R语言实战:机器学习与数据分析源代码5
Posted 白马负金羁
tags:
篇首语:本文由小常识网(cha138.com)小编为大家整理,主要介绍了R语言实战:机器学习与数据分析源代码5相关的知识,希望对你有一定的参考价值。
本文辑录了《R语言实战——机器学习与数据分析》(电子工业出版社2016年出版)一书第6章至第7章前半部分(至136页)之代码。本书引言请见如下链接:
http://blog.csdn.net/baimafujinji/article/details/51596171
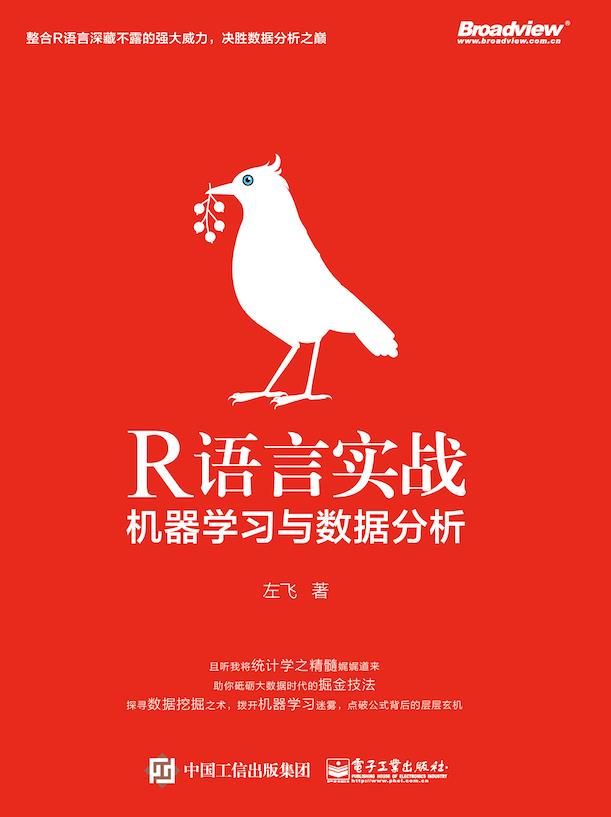
内容简介:本书系统地介绍了统计分析和机器学习领域中最为重要和流行的多种技术及它们的基本原理,在详解有关算法的基础上,结合大量R语言实例演示了这些理论在实践中的使用方法。具体内容被分成三个部分,即R语言编程基础、基于统计的数据分析方法以及机器学习理论。统计分析与机器学习部分又具体介绍了包括参数估计、假设检验、极大似然估计、非参数检验方法(包括列联分析、符号检验、符号秩检验等)、方差分析、线性回归(包括岭回归和Lasso方法)、逻辑回归、支持向量机、聚类分析(包括K均值算法和EM算法)和人工神经网络等内容。同时,统计理论的介绍也为深化读者对于后续机器学习部分的理解提供了很大助益。知识结构和阅读进度的安排上既兼顾了循序渐进的学习规律,亦统筹考虑了夯实基础的必要性
网上书店地址:
电子工业出版社官网
中国互动出版网China-pub
京东商城(1)
京东商城(2)
Chapter 6
P100~101
data(geyser, package = "MASS")
geyser
data = read.table("c:/car.txt", header=TRUE, quote="\\"")
data[1:2,]
mode(data)
P102
names(data)
dim(data)
data$lp100km
lp100km
attach(data)
lp100km
detach(data)
lp100km
data.fwf = read.fwf("c:/cities.txt", widths=c(7,7,7),
+ col.names=c("city","latitude","longitude"))
data.fwf
P103~104
data.excel = read.delim("clipboard")
data_excel[1:2,]
channel = odbcConnectExcel2007("c:/car.xlsx")
sqlTables(channel)
data_excel2 = sqlFetch(channel, "Sheet1")
data_excel2 = sqlQuery(channel, "select * from[Sheet1$]")
close(channel)
data_excel2[1:2,]
data_spss = read.spss("c:/car.sav", to.data.frame = T)
data_spss[1:2,]
P105
baseURL = "http://data.worldbank.org/indicator/NY.GDP.PCAP.CD/
+ countries/1W?display=default"
baseURL = gsub("\\\\n","",baseURL)
table = readhtmlTable(baseURL, header = TRUE, which = 1)
table = table[, 1:5]
names(table) = c("country", "2011", "2012", "2013", "2014")
table[c(40,95,71,11),]
P106~107
channel = odbcConnectAccess2007("c:/car.accdb")
data_access = sqlFetch(channel, "racv")
close(channel)
data_access[1:2,]
library(RJDBC)
con <- dbConnect(RSQLite::SQLite(),"C:/car.db")
dbListTables(con)
data_SQLite <- dbGetQuery(con, "select * from racv")
data_SQLite[1:2, ]
P108
car = file("d:/car.txt")
cat("Make lp100km mass.kg List.price",
+ "\\"Alpha Romeo\\" 9.5 1242 38500",
+ "\\"Audi A3\\" 8.8 1160 38700", file = car, sep = "\\n")
close(car)
data = USArrests[1:10,]
write.table(data, file = "c:/data.txt", col.names = T, quote = F)
read.table("c:/data.txt", header = T, row.names= 1)
data2 = read.table("c:/data.txt", header = T, row.names= 1)
write.csv(data2, file = "c:/data.csv", row.names = T, quote = F)
data.csv = read.csv("c:/data.csv", header = T, row.names = 1)
P111
ufc <- read.csv("c:/ufc.csv")
str(ufc)
table(ufc$species)
table(ufc$species,ufc$position)
mean(ufc$dbh.cm)
median(ufc$dbh.cm)
sd(ufc$dbh.cm)
P112
tapply(ufc$dbh.cm, ufc$species, mean)
tapply(ufc$dbh.cm, ufc$species, median)
tapply(ufc$dbh.cm, ufc$species, sd)
library(lattice)
xyplot(height.m ~ dbh.cm | species, data = ufc)
xyplot(height.m ~ dbh.cm, groups = species,
+ auto.key = list(space="right"), data = ufc)
P113~114
US_data = USArrests[1:10,]
US_data
names(US_data)
names(US_data) = c("MURDER","ASSAULT","URBANPOP","RAPE")
names(US_data)
names(US_data)[3] = "UrbanPop"
names(US_data)
dimnames(US_data)[[2]]
dimnames(US_data)[[1]]
dimnames(US_data)[[1]][1:3] = c("Alb", "Als", "Arz")
dimnames(US_data)[[1]][6:8] = c("Col", "Cnt", "Del")
dimnames(US_data)[[1]]
P115
air_data = airquality[1:7,1:4]
is.na(air_data)
sum(is.na(air_data))
complete.cases(air_data)
complete.cases(air_data$Ozone)
library(VIM)
air_data = airquality[1:31,1:4]
aggr(air_data, las = 1, numbers = TRUE)
P116~117
data1 = air_data[complete.cases(air_data),]
dim(data1)
data2 = air_data[(!is.na(air_data$Ozone))
+ &(!is.na(air_data$Solar.R)),]
dim(data2)
data3 = na.omit(air_data)
dim(data3)
air_data2 = air_data
air_data2$Ozone[is.na(air_data2$Ozone)] =
median(air_data$Ozone[!is.na(air_data$Ozone)])
air_data2$Solar.R[is.na(air_data2$Solar.R)] =
round(mean(air_data$Solar.R[!is.na(air_data$Solar.R)]))
Chapter 7
P119
goods <- list(name="Cookie", price=4.00, outdate=FALSE)
goods
typeof(goods$name)
typeof(goods$price)
typeof(goods$outdate)
goods2 <- list("Cookie", 4.00, FALSE)
goods2
P120
temp <- vector(mode="list")
temp[["name"]] <- "Cookie"
temp
goods$name
goods[["name"]]
goods[[1]]
h1 <- goods["name"]
h2 <- goods[1]
class(h1) #查看h1 的类型
h1
class(h2) #查看h2 的类型
h2
class(goods[["name"]])
class(goods[[1]])
P121~122
goods[1:2]
goods[[1:2]]
names(goods)
goods
goods$producer <- "A Company" #添加标签并初始化
goods
goods[["material"]] <- "flour"
goods[[6]] <- 1
goods
P123~124
goods$material <- NULL
goods
c(list(A=1,c="C"),list(new="NEW"))
unlist(goods)
ngoods <- unlist(goods)
names(ngoods)
names(ngoods) <- NULL
ngoods
mgoods <- unlist(goods)
names(mgoods)
unname(mgoods)
c(goods,recursive=T)
P125~126
temp <- list(1:10,-2:-9)
lapply(temp, mean)
sapply(temp,mean)
sapply(temp,mean,simplify=FALSE,USE.NAMES=FALSE)
a1 <- list(name="Cookie", price=4.0, outdate=FALSE)
a2 <- list(name="Milk", price=2.0, outdate=TRUE)
warehouse <- list(a1, a2)
warehouse
male <- c(124,88,200)
female <- c(108,56,221)
degree <- c("low","middle","high")
myopia <- data.frame(degree,male,female)
myopia
P127
myopia2 <- data.frame(c("low","middle","high"),
+ c(124,88,200),c(108,56,221))
myopia2
weight <- c(50, 70.6, 80, 59.5)
age <- c(20, 30)
wag <- data.frame(weight, age)
wag
str(myopia)
rat <- read.csv("F:/R/data/rat_fibres.csv")
rat
myopia$degree
myopia[["degree"]]
myopia[[1]]
myopia[1,]
myopia[,2]
myopia[3,2]
P129~130
(sub <- myopia[2:3,1:2])
class(sub)
(sub1 <- myopia[2:3,2])
class(sub1)
(sub2 <- myopia[2:3,2,drop=F])
class(sub2)
myopia[1:2]
myopia[1]
myopia[c("male", "female")]
myopia[myopia$male>100,]
myopia[male>100,]
male
male <- c(1,2,3)
myopia[male>100,]
myopia[myopia$male>100,]
P131~134
names <- c("Jack", "Steven")
ages <- c(15, 16)
students <- data.frame(names, ages, stringsAsFactors=F)
students
rbind(students, list("Sariah",15))
cbind(students, gender=c("M","M"))
students
students$gender <- c("M","M")
students
students
students$gender <- NULL
students
students
students2
merge(students,students2)
students
students3
merge(students,students3,by.x="names",by.y="na")
merge(students,students3,by.y="na",by.x="names",all.x=T)
merge(students,students3,by.y="na",by.x="names",all.y=T)
merge(students,students3,by.y="na",by.x="names",all=T)
students4
students
merge(students,students4,by.x="names",by.y="na")
students
tt<-rbind(students,list("Kevin",30))
tt$grade <- c(88,74,90,82)
tt
apply(tt[,2:3,drop=F],2,mean)
P135~136
(s1 <- lapply(students,sort))
(s2 <- sapply(students,sort))
as.data.frame(s1)
as.data.frame(s2)
ssample <- c("BJ","SH","CQ","SH")
(sf <- factor(ssample))
nsample <- c(2,3,3,5)
(nf <- factor(nsample))
str(nf)
unclass(nf)
str(sf)
unclass(sf)
以上是关于R语言实战:机器学习与数据分析源代码5的主要内容,如果未能解决你的问题,请参考以下文章
1024程序员节|代码改变世界,科技创造未来 虚竹哥联合机械工业出版社好书相赠
1024程序员节|代码改变世界,科技创造未来 虚竹哥联合机械工业出版社好书相赠