基于参考点的非支配遗传算法-NSGA-III
Posted 流浪若相惜
tags:
篇首语:本文由小常识网(cha138.com)小编为大家整理,主要介绍了基于参考点的非支配遗传算法-NSGA-III相关的知识,希望对你有一定的参考价值。
上一篇我们讲了有关非支配遗传算法NSGA-III的非约束过程,接下来这一篇我们讲一下NSGA-III约束实现以及扩展自适应方法。同理,我们先列一下我们参考的博客、代码以及论文。
文章目录
参考博客
platEMO
基于参考点的非支配遗传算法-NSGA-III(一)
参考论文
因参考论文较多,此直接给予所有论文,请点击下载。论文中重要的部分已做了注解和标注。
- Y. Tian, R. Cheng, X.Y. Zhang, and Y.C. Jin, “PlatEMO: A MATLAB platform for evolutionary multi‐objective optimization [educational forum],” IEEE Computational Intelligence Magazine, 2017, 12(4): 73‐87.
- K. Deb, H. Jain . An Evolutionary Many-Objective Optimization Algorithm Using Reference Point-Based Nondominated Sorting Approach, Part I: Solving Problems With Box Constraints[J]. IEEE Transactions on Evolutionary Computation, 2014, 18(4):577-601.
- Y. Tian , X. Xiang , X. Zhang , et al. Sampling Reference Points on the Pareto Fronts of Benchmark Multi-Objective Optimization Problems[C]. 2018 IEEE Congress on Evolutionary Computation (CEC). IEEE, 2018.
- X. Zhang, Y. Tian, R. Cheng, and Y. Jin, “An efficient approach to nondominated sorting for evolutionary multiobjective optimization,” IEEE Trans. Evol. Comput., vol. 19, no. 2, pp. 201–213, Apr. 2015.
- I. Das and J. Dennis, “Normal-boundary intersection: A new method
for generating the Pareto surface in nonlinear multicriteria optimization
problems,” SIAM J. Optimization, vol. 8, no. 3, pp. 631–657, 1998. - K. Deb, R.B. Agrawal, Simulated binary crossover for continuous search space, Complex Systems. 9 (1995) 115–148.
在原有参考论文的基础上,本博客主要参考论文 - H. Jain,K. Deb, A. Pratap, S. An Evolutionary Many-Objective Optimization Algorithm Using Reference-point Based Non-dominated Sorting Approach, Part II: Handling Constraints and Extending to an Adaptive Approach, IEEE Trans. Evol. Comput.,18(4):602-622, 2014.
参考代码
NSGA-III约束实现
在NSGA-II中我们提到,我们是在划分等级的时候实现了考虑约束的多目标优化 (详细请参考:多目标非支配排序遗传算法-NSGA-II(一)),主要包括以下几种情况:
主要实现代码如下:
function domMat = calcDominationMatrix(nViol, violSum, obj)
% Function: domMat = calcDominationMatrix(nViol, violSum, obj)
% Description: Calculate the domination maxtrix which specified the domination
% releation between two individual using constrained-domination.
%
% Return:
% domMat(N,N) : domination matrix
% domMat(p,q)=1 : p dominates q
% domMat(p,q)=-1 : q dominates p
% domMat(p,q)=0 : non dominate
%
% Copyright 2011 by LSSSSWC
% Revision: 1.0 Data: 2011-07-13
%*************************************************************************
N = size(obj, 1); %行个数(种群)
numObj = size(obj, 2);% 列个数(目标函数)
domMat = zeros(N, N);
for p = 1:N-1
for q = p+1:N
%*************************************************************************
% 1. p and q are both feasible
%*************************************************************************
%两个个体约束均为0的情况
if(nViol(p) == 0 && nViol(q)==0)
pdomq = false;
qdomp = false;
for i = 1:numObj
if( obj(p, i) < obj(q, i) ) % objective function is minimization!
pdomq = true; % p支配q
elseif(obj(p, i) > obj(q, i))
qdomp = true;
end
end
%只有两个对比个体所有目标函数都为1或0是才会产生支配,若存在不同目标函数个体支配相反情况,则两个个体不存在支配与被支配关系
if( pdomq && ~qdomp )
domMat(p, q) = 1; %表示p支配q
elseif(~pdomq && qdomp )
domMat(p, q) = -1; %表示q支配p
end
%*************************************************************************
% 2. p is feasible, and q is infeasible
%*************************************************************************
elseif(nViol(p) == 0 && nViol(q)~=0)
domMat(p, q) = 1;
%*************************************************************************
% 3. q is feasible, and p is infeasible
%*************************************************************************
elseif(nViol(p) ~= 0 && nViol(q)==0)
domMat(p, q) = -1;
%*************************************************************************
% 4. p and q are both infeasible
%*************************************************************************
else
if(violSum(p) < violSum(q))
domMat(p, q) = 1;
elseif(violSum(p) > violSum(q))
domMat(p, q) = -1;
end
end
end
end
在NSGA-II中实现此过程是在选择之前实现的,在NSGA-III约束实现时,其原理上是一样的只是在两个地方进行了修改,我这里直接截图原文,然后再每一部分具体解释一下。
Modifications in the Elitist Selection Operator
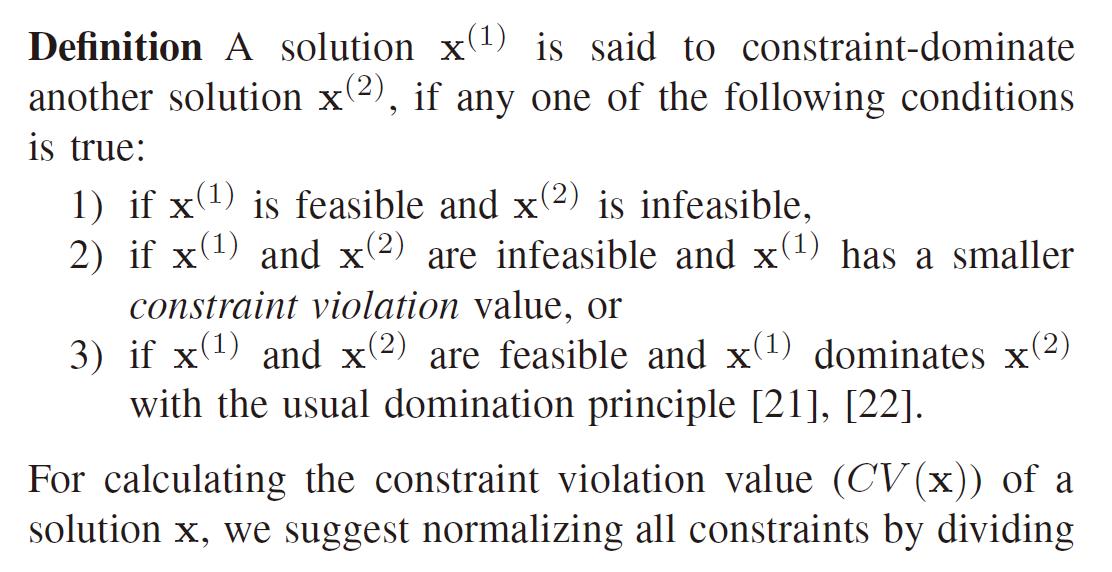
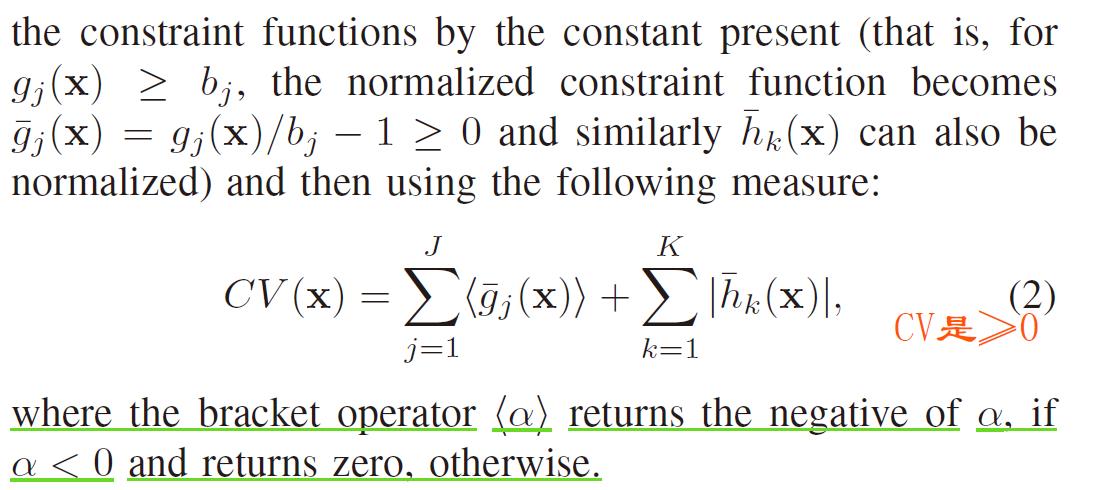
其实,我们在NSGA-II中也是采用了此思想,不同的是我们在NSGA-II中令违背约束的条件个数=0,而此采用的是
C
V
(
x
)
=
∑
j
=
1
J
<
g
‾
j
(
x
)
>
+
∑
k
=
1
K
∣
h
‾
k
(
x
)
∣
(1)
CV(\\bf x)=\\sum_j=1^J<\\overline g_j(\\bf x)>+\\sum_k=1^K|\\overline h_k(\\bf x)| \\tag 1
CV(x)=j=1∑J<gj(x)>+k=1∑K∣hk(x)∣(1)其中,运算符的作用其实就是让
C
V
≥
0
CV \\geq 0
CV≥0, 且让
g
‾
j
\\overline g_j
gj变为
−
g
‾
j
-\\overline g_j
−gj 。我们在往下思考一下,
C
V
≥
0
CV \\geq 0
CV≥0意味着什么?我们不妨列举一个test problem来说明此问题。例如C1_DTLZ1的约束为:
c
(
x
)
=
1
−
f
M
(
x
)
0.6
−
∑
i
=
1
M
−
1
f
i
(
x
)
0.5
≥
0
(2)
c(\\bf x)=1-\\fracf_M(\\bf x)0.6-\\sum_i=1^M-1\\fracf_i(\\bf x)0.5 \\geq 0 \\tag 2
c(x)=1−0.6fM(x)−i=1∑M−10.5fi(x)≥0(2)按照文中所说,也就是让
c
(
x
)
≤
0
c(\\bf x) \\leq 0
c(x)≤0 ,那么(2)式转为:
−
c
(
x
)
=
f
M
(
x
)
0.6
+
∑
i
=
1
M
−
1
f
i
(
x
)
0.5
−
1
≤
0
(3)
-c(\\bf x)=\\fracf_M(\\bf x)0.6+\\sum_i=1^M-1\\fracf_i(\\bf x)0.5-1 \\leq 0 \\tag 3
−c(x)=0.6fM(x)+i=1∑M−10.5fi(x)−1≤0(3)
(2)式跟(3)式所要表示的含义是一致的,但运算符
<
>
<>
<>操作后,会使得
C
V
≥
0
CV \\geq 0
CV≥0,这与原有的constraints相反,也就说所谓的constraint violation含义。也就说在选择等操作所用的约束条件是违背的,也就是所谓的infeasible solution。
需要注意的是,文中的α已经是<>之后的了,运算符之后,feasible solution constraints 已经变为了<0, 也就是说将<0的约束(feasible 约束)的违背约束设置为0。
Modification in Creation of Offspring Population
其实,我们仅需要理解下边这段话就可以了:
意思就是说,我们之前仅在NSGA-II选择的划分等级时考虑了,但是并没有在父代生成子代中考虑这种支配关系,这样我们的整个过程如下伪代码:
代码实现:
MatingPool = TournamentSelection(2,N,sum(max(0,PopCon),2));
function index = TournamentSelection(K,N,varargin)
varargin = cellfun(@(S) reshape(S,[],1),varargin,'UniformOutput',false);% return by the form of cell type
% For example
% A = cellfun(@(x) x(1:3),C,'UniformOutput',false)
% A = 1x5 cell
% 'Mon' 'Tue' 'Wed' 'Thu' 'Fri'
% reshape restruct array
[~,rank] = sortrows([varargin:]);%默认第一列排序,rank种群排列的索引位置
[~,rank] = sort(rank);%升序排列,rank种群原要素的排序位置
Parents = randi(length(varargin1),K,N);%随机产生二元锦标赛个体
[~,best] = min(rank(Parents),[],1);%按列排序
index = Parents(best+(0:N-1)*K);%种群个体索引位置
end
对于TournamentSelection()我们可以这样去理解,这个函数是为了说明在父代生成子代过程时发生的,需要进行条件约束下的支配选择。这个函数的关键点就在于对 s u m ( m a x ( 0 , P o p C o n ) , 2 ) sum(max(0,PopCon),2) sum(max(0,PopCon),2) 的理解,它实则就是实现我们前边所说的运算符 < > <> <>。我们前边也介绍了PopCon是符合约束的,那么对于feasible solution 这个操作的结果肯定是0,而对于infeasible solution来说肯定是sum(PopCon)。那么在进入函数二元锦标赛选择时,我们的排序是根据sum(PopCon)从小到大排序的,那么feasible solution肯定是在最前边的,也就是说feasible solution的 sum(PopCon)(约束违背)要小于infeasible solution的,对于两个feasible solution我们可以任一选择一个,对于两个infeasible solution来说谁的sum(PopCon)小,则优选谁。
function [FrontNo,MaxFNo] = NDSort(varargin)
PopObj = varargin1;
[N,M] = size(PopObj);
if nargin == 2
nSort = varargin2;
else
PopCon = varargin2;
nSort = varargin3;%reference points count
Infeasible = any(PopCon>0,2);%any one PopCon>0 will be a infeasible solution
PopObj(Infeasible,:) = repmat(max(PopObj,[],1),sum(Infeasible),1) + repmat(sum(max(0,PopCon(Infeasible,:)),2),1,M);
%不可行解一定obj一定是大于所有解的最大的,这样的就可以得到最小化问题中feasible solution obj一定小于
%infeasible solution obj,然后对于两个feasible solution仅需对比obj,对于两个infeasible solution 仅需对比PopCon
end
if M < 5 || N < 500
% Use efficient non-dominated sort with sequential search (ENS-SS)
[FrontNo,MaxFNo] = ENS_SS(PopObj,nSort);
在选择过程中,重点理解
Infeasible = any(PopCon>0,2);
PopObj(Infeasible,:) = repmat(max(PopObj,[],1),sum(Infeasible),1) + repmat(sum(max(0,PopCon(Infeasible,:)),2),1,M);
这两句的意思就可以实现feasible solution以及infeasible solution的所有组合情况,对于feasible 我们仍然是对于其目标函数的对比,对于infeasible solution我们可以通过sum(PopCon),而对于feasible solution以及infeasible solution来讲,我们
P
o
p
O
b
j
(
I
n
f
e
a
s
i
b
l
e
,
:
)
=
r
e
p
m
a
t
(
m
a
x
(
P
o
p
O
b
j
,
[
]
,
1
)
,
s
u
m
(
I
n
f
e
a
s
i
b
l
e
)
,
1
)
+
r
e
p
m
a
t
(
s
u
m
(
m
a
x
(
0
,
P
o
p
C
o
n
(
I
n
f
e
a
s
i
b
l
e
,
:
)
)
,
2
)
,
1
,
M
)
;
PopObj(Infeasible,:) = repmat(max(PopObj,[],1),sum(Infeasible),1) + repmat(sum(max(0,PopCon(Infeasible,:)),2),1,M);
PopObj(Infeasible,:)=repmat(max(PopObj,[],1),sum(Infeasible),1)+repmat(sum(max(0,PopCon(Infeasible,:)),2),1,M);就可以看出,infeasible solution即使再小,也是大于feasible solution中目标函数最大的那个(我们这里采用min function),即任一一个infeasible solution的“目标函数值”要大于所有的feasible solution。这样我们就实现了父代生成子代、种群选择过程中的约束优选算法。
为庆祝此役的胜利,附上C2_DTLZ2 test problem的运行结果:
以上是关于基于参考点的非支配遗传算法-NSGA-III的主要内容,如果未能解决你的问题,请参考以下文章