Spark 实战
Posted yunpiao123456
tags:
篇首语:本文由小常识网(cha138.com)小编为大家整理,主要介绍了Spark 实战相关的知识,希望对你有一定的参考价值。
ALS是交替最小二乘法
ALS是交替最小二乘的简称。在Spark MLlib中特指通过交替最小二乘法求解的协同推荐算法。它通过观察所有用户给产 品打分,来推断每个用户的喜好并向用户推荐适合的产品。用户评分矩阵的每行代表一个用户,每列代表一个物品。我们认为用户评分矩阵是由用户特征矩阵U乘以物品特征矩阵V得到。即A = U*V。因此,如果知道了U、V就不难求得A。 ALS假设打分矩阵A是近似低秩的,也就是一个m*n的打分矩阵A可以用两个m*k和n*k的矩阵乘积近似,其中k远小于m和n。这样降低了整个系统的自由度。 可以通过U、 V矩阵重构打分矩阵A。重构过程中产生的误差可以使用Frobenius范数来量化。实际上就是每个元素与重构之间的误差平方和。因此可得ALS的优化目标如下式所示:
这样,我们就把一个协同推荐问题转化成了一个利用ALS-交替最小二乘法求解的优化问题。只有我们将U、V两个矩阵其中的一个固定,该优化问题才会变成凸且可拆分的问题。交替的意思是,我们随机生成U0,然后固定它求解V0,再固定V0求解U1,这样交替进行。因为每步迭代都会降低重构误差,并且误差有下界,所以ALS一定会收敛。基于问题是非凸的不保证会收敛到全局最优解。但实际中,ALS对初始点不敏感,是不是全局最优解影响并不大。 源码分析概述 MLlib的ALS模型采用了一种高效的矩阵分解计算,主要通过设计数据分区和RDD缓存来减少数据交换。通过如图所示的数据分区结构,在ALS交替迭代过程中,比如通过U求解V,在每个分区中u和v通过合理的分区设计使得在同一个分区中,在计算过程中可以直接在分区中计算,不需要从其他节点或者分区中传输数据,分以下两步: 1)在P1,需要将每一个U发送给不同的Q,将这种关系存储在每一个块中,称作OutBlock。 2)在Q1,我们需要知道每一个V和哪些U有关联及其对应的打分,这部分数据不仅包含原始打分数据,还包含每个用户分区收到的向量排序信息,称作InBlock。 所以从U求解V,我们需要通过用户的OutBlock信息将用户向量发送给产品分区,然后通过产品的InBlock信息构建最小二乘问题并求解。反之也成立。
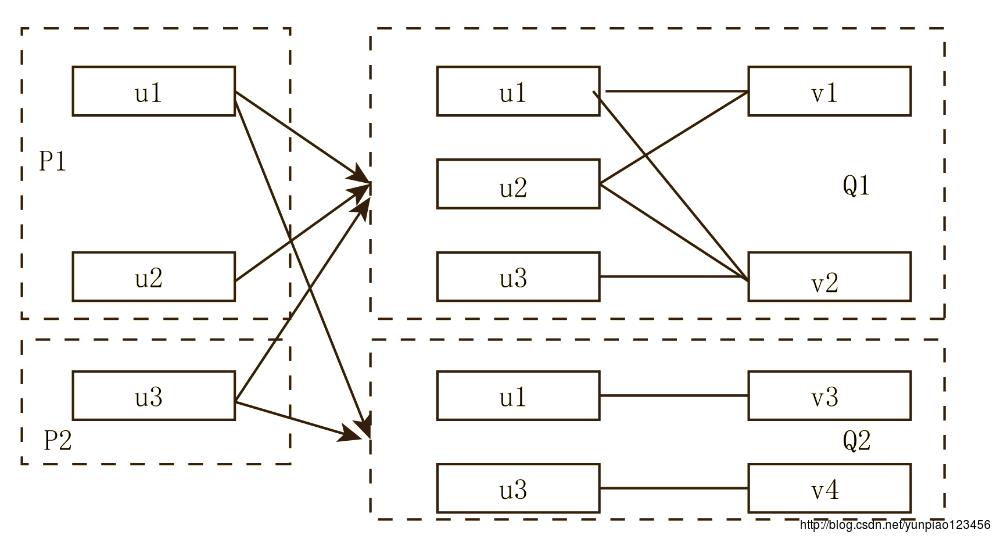
只需要一次扫描就可以建立好信息并且缓存块,从而建立OutBlock、InBlock。这样在以后的迭代计算中可以直接进行计算,大大减少节点数据传输。 MLlib对OutBlock、InBlock的数据结构进行了优化。InBlock数据结构优化如下: v1和v2评分原始数据如下: (v1,u1,a11),(v2,u1,a12),(v1,u2,a21),(v2,u2,a22),(v2,u3,a32) InBlock结构优化1 ([v1,v2,v1,v2,v2],[u1,u1,u2,u2,u3],[a11,a12,a21,a22,a32]) 把多个(v1,u1,a11)三元组数据存储为一个三元组数据,减少了实例数量,并且有效利用连续内存。 InBlock结构优化2 ([v1,v1,v2,v2,v2],[u1,u2,u1,u2,u3],[a11,a21,a12,a22,a32]) 对产品ID排序,解决查找某一个产品ID的复杂度。 InBlock结构优化3 ([v1,v2],[0,2,5],[u1,u2,u1,u2,u3],[a11,a21,a12,a22,a32]) 排序后数据进行了压缩,对于每个产品,只需记录开始和结束位置。 通过优化使得计算数据在内存使用、时间、和空间复杂度上都达到了最佳效果,接下来是源码剖析部分。 源码剖析 该部分代码主要定义了ASL的一些参数设置
/*
* Licensed to the Apache Software Foundation (ASF) under one or more
* contributor license agreements. See the NOTICE file distributed with
* this work for additional information regarding copyright ownership.
* The ASF licenses this file to You under the Apache License, Version 2.0
* (the "License"); you may not use this file except in compliance with
* the License. You may obtain a copy of the License at
*
* http://www.apache.org/licenses/LICENSE-2.0
*
* Unless required by applicable law or agreed to in writing, software
* distributed under the License is distributed on an "AS IS" BASIS,
* WITHOUT WARRANTIES OR CONDITIONS OF ANY KIND, either express or implied.
* See the License for the specific language governing permissions and
* limitations under the License.
*/
package org.apache.spark.mllib.recommendation
import org.apache.spark.annotation.DeveloperApi, Since
import org.apache.spark.api.java.JavaRDD
import org.apache.spark.internal.Logging
import org.apache.spark.ml.recommendation.ALS => NewALS
import org.apache.spark.rdd.RDD
import org.apache.spark.storage.StorageLevel
/**
* A more compact class to represent a rating than Tuple3[Int, Int, Double].
*/
@Since("0.8.0")
case class Rating @Since("0.8.0") (
@Since("0.8.0") user: Int,
@Since("0.8.0") product: Int,
@Since("0.8.0") rating: Double)
/**
* Alternating Least Squares matrix factorization.
*
* ALS attempts to estimate the ratings matrix `R` as the product of two lower-rank matrices,
* `X` and `Y`, i.e. `X * Yt = R`. Typically these approximations are called 'factor' matrices.
* The general approach is iterative. During each iteration, one of the factor matrices is held
* constant, while the other is solved for using least squares. The newly-solved factor matrix is
* then held constant while solving for the other factor matrix.
*
* This is a blocked implementation of the ALS factorization algorithm that groups the two sets
* of factors (referred to as "users" and "products") into blocks and reduces communication by only
* sending one copy of each user vector to each product block on each iteration, and only for the
* product blocks that need that user's feature vector. This is achieved by precomputing some
* information about the ratings matrix to determine the "out-links" of each user (which blocks of
* products it will contribute to) and "in-link" information for each product (which of the feature
* vectors it receives from each user block it will depend on). This allows us to send only an
* array of feature vectors between each user block and product block, and have the product block
* find the users' ratings and update the products based on these messages.
*
* For implicit preference data, the algorithm used is based on
* "Collaborative Filtering for Implicit Feedback Datasets", available at
* <a href="http://dx.doi.org/10.1109/ICDM.2008.22">here</a>, adapted for the blocked approach
* used here.
*
* Essentially instead of finding the low-rank approximations to the rating matrix `R`,
* this finds the approximations for a preference matrix `P` where the elements of `P` are 1 if
* r > 0 and 0 if r <= 0. The ratings then act as 'confidence' values related to strength of
* indicated user
* preferences rather than explicit ratings given to items.
*/
@Since("0.8.0")
class ALS private (
private var numUserBlocks: Int,
private var numProductBlocks: Int,
private var rank: Int,
private var iterations: Int,
private var lambda: Double,
private var implicitPrefs: Boolean,
private var alpha: Double,
private var seed: Long = System.nanoTime()
) extends Serializable with Logging
/**
* Constructs an ALS instance with default parameters: numBlocks: -1, rank: 10, iterations: 10,
* lambda: 0.01, implicitPrefs: false, alpha: 1.0.
*/
@Since("0.8.0")
def this() = this(-1, -1, 10, 10, 0.01, false, 1.0)
/** If true, do alternating nonnegative least squares. */
private var nonnegative = false
/** storage level for user/product in/out links */
private var intermediateRDDStorageLevel: StorageLevel = StorageLevel.MEMORY_AND_DISK
private var finalRDDStorageLevel: StorageLevel = StorageLevel.MEMORY_AND_DISK
/** checkpoint interval */
private var checkpointInterval: Int = 10
/**
* Set the number of blocks for both user blocks and product blocks to parallelize the computation
* into; pass -1 for an auto-configured number of blocks. Default: -1.
*/
@Since("0.8.0")
def setBlocks(numBlocks: Int): this.type =
require(numBlocks == -1 || numBlocks > 0,
s"Number of blocks must be -1 or positive but got $numBlocks")
this.numUserBlocks = numBlocks
this.numProductBlocks = numBlocks
this
/**
* Set the number of user blocks to parallelize the computation.
*/
@Since("1.1.0")
def setUserBlocks(numUserBlocks: Int): this.type =
require(numUserBlocks == -1 || numUserBlocks > 0,
s"Number of blocks must be -1 or positive but got $numUserBlocks")
this.numUserBlocks = numUserBlocks
this
/**
* Set the number of product blocks to parallelize the computation.
*/
@Since("1.1.0")
def setProductBlocks(numProductBlocks: Int): this.type =
require(numProductBlocks == -1 || numProductBlocks > 0,
s"Number of product blocks must be -1 or positive but got $numProductBlocks")
this.numProductBlocks = numProductBlocks
this
/** Set the rank of the feature matrices computed (number of features). Default: 10. */
@Since("0.8.0")
def setRank(rank: Int): this.type =
require(rank > 0,
s"Rank of the feature matrices must be positive but got $rank")
this.rank = rank
this
/** Set the number of iterations to run. Default: 10. */
@Since("0.8.0")
def setIterations(iterations: Int): this.type =
require(iterations >= 0,
s"Number of iterations must be nonnegative but got $iterations")
this.iterations = iterations
this
/** Set the regularization parameter, lambda. Default: 0.01. */
@Since("0.8.0")
def setLambda(lambda: Double): this.type =
require(lambda >= 0.0,
s"Regularization parameter must be nonnegative but got $lambda")
this.lambda = lambda
this
/** Sets whether to use implicit preference. Default: false. */
@Since("0.8.1")
def setImplicitPrefs(implicitPrefs: Boolean): this.type =
this.implicitPrefs = implicitPrefs
this
/**
* Sets the constant used in computing confidence in implicit ALS. Default: 1.0.
*/
@Since("0.8.1")
def setAlpha(alpha: Double): this.type =
this.alpha = alpha
this
/** Sets a random seed to have deterministic results. */
@Since("1.0.0")
def setSeed(seed: Long): this.type =
this.seed = seed
this
/**
* Set whether the least-squares problems solved at each iteration should have
* nonnegativity constraints.
*/
@Since("1.1.0")
def setNonnegative(b: Boolean): this.type =
this.nonnegative = b
this
/**
* :: DeveloperApi ::
* Sets storage level for intermediate RDDs (user/product in/out links). The default value is
* `MEMORY_AND_DISK`. Users can change it to a serialized storage, e.g., `MEMORY_AND_DISK_SER` and
* set `spark.rdd.compress` to `true` to reduce the space requirement, at the cost of speed.
*/
@DeveloperApi
@Since("1.1.0")
def setIntermediateRDDStorageLevel(storageLevel: StorageLevel): this.type =
require(storageLevel != StorageLevel.NONE,
"ALS is not designed to run without persisting intermediate RDDs.")
this.intermediateRDDStorageLevel = storageLevel
this
/**
* :: DeveloperApi ::
* Sets storage level for final RDDs (user/product used in MatrixFactorizationModel). The default
* value is `MEMORY_AND_DISK`. Users can change it to a serialized storage, e.g.
* `MEMORY_AND_DISK_SER` and set `spark.rdd.compress` to `true` to reduce the space requirement,
* at the cost of speed.
*/
@DeveloperApi
@Since("1.3.0")
def setFinalRDDStorageLevel(storageLevel: StorageLevel): this.type =
this.finalRDDStorageLevel = storageLevel
this
/**
* :: DeveloperApi ::
* Set period (in iterations) between checkpoints (default = 10). Checkpointing helps with
* recovery (when nodes fail) and StackOverflow exceptions caused by long lineage. It also helps
* with eliminating temporary shuffle files on disk, which can be important when there are many
* ALS iterations. If the checkpoint directory is not set in [[org.apache.spark.SparkContext]],
* this setting is ignored.
*/
@DeveloperApi
@Since("1.4.0")
def setCheckpointInterval(checkpointInterval: Int): this.type =
this.checkpointInterval = checkpointInterval
this
/**
* Run ALS with the configured parameters on an input RDD of [[Rating]] objects.
* Returns a MatrixFactorizationModel with feature vectors for each user and product.
*/
@Since("0.8.0")
def run(ratings: RDD[Rating]): MatrixFactorizationModel =
val sc = ratings.context
val numUserBlocks = if (this.numUserBlocks == -1)
math.max(sc.defaultParallelism, ratings.partitions.length / 2)
else
this.numUserBlocks
val numProductBlocks = if (this.numProductBlocks == -1)
math.max(sc.defaultParallelism, ratings.partitions.length / 2)
else
this.numProductBlocks
/** 采用交替最小二乘求解用户和物品的特征矩阵 */
val (floatUserFactors, floatProdFactors) = NewALS.train[Int](
ratings = ratings.map(r => NewALS.Rating(r.user, r.product, r.rating.toFloat)),
rank = rank,
numUserBlocks = numUserBlocks,
numItemBlocks = numProductBlocks,
maxIter = iterations,
regParam = lambda,
implicitPrefs = implicitPrefs,
alpha = alpha,
nonnegative = nonnegative,
intermediateRDDStorageLevel = intermediateRDDStorageLevel,
finalRDDStorageLevel = StorageLevel.NONE,
checkpointInterval = checkpointInterval,
seed = seed)
val userFactors = floatUserFactors
.mapValues(_.map(_.toDouble))
.setName("users")
.persist(finalRDDStorageLevel)
val prodFactors = floatProdFactors
.mapValues(_.map(_.toDouble))
.setName("products")
.persist(finalRDDStorageLevel)
if (finalRDDStorageLevel != StorageLevel.NONE)
userFactors.count()
prodFactors.count()
new MatrixFactorizationModel(rank, userFactors, prodFactors)
/**
* Java-friendly version of `ALS.run`.
*/
@Since("1.3.0")
def run(ratings: JavaRDD[Rating]): MatrixFactorizationModel = run(ratings.rdd)
/**
* Top-level methods for calling Alternating Least Squares (ALS) matrix factorization.
*/
@Since("0.8.0")
object ALS
/**
* Train a matrix factorization model given an RDD of ratings by users for a subset of products.
* The ratings matrix is approximated as the product of two lower-rank matrices of a given rank
* (number of features). To solve for these features, ALS is run iteratively with a configurable
* level of parallelism.
*
* @param ratings RDD of [[Rating]] objects with userID, productID, and rating
* @param rank number of features to use
* @param iterations number of iterations of ALS
* @param lambda regularization parameter
* @param blocks level of parallelism to split computation into
* @param seed random seed for initial matrix factorization model
*/
@Since("0.9.1")
def train(
ratings: RDD[Rating],
rank: Int,
iterations: Int,
lambda: Double,
blocks: Int,
seed: Long
): MatrixFactorizationModel =
new ALS(blocks, blocks, rank, iterations, lambda, false, 1.0, seed).run(ratings)
/**
* Train a matrix factorization model given an RDD of ratings by users for a subset of products.
* The ratings matrix is approximated as the product of two lower-rank matrices of a given rank
* (number of features). To solve for these features, ALS is run iteratively with a configurable
* level of parallelism.
*
* @param ratings RDD of [[Rating]] objects with userID, productID, and rating
* @param rank number of features to use
* @param iterations number of iterations of ALS
* @param lambda regularization parameter
* @param blocks level of parallelism to split computation into
*/
@Since("0.8.0")
def train(
ratings: RDD[Rating],
rank: Int,
iterations: Int,
lambda: Double,
blocks: Int
): MatrixFactorizationModel =
new ALS(blocks, blocks, rank, iterations, lambda, false, 1.0).run(ratings)
/**
* Train a matrix factorization model given an RDD of ratings by users for a subset of products.
* The ratings matrix is approximated as the product of two lower-rank matrices of a given rank
* (number of features). To solve for these features, ALS is run iteratively with a level of
* parallelism automatically based on the number of partitions in `ratings`.
*
* @param ratings RDD of [[Rating]] objects with userID, productID, and rating
* @param rank number of features to use
* @param iterations number of iterations of ALS
* @param lambda regularization parameter
*/
@Since("0.8.0")
def train(ratings: RDD[Rating], rank: Int, iterations: Int, lambda: Double)
: MatrixFactorizationModel =
train(ratings, rank, iterations, lambda, -1)
/**
* Train a matrix factorization model given an RDD of ratings by users for a subset of products.
* The ratings matrix is approximated as the product of two lower-rank matrices of a given rank
* (number of features). To solve for these features, ALS is run iteratively with a level of
* parallelism automatically based on the number of partitions in `ratings`.
*
* @param ratings RDD of [[Rating]] objects with userID, productID, and rating
* @param rank number of features to use
* @param iterations number of iterations of ALS
*/
@Since("0.8.0")
def train(ratings: RDD[Rating], rank: Int, iterations: Int)
: MatrixFactorizationModel =
train(ratings, rank, iterations, 0.01, -1)
/**
* Train a matrix factorization model given an RDD of 'implicit preferences' given by users
* to some products, in the form of (userID, productID, preference) pairs. We approximate the
* ratings matrix as the product of two lower-rank matrices of a given rank (number of features).
* To solve for these features, we run a given number of iterations of ALS. This is done using
* a level of parallelism given by `blocks`.
*
* @param ratings RDD of (userID, productID, rating) pairs
* @param rank number of features to use
* @param iterations number of iterations of ALS
* @param lambda regularization parameter
* @param blocks level of parallelism to split computation into
* @param alpha confidence parameter
* @param seed random seed for initial matrix factorization model
*/
@Since("0.8.1")
def trainImplicit(
ratings: RDD[Rating],
rank: Int,
iterations: Int,
lambda: Double,
blocks: Int,
alpha: Double,
seed: Long
): MatrixFactorizationModel =
new ALS(blocks, blocks, rank, iterations, lambda, true, alpha, seed).run(ratings)
/**
* Train a matrix factorization model given an RDD of 'implicit preferences' of users for a
* subset of products. The ratings matrix is approximated as the product of two lower-rank
* matrices of a given rank (number of features). To solve for these features, ALS is run
* iteratively with a configurable level of parallelism.
*
* @param ratings RDD of [[Rating]] objects with userID, productID, and rating
* @param rank number of features to use
* @param iterations number of iterations of ALS
* @param lambda regularization parameter
* @param blocks level of parallelism to split computation into
* @param alpha confidence parameter
*/
@Since("0.8.1")
def trainImplicit(
ratings: RDD[Rating],
rank: Int,
iterations: Int,
lambda: Double,
blocks: Int,
alpha: Double
): MatrixFactorizationModel =
new ALS(blocks, blocks, rank, iterations, lambda, true, alpha).run(ratings)
/**
* Train a matrix factorization model given an RDD of 'implicit preferences' of users for a
* subset of products. The ratings matrix is approximated as the product of two lower-rank
* matrices of a given rank (number of features). To solve for these features, ALS is run
* iteratively with a level of parallelism determined automatically based on the number of
* partitions in `ratings`.
*
* @param ratings RDD of [[Rating]] objects with userID, productID, and rating
* @param rank number of features to use
* @param iterations number of iterations of ALS
* @param lambda regularization parameter
* @param alpha confidence parameter
*/
@Since("0.8.1")
def trainImplicit(ratings: RDD[Rating], rank: Int, iterations: Int, lambda: Double, alpha: Double)
: MatrixFactorizationModel =
trainImplicit(ratings, rank, iterations, lambda, -1, alpha)
/**
* Train a matrix factorization model given an RDD of 'implicit preferences' of users for a
* subset of products. The ratings matrix is approximated as the product of two lower-rank
* matrices of a given rank (number of features). To solve for these features, ALS is run
* iteratively with a level of parallelism determined automatically based on the number of
* partitions in `ratings`.
*
* @param ratings RDD of [[Rating]] objects with userID, productID, and rating
* @param rank number of features to use
* @param iterations number of iterations of ALS
*/
@Since("0.8.1")
def trainImplicit(ratings: RDD[Rating], rank: Int, iterations: Int)
: MatrixFactorizationModel =
trainImplicit(ratings, rank, iterations, 0.01, -1, 1.0)
以上是关于Spark 实战的主要内容,如果未能解决你的问题,请参考以下文章