Temporal Fusion Transformer (TFT) 各模块功能和代码解析(pytorch)
Posted 程序媛小哨
tags:
篇首语:本文由小常识网(cha138.com)小编为大家整理,主要介绍了Temporal Fusion Transformer (TFT) 各模块功能和代码解析(pytorch)相关的知识,希望对你有一定的参考价值。
Temporal Fusion Transformer (TFT) 各模块功能和代码解析(pytorch)
文章目录
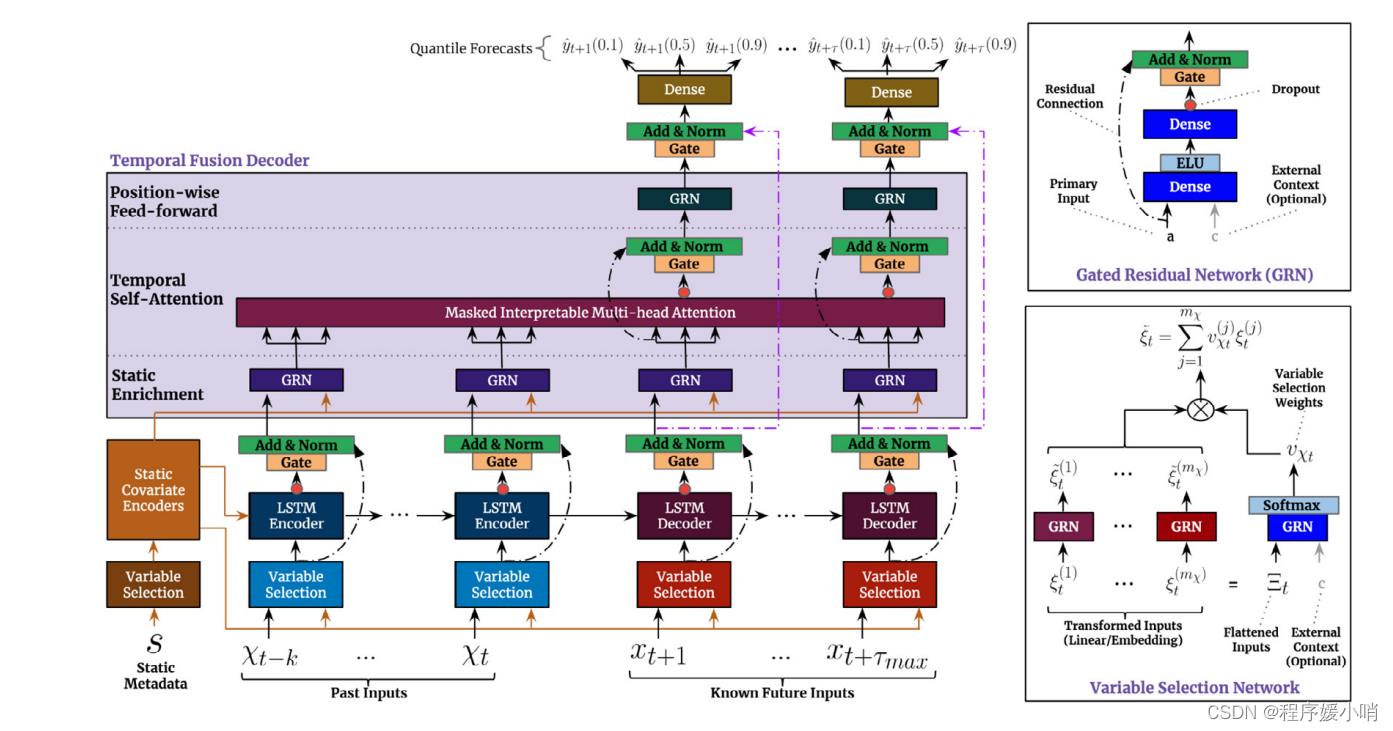
GLU(Gated Linear Unit)模块
该模块包括一个前向传播函数forward和一个参数初始化函数init_weights。
GLU是一种多层感知器(MLP)结构,由两个子层组成,分别为全连接层和sigmoid门。
全连接层有两个,一个作为输入门,另一个作为忘记门。sigmoid门负责控制两个门的权重,使得在两个门中仅有一个门起作用。
如果激活函数activation不是None,则在输出门之前添加一个激活函数。
在init_weights函数中,使用了Xavier均匀分布初始化权重和零初始化偏置。
class GatedLinearUnit(nn.Module):
def __init__(self, input_size,
hidden_layer_size,
dropout_rate,
activation = None):
super(GatedLinearUnit, self).__init__()
self.input_size = input_size
self.hidden_layer_size = hidden_layer_size
self.dropout_rate = dropout_rate
self.activation_name = activation
if self.dropout_rate:
self.dropout = nn.Dropout(p=self.dropout_rate)
self.W4 = torch.nn.Linear(self.input_size, self.hidden_layer_size)
self.W5 = torch.nn.Linear(self.input_size, self.hidden_layer_size)
if self.activation_name:
self.activation = getattr(nn, self.activation_name)()
self.sigmoid = nn.Sigmoid()
self.init_weights()
def init_weights(self):
for n, p in self.named_parameters():
if 'bias' not in n:
torch.nn.init.xavier_uniform_(p)
# torch.nn.init.kaiming_normal_(p, a=0, mode='fan_in', nonlinearity='sigmoid')
elif 'bias' in n:
torch.nn.init.zeros_(p)
def forward(self, x):
if self.dropout_rate:
x = self.dropout(x)
if self.activation_name:
output = self.sigmoid(self.W4(x)) * self.activation(self.W5(x))
else:
output = self.sigmoid(self.W4(x)) * self.W5(x)
return output
GRN(Gated Residual Network)门控残差网络
这段代码实现了一个门控残差网络(Gated Residual Network),它是Temporal Fusion Transformer中的一个组成部分。
该类的初始化方法包含了多个参数:
hidden_layer_size
:隐层的维度大小。
input_size
:输入向量的维度大小。默认为None,若未指定则使用hidden_layer_size作为输入向量的维度大小。
output_size
:输出向量的维度大小。默认为None,若未指定则输出向量的维度大小与隐层的维度大小相同。
dropout_rate
:dropout概率。
additional_context
:附加的上下文信息。默认为None。
return_gate
:是否返回门控向量。默认为False。
在初始化方法中,首先定义了该类所需要用到的线性层,其中包括self.W1、self.W2和self.W3(若有附加的上下文信息)。接着,根据是否指定了输出向量的维度大小,定义了一个门控加和归一化网络(GateAddNormNetwork),其输入向量的维度大小取决于self.hidden_layer_size和self.output_size的大小关系。最后,对模型中的参数进行了初始化。
在该类的前向传播方法中,如果有附加的上下文信息,则将输入向量和上下文信息分别输入self.W2和self.W3中,并将它们的输出进行求和,并通过激活函数(这里使用了ELU)进行激活。接着,将该结果输入self.W1中,并将其输出通过门控加和归一化网络进行处理。如果指定了输出向量的维度大小,则将输入向量通过self.skip_linear进行线性转换,并将结果与门控加和归一化网络的输出相加后得到最终的输出向量;否则,直接将输入向量与门控加和归一化网络的输出相加后得到最终的输出向量。最后返回输出向量。
class GatedResidualNetwork(nn.Module):
def __init__(self,
hidden_layer_size,
input_size = None,
output_size = None,
dropout_rate = None,
additional_context = None,
return_gate = False):
super(GatedResidualNetwork, self).__init__()
self.hidden_layer_size = hidden_layer_size
self.input_size = input_size if input_size else self.hidden_layer_size
self.output_size = output_size
self.dropout_rate = dropout_rate
self.additional_context = additional_context
self.return_gate = return_gate
self.W1 = torch.nn.Linear(self.hidden_layer_size, self.hidden_layer_size)
self.W2 = torch.nn.Linear(self.input_size, self.hidden_layer_size)
if self.additional_context:
self.W3 = torch.nn.Linear(self.additional_context, self.hidden_layer_size, bias = False)
if self.output_size:
self.skip_linear = torch.nn.Linear(self.input_size, self.output_size)
self.glu_add_norm = GateAddNormNetwork(self.hidden_layer_size,
self.output_size,
self.dropout_rate)
else:
self.glu_add_norm = GateAddNormNetwork(self.hidden_layer_size,
self.hidden_layer_size,
self.dropout_rate)
self.init_weights()
def init_weights(self):
for name, p in self.named_parameters():
if ('W2' in name or 'W3' in name) and 'bias' not in name:
torch.nn.init.kaiming_normal_(p, a=0, mode='fan_in', nonlinearity='leaky_relu')
elif ('skip_linear' in name or 'W1' in name) and 'bias' not in name:
torch.nn.init.xavier_uniform_(p)
# torch.nn.init.kaiming_normal_(p, a=0, mode='fan_in', nonlinearity='sigmoid')
elif 'bias' in name:
torch.nn.init.zeros_(p)
def forward(self, x):
if self.additional_context:
x, context = x
#x_forward = self.W2(x)
#context_forward = self.W3(context)
#print(self.W3(context).shape)
n2 = F.elu(self.W2(x) + self.W3(context))
else:
n2 = F.elu(self.W2(x))
#print('n2 shape '.format(n2.shape))
n1 = self.W1(n2)
#print('n1 shape '.format(n1.shape))
if self.output_size:
output = self.glu_add_norm(n1, self.skip_linear(x))
else:
output = self.glu_add_norm(n1, x)
#print('output shape '.format(output.shape))
return output
Transformer经典模块
Add&Normalize模块
transformer在进行self-Attention之后会进行一个layerNormalization 【将数据统一到固定区间内】
其中又分为batchNormalization和layerNormalization,batchNormalization 即按照batch维度化成均值为0标准差为1的数据
Layer则是纵向将每次的向量数据进行归一化
残差作用:加入未学习的原向量使得到的结果的效果至少不弱于原来的结果
蓝色圆圈标注的“add”是什么呢?好像初始注意力机制(如下图)中并没有add呀??
如图所示,在self-attention中求出 Z ( i ) = ∑ j = 1 T a i j v ( j ) Z^\\left( i \\right)=\\sum_j=1^Ta_ijv^\\left( j \\right) Z(i)=∑j=1Taijv(j)后直接将Z放入全连接层中,而transformer又经过了add&Norm步骤 ,这个add是什么呢???
其实add就是在Z的基础上加了一个残差块X,加入残差块X的目的是为了防止在深度神经网络训练中发生退化问题,退化的意思就是深度神经网络通过增加网络的层数,Loss逐渐减小,然后趋于稳定达到饱和,然后再继续增加网络层数,Loss反而增大。这个Add具体操作如下:其实就是将self-attention的output+input作为新的output,如下图:
关于首图所标记的2,Add&Norm这一过程指什么呢?
就是指对新的output做标准化,也就是上图的,对a+b做标准化
FeedForward:FeedForward又是什么呢?好像图二(注意力机制)中这里应该是全连接层(FC)呀?❓❓❓
先说一下FeedForward是什么?其实FeedForward是由全连接层(FC)与激活ReLu组成的结构,其实和bp神经网络结构差不多,输入:Add&Norm的输出(记作:Z’(i) ), FC:全连接层
接下来再说一下为什么要用FeedForward呢?不用单纯的FC呢?
其实主要还是想提取更深层次的特征,在Multi-Head Attention中,主要是进行矩阵乘法,即都是线性变换,而线性变换的学习能力不如非线性变换的学习能力强,我们希望通过引入ReLu激活函数,使模型增加非线性成分,强化学习能力。
回到这个模块来,它的输入是 x x x 和 s k i p skip skip 两个张量,其中 x x x 是输入张量, s k i p skip skip 是跳跃连接(skip connection),用于保留之前层的信息,防止信息丢失。
在这个模块中,先通过 Gated Linear Unit(GLU)对 x x x 进行变换,然后将变换后的结果和 s k i p skip skip 进行加和操作,再通过 Layer Normalization 进行归一化,得到输出张量。
GLU 的作用是将 x x x 变换为与之相同维度的张量,并同时生成一个门控向量。门控向量的每个元素都是介于 0 和 1 之间的实数,用于控制该元素对最终结果的贡献程度。这里的 GLU 实现了类似于 Highway Network 的效果,即能够选择性地保留或抛弃某些信息。
Layer Normalization 的作用是对张量的每个特征维度进行归一化,使得每个特征维度上的均值为 0,方差为 1,从而加快收敛速度、提高泛化能力。
最终,这个门控加和归一化网络将 x x x 和 s k i p skip skip 合并为一个输出张量,其中 s k i p skip skip 是经过跳跃连接保留下来的信息, x x x 是经过 GLU 变换后的信息,经过 Layer Normalization 归一化后得到的输出张量。
class GateAddNormNetwork(nn.Module):
def __init__(self, input_size,
hidden_layer_size,
dropout_rate,
activation = None):
super(GateAddNormNetwork, self).__init__()
self.input_size = input_size
self.hidden_layer_size = hidden_layer_size
self.dropout_rate = dropout_rate
self.activation_name = activation
self.GLU = GatedLinearUnit(self.input_size,
self.hidden_layer_size,
self.dropout_rate,
activation = self.activation_name)
self.LayerNorm = nn.LayerNorm(self.hidden_layer_size)
def forward(self, x, skip):
output = self.LayerNorm(self.GLU(x) + skip)
return output
Scaled Dot-Product Attention 模块
在实际应用中,经常会用到 Attention 机制,其中最常用的是 Scaled Dot-Product Attention,它是通过计算query和key之间的点积 来作为 之间的相似度。
Scaled 指的是 Q和K计算得到的相似度 再经过了一定的量化,具体就是 除以 根号下K_dim;
Dot-Product 指的是 Q和K之间 通过计算点积作为相似度;
Mask 可选择性 目的是将 padding的部分 填充负无穷,这样算softmax的时候这里就attention为0,从而避免padding带来的影响.
在TFT里面套用原始Transformer架构,实现了一个Scaled Dot-Product Attention。
该类定义了一个基于nn.Module的自定义PyTorch模块,包含了初始化函数和前向传递函数。S
caledDotProductAttention中的主要计算过程是计算query和key之间的点积,然后进行softmax归一化,最后加权求和得到输出。具体地,该类的初始化函数接受两个参数:dropout和scale。
dropout参数指定了在softmax之前要应用多少dropout,
而scale参数指定是否应该缩放点积结果。
该类的前向传递函数接受四个参数:q,k,v和mask。
其中,q,k和v是查询、键和值的输入向量,mask是一个可选的屏蔽向量,用于屏蔽输入中的某些位置。
在前向函数中,首先计算 q 和 k 的点积得到注意力矩阵 attn。
如果模块被初始化为缩放模式,则在计算点积时会将结果除以 k 向量长度的平方根,以缩小点积结果的值域,避免梯度消失或梯度爆炸的问题。
如果 mask 不为空,则在 attn 中填充 -1e9,以便在 softmax 操作中产生 0 的概率。
然后,通过 softmax 函数将注意力矩阵归一化为概率分布,并应用 dropout 操作以防止过拟合。最后,通过点积计算得到输出向量 output。
在输出时,函数返回输出向量 output 和注意力矩阵 attn。
class ScaledDotProductAttention(nn.Module):
def __init__(self, dropout = 0, scale = True):
super(ScaledDotProductAttention, self).__init__()
self.dropout = nn.Dropout(p=dropout)
self.softmax = nn.Softmax(dim = 2)
self.scale = scale
def forward(self, q, k, v, mask = None):
#print('---Inputs----')
#print('q: '.format(q[0]))
#print('k: '.format(k[0]))
#print('v: '.format(v[0]))
attn = torch.bmm(q, k.permute(0,2,1))
#print('first bmm')
#print(attn.shape)
#print('attn: '.format(attn[0]))
if self.scale:
dimention = torch.sqrt(torch.tensor(k.shape[-1]).to(torch.float32))
attn = attn / dimention
# print('attn_scaled: '.format(attn[0]))
if mask is not None:
#fill = torch.tensor(-1e9).to(DEVICE)
#zero = torch.tensor(0).to(DEVICE)
attn = attn.masked_fill(mask == 0, -1e9)
# print('attn_masked: '.format(attn[0]))
attn = self.softmax(attn)
#print('attn_softmax: '.format(attn[0]))
attn = self.dropout(attn)
output = torch.bmm(attn, v)
return output, attn
InterpretableMultiHeadAttention可解释的多头注意力机制
TFT参照原始Transformer架构,实现了一个可解释的多头注意力机制,即Interpretable Multi-Head Attention。
在初始化函数中,定义了注意力头的个数n_head、输入特征的维度d_model和临时的q、k、v的维度d_k、d_q、d_v。同时,通过nn.Linear函数定义了q、k、v的线性层和v的线性层的组合,还定义了缩放点积注意力的ScaledDotProductAttention层以及输出的全连接层w_h,并初始化了权重。
在前向函数中,首先对输入的q、k、v分别通过线性层得到q_i、k_i、v_i,再利用ScaledDotProductAttention层计算出每个注意力头对应的权重矩阵和输出矩阵,并对输出矩阵进行dropout操作。然后,将多个头的输出按照最后一个维度进行拼接,再通过均值池化得到最终的输出矩阵,最后通过全连接层和dropout操作得到最终的输出和注意力权重矩阵,并将它们返回。
需要注意的是,在计算每个注意力头的权重矩阵时,利用了缩放点积注意力。该注意力的计算过程包括三个步骤:1)计算q和k的点积,即attn = torch.bmm(q, k.permute(0,2,1));2)如果需要进行缩放,则除以根号下d_k;3)将得到的结果进行softmax操作得到注意力矩阵。同时,在计算注意力矩阵时,还可以通过mask参数实现对无效位置的屏蔽。
class InterpretableMultiHeadAttention(nn.Module):
def __init__(self, n_head, d_model, dropout):
super(InterpretableMultiHeadAttention, self).__init__()
self.n_head = n_head
self.d_model = d_model
self.d_k = self.d_q = self.d_v = d_model // n_head
self.dropout = nn.Dropout(p=dropout)
self.v_layer = nn.Linear(self.d_model, self.d_v, bias = False)
self.q_layers = nn.ModuleList([nn.Linear(self.d_model, self.d_q, bias = False)
for _ in range(self.n_head)])
self.k_layers = nn.ModuleList([nn.Linear(self.d_model, self.d_k, bias = False)
for _ in range(self.n_head)])
self.v_layers = nn.ModuleList([self.v_layer for _ in range(self.n_head)])
self.attention = ScaledDotProductAttention()
self.w_h = nn.Linear(self.d_v, self.d_model, bias = False)
self.init_weights()
def init_weights(self):
for name, p in self.named_parameters():
if 'bias' not in name:
torch.nn.init.xavier_uniform_(p)
# torch.nn.init.kaiming_normal_(p, a=0, mode='fan_in', nonlinearity='sigmoid')
else:
torch.nn.init.zeros_(p)
def forward(self, q, k, v, mask = None):
heads = []
attns = []
for i in range(self.n_head):
qs = self.q_layers[i](q)
ks = self.k_layers[i](k)
vs = self.v_layers[i](v)
#print('qs layer: '.format(qs.shape))
head, attn = self.attention(qs, ks, vs, mask)
#print('head layer: '.format(head.shape))
#print('attn layer: '.format(attn.shape))
head_dropout = self.dropout(head)
heads.append(head_dropout)
attns.append(attn)
head = torch.stack(heads, dim = 2) if self.n_head > 1 else heads[0]
#print('concat heads: '.format(head.shape))
#print('heads : '.format(0, head[0,0,Ellipsis]))
attn = torch.stack(attns, dim = 2)
#print('concat attn: '.format(attn.shape))
outputs = torch.mean(head, dim = 2) if self.n_head > 1 else head
#print('outputs mean: '.format(outputs.shape))
#print('outputs mean : '.format(0, outputs[0,0,Ellipsis]))
outputs = self.w_h(outputs)
outputs = self.dropout(outputs)
return outputs, attn
Variable Selection Network 变量选择网络
这段代码是 Temporal Fusion Transformer 中 VariableSelectionNetwork 的前向传递方法。
VariableSelectionNetwork 的目的是选择哪些变量对于当前时间步骤的预测是最重要的。这里有两种情况:使用静态上下文(只有输入嵌入)和使用非静态上下文(输入嵌入和静态上下文)。
在这个方法中,如果使用了非静态上下文,输入会分成嵌入和静态上下文两个部分。
首先,使用 GatedResidualNetwork 对嵌入进行非线性转换,
然后使用 Softmax 函数将其转换为稀疏权重。
接着,对于每个要预测的特征,使用 GatedResidualNetwork 对嵌入进行非线性转换,将其与稀疏权重相乘,以生成一组经过转换的特征,最后将这些特征相加以生成最终
以上是关于Temporal Fusion Transformer (TFT) 各模块功能和代码解析(pytorch)的主要内容,如果未能解决你的问题,请参考以下文章