_size=(1, k), groups=groups, bias=bias)
self.k = k
def __call__(self, edge_f, mesh):
return self.forward(edge_f, mesh)
def forward(self, x, mesh):
if self.k == 1:
# x = x.squeeze(-1)
x = self.conv(x)
else:
x = x.squeeze(-1)
G = torch.cat([self.pad_gemm(i, x.shape[2], x.device) for i in mesh], 0) # batchsize
G = self.create_GeMM(x, G)
x = self.conv(G)
return x
def flatten_gemm_inds(self, Gi):
(b, ne, nn) = Gi.shape
ne += 1
batch_n = torch.floor(torch.arange(b * ne, device=Gi.device).float() / ne).view(b, ne)
add_fac = batch_n * ne
add_fac = add_fac.view(b, ne, 1)
add_fac = add_fac.repeat(1, 1, nn)
Gi = Gi.float() + add_fac[:, 1:, :]
return Gi
def create_GeMM(self, x, Gi):
Gishape = Gi.shape
padding = torch.zeros((x.shape[0], x.shape[1], 1), requires_grad=True, device=x.device)
x = torch.cat((padding, x), dim=2)
Gi = Gi + 1
Gi_flat = self.flatten_gemm_inds(Gi)
Gi_flat = Gi_flat.view(-1).long()
odim = x.shape
x = x.permute(0, 2, 1).contiguous()
x = x.view(odim[0] * odim[2], odim[1])
f = torch.index_select(x, dim=0, index=Gi_flat)
f = f.view(Gishape[0], Gishape[1], Gishape[2], -1)
f = f.permute(0, 3, 1, 2).contiguous() # 不加contiguous有时候会报错 - 中断训练
return f
def pad_gemm(self, m, xsz, device):
padded_gemm = torch.tensor(m.mesh_nb[:, 0:self.k - 1], device=device).float().requires_grad_()
padded_gemm = torch.cat((torch.arange(len(m.faces), device=device).float().unsqueeze(1), padded_gemm), dim=1)
padded_gemm = F.pad(padded_gemm, (0, 0, 0, xsz - len(m.faces)), "constant", 0)
padded_gemm = padded_gemm.unsqueeze(0)
return padded_gemm
2.2 网络框架
网络框架主要参考ConvNeXt
1:A ConvNet for the 2020s
- 主要借用了其中的
ConvNeXt Block
,通道可分离卷积 - LN - conv1x1up - GELU - conv1x1dn - 由于本文使用数据集较小,主要由几个Block串联组成分类网络
class TriCNN(nn.Module):
def __init__(self, dim_in, dims, classes_n=30):
super(TriCNN, self).__init__()
self.dims = dims[0:]
self.first_conv = FaceConv(dim_in, dims[0], k=4, groups=1, bias=True)
self.first_ln = nn.LayerNorm(dims[0], eps=1e-6)
self.conv1x1up = nn.Conv1d(dims[0], dims[0] * 2, 1)
self.act = nn.GELU()
self.conv1x1dn = nn.Conv1d(dims[0] * 2, dims[0], 1)
for i, dim in enumerate(self.dims[:]):
setattr(self, 'Block'.format(i), Block(dim, k=4))
self.last_ln = nn.LayerNorm(dims[-1], eps=1e-6)
# cls
self.gp = nn.AdaptiveAvgPool1d(1)
self.dp1 = nn.Dropout(0.5)
self.fc = nn.Linear(self.dims[-1], classes_n)
def forward(self, x, mesh):
x = x.permute(0, 2, 1).contiguous()
# 1.first Block
x = self.first_conv(x, mesh)
x = x.squeeze(-1)
x = x.permute(0, 2, 1).contiguous()
x = self.first_ln(x)
x = x.permute(0, 2, 1).contiguous()
x = self.conv1x1up(x)
x = self.act(x)
x = self.conv1x1dn(x)
# 2.Blocks
for i in range(len(self.dims) - 1):
x = getattr(self, 'Block'.format(i))(x, mesh)
# 3.final
x = x.squeeze(-1)
x = x.permute(0, 2, 1).contiguous()
x = self.last_ln(x)
x = x.permute(0, 2, 1).contiguous()
# 4.cls
x = self.gp(x)
x = x.view(-1, self.dims[-1])
x = self.dp1(x)
x = self.fc(x)
return x
class Block(nn.Module):
def __init__(self, dim, k=10, bias=True):
super(Block, self).__init__()
self.conv = FaceConv(dim, dim, groups=dim, k=k, bias=bias)
self.ln = nn.LayerNorm(dim,eps=1e-6)
self.conv1x1up = nn.Conv1d(dim, dim * 2, 1)
self.act = nn.GELU()
self.conv1x1dn = nn.Conv1d(dim * 2, dim, 1)
self.w = nn.Parameter(torch.zeros(1))
def forward(self, x, mesh):
identity = x
x = self.conv(x, mesh)
x = x.squeeze(-1)
x = x.permute(0, 2, 1).contiguous()
x = self.ln(x)
x = x.permute(0, 2, 1).contiguous()
x = self.conv1x1up(x)
x = self.act(x)
x = self.conv1x1dn(x)
x = x * self.w
x = x + identity
return x
三、基于CNN的网格分类
数据集是SHREC’11
可参考三角网格(Triangular Mesh)分类数据集 或 MeshCNN
3.1 分类结果
学习率还是有点高,权重波动很大,期间最高准确率是99.67
不得不提一句,其在网格分割上的准确率不如
UNet
形式的网络,也可能是没加入池化的锅…
3.2 全部代码
DataLoader代码请参考4:从零开始网格上的深度学习-1:输入篇(Pytorch)
import torch
import torch.nn as nn
import torch.nn.functional as F
from DataLoader_shrec11 import DataLoader
from DataLoader_shrec11 import Mesh
class TriCNN(nn.Module):
def __init__(self, dim_in, dims, classes_n=30):
super(TriCNN, self).__init__()
self.dims = dims[0:]
self.first_conv = FaceConv(dim_in, dims[0], k=4, groups=1, bias=True)
self.first_ln = nn.LayerNorm(dims[0], eps=1e-6)
self.conv1x1up = nn.Conv1d(dims[0], dims[0] * 2, 1)
self.act = nn.GELU()
self.conv1x1dn = nn.Conv1d(dims[0] * 2, dims[0], 1)
for i, dim in enumerate(self.dims[:]):
setattr(self, 'Block'.format(i), Block(dim, k=4))
self.last_ln = nn.LayerNorm(dims[-1], eps=1e-6)
# cls
self.gp = nn.AdaptiveAvgPool1d(1)
self.dp1 = nn.Dropout(0.5)
self.fc = nn.Linear(self.dims[-1], classes_n)
def forward(self, x, mesh):
x = x.permute(0, 2, 1).contiguous()
# 1.first Block
x = self.first_conv(x, mesh)
x = x.squeeze(-1)
x = x.permute(0, 2, 1).contiguous()
x = self.first_ln(x)
x = x.permute(0, 2, 1).contiguous()
x = self.conv1x1up(x)
x = self.act(x)
x = self.conv1x1dn(x)
# 2.Blocks
for i in range(len(self.dims) - 1):
x = getattr(self, 'Block'.format(i))(x, mesh)
# 3.final
x = x.squeeze(-1)
x = x.permute(0, 2, 1).contiguous()
x = self.last_ln(x)
x = x.permute(0, 2, 1).contiguous()
# 4.cls
x = self.gp(x)
x = x.view(-1, self.dims[-1])
x = self.dp1(x)
x = self.fc(x)
return x
class Block(nn.Module):
def __init__(self, dim, k=10, bias=True):
super(Block, self).__init__()
self.conv = FaceConv(dim, dim, groups=dim, k=k, bias=bias)
self.ln = nn.LayerNorm(dim,eps=1e-6)
self.conv1x1up = nn.Conv1d(dim, dim * 2, 1)
self.act = nn.GELU()
self.conv1x1dn = nn.Conv1d(dim * 2, dim, 1)
self.w = nn.Parameter(torch.zeros(1))
def forward(self, x, mesh):
identity = x
x = self.conv(x, mesh)
x = x.squeeze(-1)
x = x.permute(0, 2, 1).contiguous()
x = self.ln(x)
x = x.permute(0, 2, 1).contiguous()
x = self.conv1x1up(x)
x = self.act(x)
x = self.conv1x1dn(x)
x = x * self.w
x = x + identity
return x
class FaceConv(nn.Module):
"""
Face convolution with convolution region (参考 MeshCNN)
"""
def __init__(self, dim_in, dim_out, k, groups=1, bias=True):
super(FaceConv, self).__init__()
self.conv
2. 全连接神经网络与卷积神经网络的联系与区别。
全连接神经网络和卷积神经网络中的每一个节点就是一个神经元。
在全连接神经网络中,每相邻两层之间的节点都有边相连,于是会将每一层的全连接层中的节点组织成一列,这样方便显示连接结构。而对于卷积神经网络,相邻两层之间只有部分节点相连,为了展示每一层神经元的维度,一般会将每一层卷积层的节点组织成一个三维矩阵。
3.理解卷积计算。
以digit0为例,进行手工演算。
from sklearn.datasets import load_digits #小数据集8*8
digits = load_digits()
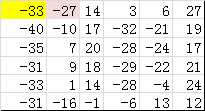
4.理解卷积如何提取图像特征。
读取一个图像;
以下矩阵为卷积核进行卷积操作;
显示卷积之后的图像,观察提取到什么特征。
1
0
-1
1
0
-1
1
0
-1
1
1
1
0
0
0
-1
-1
-1
-1
-1
-1
-1
8
-1
-1
-1
-1
卷积API
scipy.signal.convolve2d
tf.keras.layers.Conv2D
1
2
3
4
5
6
7
8
9
10
11
12
13
14
15
16
17
18
19
20
21
from
PIL
import
Image
import
matplotlib.pyplot as plt
import
numpy as np
from
scipy.signal
import
convolve2d
I
=
Image.
open
(r
‘C:Userslucas-lywDesktop img.jpg‘
)
L
=
I.convert(
‘L‘
)
pig
=
np.array(I)
pigg
=
np.array(L)
k1
=
np.array([[
1
,
0
,
-
1
], [
1
,
0
,
-
1
], [
1
,
0
,
-
1
]])
k2
=
np.array([[
1
,
1
,
1
], [
0
,
0
,
0
], [
-
1
,
-
1
,
-
1
]])
k3
=
np.array([[
-
1
,
-
1
,
-
1
], [
-
1
,
8
,
-
1
], [
-
1
,
-
1
,
-
1
]])
pig1
=
convolve2d(pigg, k1, boundary
=
‘symm‘
, mode
=
‘same‘
)
pig2
=
convolve2d(pigg, k2, boundary
=
‘symm‘
, mode
=
‘same‘
)
pig3
=
convolve2d(pigg, k3, boundary
=
‘symm‘
, mode
=
‘same‘
)
plt.matshow(pig1)
plt.matshow(pig2)
plt.matshow(pig3)
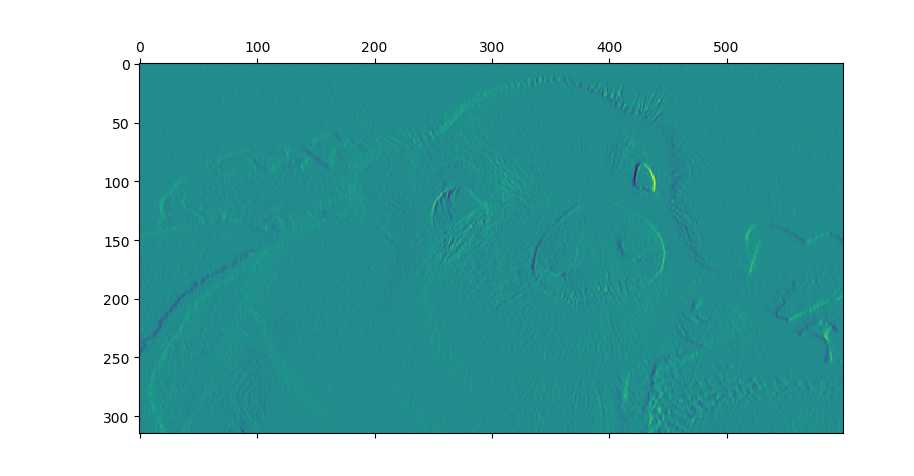
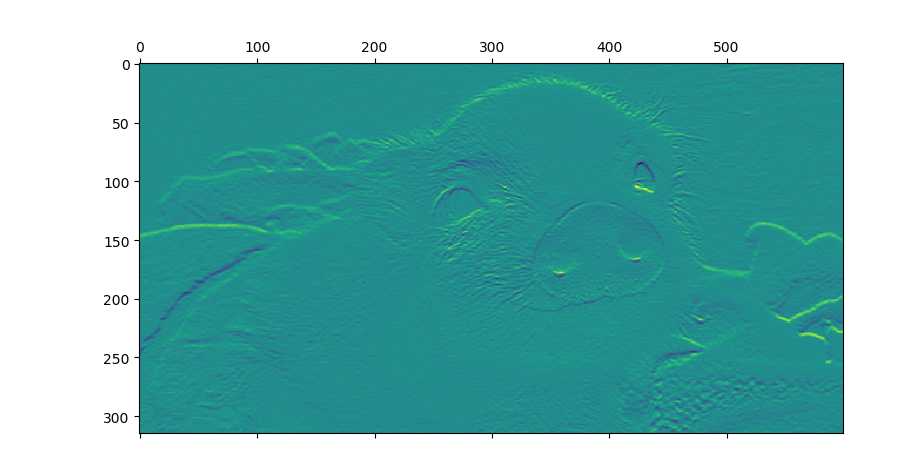
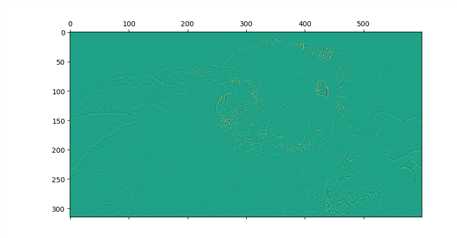
5. 安装Tensorflow,keras
以上是关于三维几何学习从零开始网格上的深度学习-2:卷积网络CNN篇(Pytorch)的主要内容,如果未能解决你的问题,请参考以下文章